用python画熊猫
Python短裤 (Python Shorts)
Pandas is one of the best data manipulation libraries in recent times. It lets you slice and dice, groupby, join and do any arbitrary data transformation. You can take a look at this post, which talks about handling most of the data manipulation cases using a straightforward, simple, and matter of fact way using Pandas.
熊猫是最近最好的数据处理库之一。 它使您可以切片,切块,分组,加入并进行任意数据转换。 您可以看一下这篇文章 ,该文章讨论了使用Pandas通过一种简单,简单且实际的方式处理大多数数据处理案例。
But even with how awesome pandas generally is, there sometimes are moments when you would like to have just a bit more. Say you come from a SQL background in which the same operation was too easy. Or you wanted to have more readable code. Or you just wanted to run an ad-hoc SQL query on your data frame. Or, maybe you come from R and want a replacement for sqldf.
但是,即使一般的熊猫都很棒,有时您还是会想要多一点。 假设您来自SQL背景,在该背景下,同一操作太容易了。 或者您想拥有更具可读性的代码。 或者,您只想在数据框上运行临时SQL查询。 或者,也许您来自R并想要替代sqldf.
For example, one of the operations that Pandas doesn’t have an alternative for is non-equi joins, which are quite trivial in SQL.
例如,Pandas没有替代品的操作之一是非等额联接,这在SQL中非常简单。
In this series of posts named Python Shorts, I will explain some simple but very useful constructs provided by Python, some essential tips, and some use cases I come up with regularly in my Data Science work.
在这一系列名为Python Shorts的文章中,我将解释一些由Python提供的简单但非常有用的结构,一些基本技巧以及一些在数据科学工作中定期提出的用例。
This post is essentially about using SQL with pandas Dataframes.
这篇文章实质上是关于将SQL与pandas Dataframes一起使用。
但是,什么是非等额联接,为什么我需要它们? (But, what are non-equi joins, and why would I need them?)
Let’s say you have to join two data frames. One shows us the periods where we offer some promotions on some items. And the second one is our transaction Dataframe. I want to know the sales that were driven by promotions, i.e., the sales that happen for an item in the promotion period.
假设您必须连接两个数据框。 一个向我们展示了我们在某些项目上提供促销的时期。 第二个是我们的交易数据框。 我想知道由促销推动的销售,即促销期间某项目发生的销售。
We can do this by doing a join on the item
column as well as a join condition (TransactionDt≥StartDt
and TransactionDt≤EndDt
). Since now our join conditions have a greater than and less than signs as well, such joins are called non-equi joins. Do think about how you will do such a thing in Pandas before moving on.
我们可以通过在item
列上进行item
以及联接条件( TransactionDt≥StartDt
和TransactionDt≤EndDt
)来实现。 从现在开始,我们的联接条件也具有大于和小于的符号,这种联接称为非等联接。 在继续之前,请考虑一下如何在熊猫中做这样的事情。
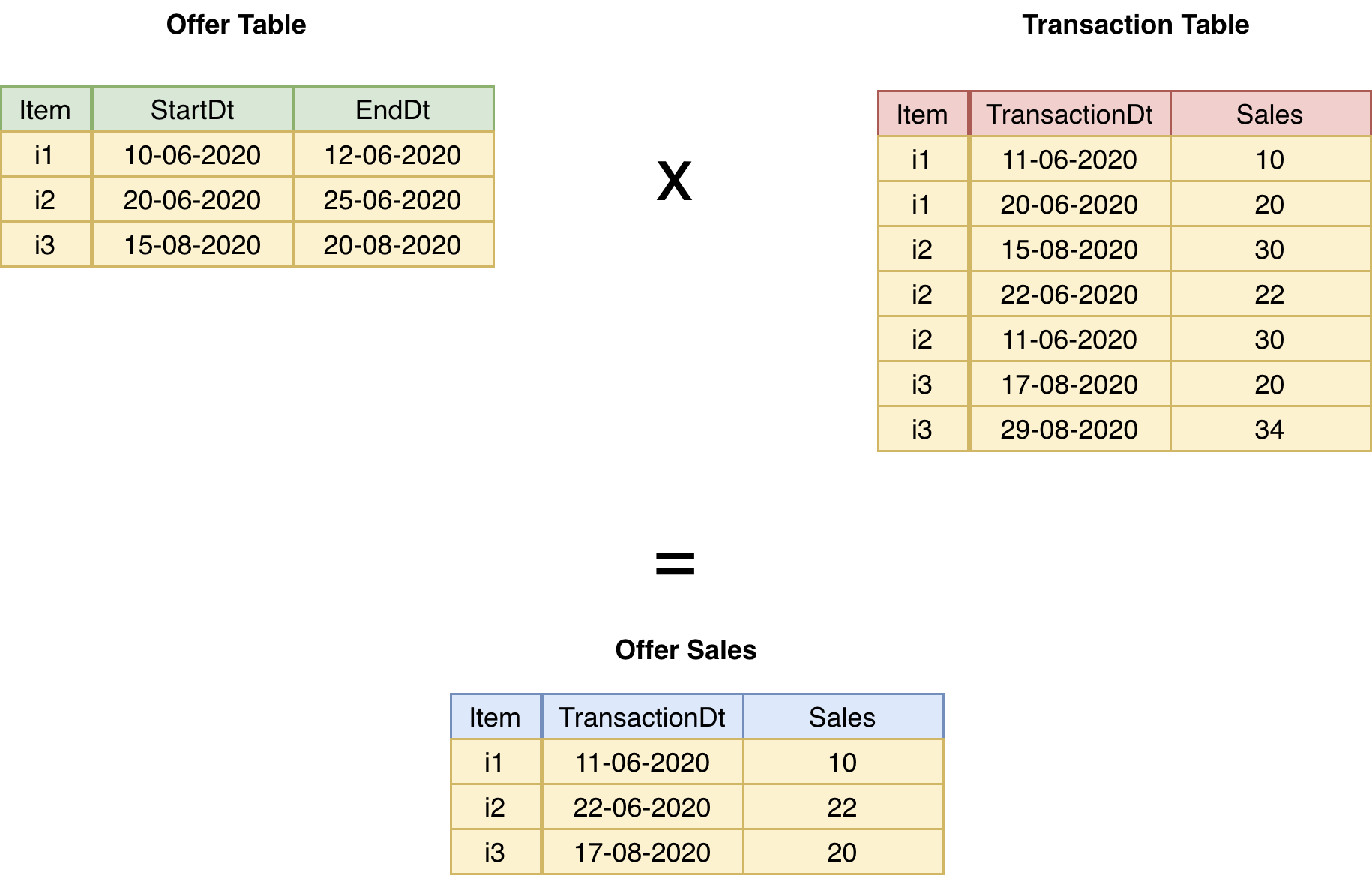
熊猫解决方案 (The Pandas Solution)
So how will you do it in Pandas? Yes, a Pandas based solution exists, though I don’t find it readable enough.
那么,您将如何在Pandas中做到这一点? 是的,存在基于Pandas的解决方案,尽管我认为它不够可读。
Let’s start by generating some random data to work with.
让我们从生成一些随机数据开始。
import pandas as pd
import random
import datetime
def random_dt_bw(start_date,end_date):
days_between = (end_date - start_date).days
random_num_days = random.randrange(days_between)
random_dt = start_date + datetime.timedelta(days=random_num_days)
return random_dt
def generate_data(n=1000):
items = [f"i_{x}" for x in range(n)]
start_dates = [random_dt_bw(datetime.date(2020,1,1),datetime.date(2020,9,1)) for x in range(n)]
end_dates = [x + datetime.timedelta(days=random.randint(1,10)) for x in start_dates]
offerDf = pd.DataFrame({"Item":items,
"StartDt":start_dates,
"EndDt":end_dates})
transaction_items = [f"i_{random.randint(0,n)}" for x in range(5*n)]
transaction_dt = [random_dt_bw(datetime.date(2020,1,1),datetime.date(2020,9,1)) for x in range(5*n)]
sales_amt = [random.randint(0,1000) for x in range(5*n)]
transactionDf = pd.DataFrame({"Item":transaction_items,"TransactionDt":transaction_dt,"Sales":sales_amt})
return offerDf,transactionDf
offerDf,transactionDf = generate_data(n=100000)
You don’t need to worry about the random data generation code above. Just know how our random data looks like:
您无需担心上面的随机数据生成代码。 只要知道我们的随机数据是什么样子:
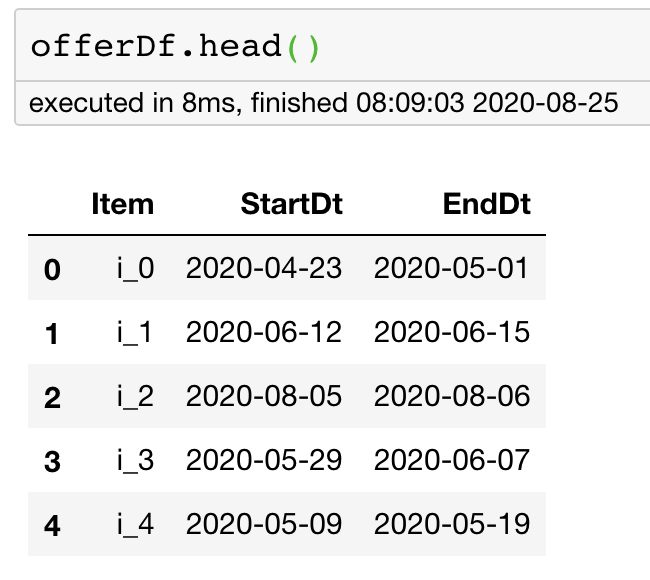

Once we have the data, we can do the non-equi join by merging the data on the column item
and then filtering by the required condition.
有了数据后,我们可以通过合并列item
上的数据,然后按所需条件进行过滤来进行非等价联接。
merged_df = pd.merge(offerDf,transactionDf,on='Item')pandas_solution = merged_df[(merged_df['TransactionDt']>=merged_df['StartDt']) &
(merged_df['TransactionDt']<=merged_df['EndDt'])]
The result is below just as we wanted:
结果如下所示:

PandaSQL解决方案 (The PandaSQL solution)
The Pandas solution is alright, and it does what we want, but we could also have used PandaSQL to get the same thing done in a much more readable way.
Pandas解决方案还可以,它可以满足我们的要求,但是我们也可以使用PandaSQL以更易读的方式完成同样的事情。
What is PandaSQL?
什么是PandaSQL ?
PandaSQL provides us with a way to write SQL on Pandas Dataframes. So if you have got some SQL queries already written, it might make more sense to use pandaSQL rather than converting them to pandas syntax. To get started with PandaSQL we install it simply with:
PandaSQL为我们提供了一种在Pandas Dataframe上编写SQL的方法。 因此,如果您已经编写了一些SQL查询,则使用pandaSQL而不是将其转换为pandas语法可能更有意义。 要开始使用PandaSQL,我们只需使用以下命令进行安装:
pip install -U pandasql
Once we have pandaSQL installed, we can use it by creating a pysqldf
function that takes a query as an input and runs the query to return a Pandas DF. Don’t worry about the syntax; it remains more or less constant.
一旦安装了pandaSQL,就可以通过创建pysqldf
函数来使用它,该函数将查询作为输入并运行查询以返回Pandas DF。 不用担心语法。 它或多或少保持不变。
from pandasql import sqldf
pysqldf = lambda q: sqldf(q, globals())
We can now run any SQL query on our Pandas data frames using this function. And, below is the non-equi join, we want to do in the much more readable SQL format.
现在,我们可以使用此函数在Pandas数据帧上运行任何SQL查询。 而且,下面是非等号联接,我们希望以更具可读性SQL格式进行操作。
q = """
SELECT A.*,B.TransactionDt,B.Sales
FROM
offerDf A
INNER JOIN
transactionDf B
ON
A.Item = B.Item AND
A.StartDt <= B.TransactionDt AND
A.EndDt >= B.TransactionDt;
"""
pandaSQL_solution = pysqldf(q)
The result is a pandas Dataframe as we would expect. The index is already reset for us, unlike before.
结果就是我们期望的熊猫数据框。 与以前不同,该索引已为我们重置。

注意事项: (Caveats:)
While the PandaSQL function lets us run SQL queries on our Pandas data frames and is an excellent tool to be aware of in certain situations, it is not as performant as pure pandas syntax.
虽然PandaSQL函数使我们可以在Pandas数据帧上运行SQL查询,并且是在某些情况下要注意的出色工具,但它的性能不如纯Pandas语法高。

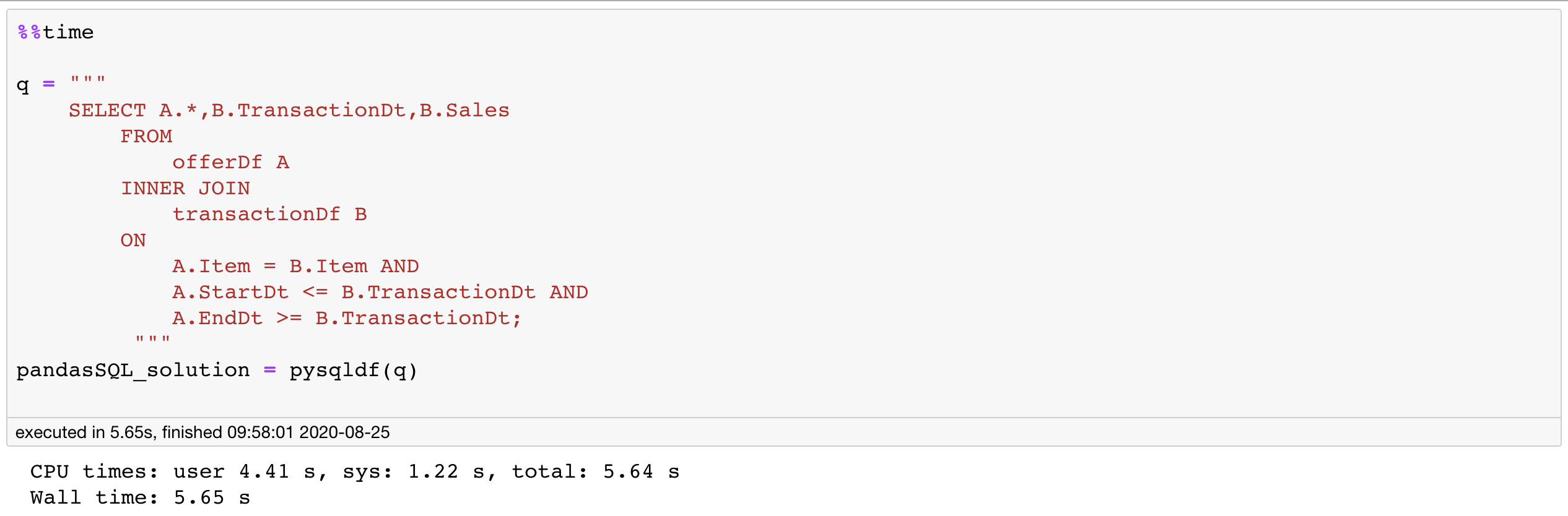
When we time Pandas against the more readable PandaSQL, we find that the PandaSQL takes around 10x the time of native Pandas.
当我们将Pandas与更具可读性的PandaSQL进行计时时,我们发现PandaSQL花费的时间是本地Pandas的10倍左右。
结论 (Conclusion)
In this post of the Python Shorts series, we learned about pandaSQL, which lets us use SQL queries on our Dataframes. We also looked at how to do non-equi joins using both native pandas as well as pandaSQL.
在Python Shorts系列的这篇文章中,我们了解了pandaSQL,它使我们可以在数据框上使用SQL查询。 我们还研究了如何使用本地pandas和pandaSQL进行非等额联接。
While the PandaSQL library is not as performant as native pandas, it is a great addition to our data analytics toolbox when we want to do ad-hoc analysis and to people who feel much more comfortable with using SQL queries.
虽然PandaSQL库的性能不如本地熊猫,但它是我们想要进行即席分析的数据分析工具箱的一个很好的补充,对于那些更愿意使用SQL查询的人来说,它是一个很好的补充。
For a closer look at the code for this post, please visit my GitHub repository, where you can find the code for this post as well as all my posts.
要进一步了解该文章的代码,请访问我的GitHub存储库,在这里您可以找到该文章的代码以及我的所有文章。
继续学习 (Continue Learning)
If you want to learn more about Python 3, I would like to call out an excellent course on Learn Intermediate level Python from the University of Michigan. Do check it out.
如果您想了解有关Python 3的更多信息,我想在密歇根大学(University of Michigan)开设一门优秀的学习中级Python的课程。 请检查一下。
I am going to be writing more beginner-friendly posts in the future too. Follow me up at Medium or Subscribe to my blog to be informed about them. As always, I welcome feedback and constructive criticism and can be reached on Twitter @mlwhiz.
我将来也会写更多对初学者友好的文章。 在Medium上关注我,或订阅我的博客以了解有关它们的信息。 与往常一样,我欢迎您提供反馈和建设性的批评,可以在Twitter @mlwhiz上与我们联系 。
Also, a small disclaimer — There might be some affiliate links in this post to relevant resources, as sharing knowledge is never a bad idea.
另外,这是一个小的免责声明-由于共享知识从来都不是一个坏主意,因此本文中可能会有一些与相关资源相关的会员链接。
翻译自: https://towardsdatascience.com/when-pandas-is-not-enough-use-pandasql-d762b9b84b38
用python画熊猫