人机验证
内容 (Contents)
-
-
--
Trust, transparency & responsibility
-
--
Fair & ethical design standards
-
--
-
--
1.简介 (1. Intro)
Human + Machine is written by Paul Daugherty (Accenture Chief Technology Officer) and James Wilson (Accenture Managing Director of IT and Business Research).
Human + Machine由Paul Daugherty(埃森哲首席技术官)和James Wilson(埃森哲IT和业务研究常务董事)撰写。
Dispelling the common narrative of the binary approach of human vs machine “fighting for the other’s jobs”, Daugherty and Wilson argue in favour of a future symbiosis between human and machine, in what they call “the third wave of business transformation”. Wave 1 involved standardised processes — think Henry Ford. Wave 2 saw automation — IT from the 1970s-90s. Wave 3 will involve “adaptive processes… driven by real-time data rather than by a prior sequence of steps”.
Daugherty和Wilson驳斥了人类与机器“为他人的工作而战”的二元方法的共同叙述,主张人类与机器之间未来的共生,即所谓的“ 第三波业务转型 ”。 第一波涉及标准化流程-想想亨利·福特(Henry Ford)。 Wave 2看到了自动化-1970年代至90年代的IT。 第三波将涉及“自适应过程……由实时数据驱动,而不是由先前的步骤序列驱动”。
This “third wave” will allow for “machines … doing what they do best: performing repetitive tasks, analysing huge data sets, and handling routine cases. And humans … doing what they do best: resolving ambiguous information, exercising judgment in difficult cases, and dealing with dissatisfied customers.” They go further in discussing the “missing middle” — human and machine hybrid activities — where “humans complement machines”, and “AI gives humans superpowers”, as well as making a business case for AI: “AI isn’t your typical capital investment; its value actually increases over time and it, in turn, improves the value of people as well.” And unexpectedly, “the problem right now isn’t so much that robots are replacing jobs; it’s that workers aren’t prepared with the right skills necessary for jobs that are evolving fast due to new technologies, such as AI.” Future issues will actually surround the constant re-skilling and learning of the workforce.
这“第三波”将使“机器……尽力而为:执行重复性任务,分析大量数据集并处理例行案件。 而人类……做他们最擅长的事情:解决模棱两可的信息,在困难的情况下做出判断,并与不满意的客户打交道。” 他们进一步讨论了“ 缺失的中间点 ”,即人与机器的混合活动,其中“人类补充了机器”,“人工智能赋予了人类超能力”,并提出了人工智能的商业案例:“人工智能不是您的典型资本投资; 它的价值实际上随着时间的推移而增加,反过来也提高了人们的价值。” 出乎意料的是,“目前的问题不仅仅在于机器人正在替代工作;而是在于解决问题。” 这是因为,由于诸如AI之类的新技术,工人没有为快速发展的工作所必需的正确技能做好准备。” 未来的问题实际上将围绕员工不断的重新技能和学习。
2. AI —概述 (2. AI — An Overview)
First envisioned in the 1950s, Artificial Intelligence (AI) and Machine Learning (ML) allow computers to see patterns in data and produce outputs without every step of the process being hard-coded. To distinguish the two, AI is the broader concept of machines carrying out ‘intelligent’ tasks, and ML is an application of AI where machines can be provided with data (e.g. an individual’s health data), learn for themselves, and produce an output (e.g. a recommended course of treatment for an individual).
人工智能(AI)和机器学习(ML)最早在1950年代被设想出来,它使计算机可以看到数据模式并产生输出,而无需对过程的每个步骤进行硬编码。 为了区别两者,人工智能是机器执行“智能”任务的广义概念,而机器学习是人工智能的应用,在机器上可以向机器提供数据(例如个人的健康数据),自己学习并产生输出(例如针对个人的推荐治疗方案)。
After some funding dry-ups, AI has been gaining popularity since around the 2000s and is becoming ever more visible in businesses and everyday life today: you wake up to an alarm set by Alexa, who tells you your schedule for the day. You open your computer, likely made by smart robots in a factory. You log in, making use of its facial recognition system. You procrastinate from work by scrolling a curated Facebook feed designed to keep your attention, or on Netflix where you’re recommended TV shows and movies to cater to your viewing-tastes. It’s also likely your work software implements many kinds of AI and ML.
经过一番资金枯竭之后,自2000年代以来,人工智能就开始流行,并且在当今的企业和日常生活中变得越来越明显:您会醒来Alexa发出的警报,告诉您当天的行程。 您打开计算机,这很可能是由工厂中的智能机器人制造的。 您使用其面部识别系统登录。 您可以滚动浏览旨在吸引您注意力的精选Facebook feed,或在Netflix上推荐您观看电视节目和电影来满足自己的观看口味的工作,从而拖延工作。 您的工作软件也可能实现了多种AI和ML。
These algorithms work by having train and test data. For instance, a CV-checking model could be trained on past hiring data, accepting through to the next stage people who demonstrate qualities described in the job description, and rejecting those who do not. The model then gets fed new test data (new CVs it hasn’t seen before) so someone can check whether the predicted outcomes are desirable. Once the model has been tweaked, it is rolled out for use on actual applicants’ CVs. The issue then is making sure the training data is not biased — take the 2014 case study where Amazon’s trial recruitment algorithm was trained on data where a majority of men were hired, resulting in female applicants being penalised — this algorithm was soon scrapped.
这些算法通过训练和测试数据来工作 。 例如,可以使用过去的招聘数据来训练CV检查模型,接受进入下一个阶段的人员,这些人员要表现出工作说明中描述的素质,而拒绝那些没有表现出来的素质。 然后,该模型将获得新的测试数据(之前从未见过的新CV),因此有人可以检查预测结果是否令人满意。 对模型进行调整后,将其推出以供实际申请人的简历使用。 然后,问题是确保培训数据没有偏见-以2014年的案例研究为例,其中亚马逊的试行招募算法是根据雇用大多数男性的数据进行培训的,从而导致女性申请人受到惩罚-该算法很快就被取消了。
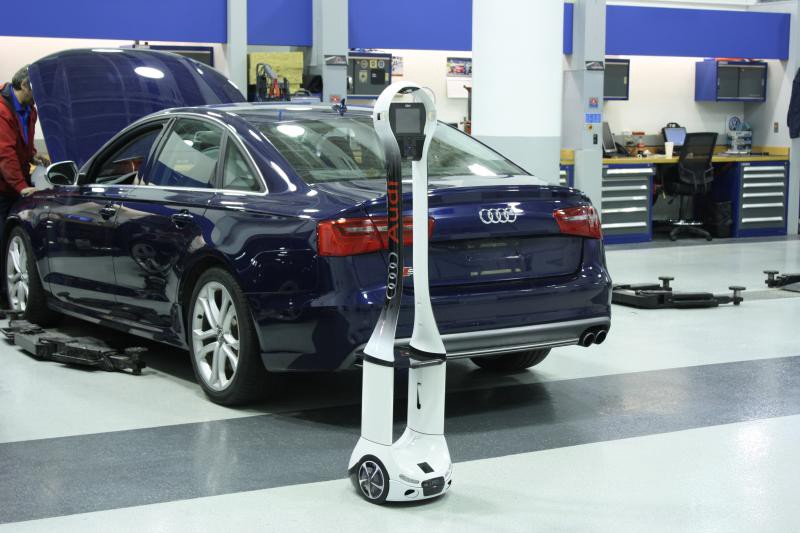
There are different branches of ML too:
ML也有不同的分支:
Supervised learning