dsp出价
In this article I will take you through how I developed an emotion recognition system using speech as an input and then boosted performance using self-supervised representations trained with Contrastive Predictive Coding (CPC). Results have improved from a baseline of 71% to 80% accuracy when using CPC. This is a significant relative reduction in error of 30%.
在本文中,我将带您逐步了解如何开发一种使用语音作为输入的情感识别系统,然后如何使用经过对比预测编码(CPC)训练的自我监督表示来提高性能的系统。 使用CPC时,结果从71%的基线精度提高到80%。 相对误差显着降低了30%。
View the full code here.
在此处查看完整代码。
In addition, I benchmarked various architectures of the model trained using these representations which include simple multilayer perceptrons (MLPs), Recurrent Neural Networks (RNNs) and WaveNet style models that use dilated convolutions.
此外,我对使用这些表示训练的模型的各种体系结构进行了基准测试,包括简单的多层感知器(MLP),递归神经网络(RNN)和使用扩张卷积的WaveNet样式模型。
I found that a bi-directional RNN model using pre-trained CPC representations as input features was the highest performing setup that leads to 79.6% frame-wise accuracy when classifying eight emotions in the RAVDESS dataset. To the best of my knowledge, this is a very competitive system compared to others trained on this data.
我发现,使用预训练的CPC表示作为输入特征的双向RNN模型是性能最高的设置,在对RAVDESS数据集中的八种情绪进行分类时,可达到79.6%的逐帧准确性。 据我所知,与接受此数据培训的其他系统相比,这是一个非常有竞争力的系统。
介绍 (Introduction)
Emotion recognition from speech involves predicting someone’s emotion from a set of classes such as happy, sad, angry, etc. There are many potential applications in businesses such as call centres, health care and human resources [1]. For example, in a call centre, it would allow an automated way of discovering sentiment of potential customers to guide a sales representative towards a better sales approach.
语音中的情感识别涉及从诸如快乐,悲伤,愤怒等一组类别中预测某人的情感。在呼叫中心,医疗保健和人力资源等业务中有许多潜在的应用[ 1 ]。 例如,在呼叫中心,这将允许发现潜在客户情绪的自动化方法,以指导销售代表寻求更好的销售方法。
Predicting an emotion from audio is challenging, since emotions are perceived differently from person to person and can often be difficult to interpret. In addition, many emotional cues come from areas unrelated to speech such as facial expressions, the person’s particular mentality and the context of the interaction. As humans we naturally take all of these signals into account as well as our past communication experiences before making a final judgment. Some authors improve performance using multimodal approaches where audio is combined with text [3,4] or video [5]. Ideally, a world model that understands the links between these areas and social interactions (see World Scopes in [2]) would be trained for this task. However, this is an on going area of research and it is currently unclear how to learn from social interactions, rather than just learning trends from the data itself. In this work, I boost the performance by using self-supervised representations training with a Contrastive Predictive Coding (CPC [8]) framework rather than multimodal training.
从音频中预测情感是具有挑战性的,因为情感因人而异,通常难以解释。 此外,许多情绪暗示来自与言语无关的领域,例如面部表情,人的特殊心态和互动背景。 作为人类,我们在做出最终判断之前自然会考虑所有这些信号以及我们过去的交流经验。 一些作者使用多模式方法提高了性能,其中音频与文本[3,4]或视频[5]相结合。 理想情况下,将训练一个了解这些区域与社会互动之间的联系的世界模型(请参阅[2]中的“世界范围” )。 但是,这是一个进行中的研究领域,目前尚不清楚如何从社交互动中学习,而不仅仅是从数据本身中学习趋势。 在这项工作中,我通过使用自预测表示训练和对比预测编码(CPC [8])框架而不是多模式训练来提高性能。
In the field of representational learning for speech, phone and speaker identification are widely used to evaluate features generated from self-supervised learning techniques since they evaluate local and global structure in the audio respectively. In this article, I demonstrate that emotion recognition can also be used as a downstream task for gauging the quality of representations. Furthermore, classifying emotions supplements phone and speaker identification when benchmarking how good representations are, since emotions only loosely depend on the words being said or how a persons voice sounds.
在语音的代表性学习领域中,电话和说话者识别被广泛用于评估自监督学习技术产生的特征,因为它们分别评估音频中的局部和全局结构。 在本文中,我演示了情感识别也可以用作衡量表示质量的下游任务。 此外,对情感进行分类可以在基准测试良好的表示效果时补充电话和说话者的识别能力,因为情感仅大致取决于说出的单词或人的发音方式。
相关工作 (Related work)
情绪识别 (Emotion recognition)
The majority of emotion recognition systems [3,4,6] have been trained using Mel-Frequency Cepstral Coefficients (MFCCs) which are popular audio features based on a frequency spectrogram. Fbanks, also known as Mel spectrograms, are similar to MFCCs and are widely used. Both capture the frequency content that humans are sensitive to. There has been little work showing the performance gains when using machine learned features through self-supervised learning on the emotion recognition task. It is worth noting that MFCCs and Fbanks can still be used as an input to the self-supervised task instead of raw audio and can often be a good starting point when extracting richer representations. I will talk more about that later.
大多数情绪识别系统[3,4,6]已经使用Mel-频率倒谱系数 (MFCC)进行了训练,它们是基于频谱图的流行音频功能。 Fbank,也称为梅尔(Mel)频谱图,类似于MFCC,并被广泛使用。 两者都捕获了人类敏感的频率内容。 很少有工作显示通过情绪监督任务的自我监督学习使用机器学习功能时的性能提升。 值得注意的是,MFCC和Fbank仍可以用作自我监督任务的输入,而不是原始音频,并且在提取更丰富的表示形式时通常可以作为一个很好的起点。 稍后我将详细讨论。
自我监督学习 (Self-supervised learning)
There are a variety of self-supervised techniques for speech. Self-supervised learning is ‘unsupervised’ in the sense that it takes advantage of the inherent structure of the data to generate labels. The motivation is the ability to use vast quantities of unlabelled audio data on the internet to generate general representations in a similar way that language models learn from unlabelled text data. Ideally, this leads to needing less human labelled data to get the same performance on a downstream task compared to a fully supervised approach. Less human labelled data means, for example, companies can avoid using expensive transcribers to get accurate audio transcripts for automatic speech recognition (ASR).
有各种各样的语音自我监督技术。 自我监督学习是“无监督的”,即它利用数据的固有结构来生成标签。 其动机是能够使用互联网上的大量未标记音频数据以类似于语言模型从未标记文本数据中学习的方式生成一般表示的能力。 理想情况下,与完全监督的方法相比,这需要较少的人工标记数据才能在下游任务上获得相同的性能。 较少的人工标记数据意味着,例如,公司可以避免使用昂贵的转录器来获取准确的音频转录本,以进行自动语音识别(ASR)。
Relying purely on supervised learning has the perils of task specific solutions where the models may struggle to generalise across different domains, such as TV broadcasts and telephone calls, or across different noisy environments. Furthermore, supervised learning tends to ignore the rich underlying structure of audio, which self-supervised learning takes advantage of.
单纯依靠监督学习会带来任务特定解决方案的风险,其中模型可能难以在不同领域(例如电视广播和电话呼叫)或不同嘈杂环境中进行概括。 此外,监督学习往往会忽略音频的丰富底层结构,而自我监督学习正是利用了这种底层结构。
There are two main forms of self-supervised learning [7]:
自我监督学习有两种主要形式[7]:
- Generative — focus on minimising a reconstruction error, therefore, the loss is measured in the output space. 生成式-专注于最小化重建误差,因此,在输出空间中测量损耗。
- Contrastive — strives to be able to single out a positive sample from a set of distractors that correspond to different segments of audio. The loss is measured in the representation space. 对比-努力能够从与音频的不同片段相对应的一组干扰因素中挑选出一个正样本。 损失是在表示空间中测量的。
流行的方法 (Popular approaches)
A popular generative self-supervised approach is Autoregressive Predictive Coding (APC [9]). Once the raw audio is converted to Fbanks, the task is to predict the feature vector N time steps in the future given the features before that time step, where the range 1 ≤ N ≤ 10 gives rise to good representations. The past context is summarised by a recurrent neural network (RNN) or transformer [10] and the activations of the final layers are taken as the representations to be used. The loss is mean squared error with respect to the reference. Recent work adds a vector quantised layer [11] to improve results on phone/speaker identification further.
流行的生成式自我监督方法是自回归预测编码(APC [9])。 一旦原始音频被转换为Fbanks,任务是预测时间步长,其中,所述范围为1≤N≤10产生了良好的表示之前给出的特征在未来特征向量N个时间步骤。 过去的上下文由递归神经网络(RNN)或变换器[10]概括,最后层的激活被用作要使用的表示。 损耗是相对于参考的均方误差。 最近的工作增加了矢量量化层[11],以进一步改善电话/扬声器识别的结果。
One form of contrastive self-supervised learning, and the one used in this work, is CPC. The raw data is encoded to a latent space and the task is to classify positive and negative samples correctly in this space. The loss used here is called InfoNCE. A more detailed summary of CPC is in the next section.
CPC是一种对比性的自我监督学习形式,它是这项工作中使用的一种形式。 原始数据被编码到一个潜在空间中,任务是在该空间中正确地对正样本和负样本进行分类。 这里使用的损失称为InfoNCE。 下一部分将对CPC进行更详细的总结。
Other competitive methods include Momentum Contrast (MoCo [12]) and Problem-Agnostic Speech Encoder (PASE [13]). The latter uses emotion recognition as one of the workers to push relevant information into the representations.
其他竞争方法包括动量对比度(MoCo [12])和与问题无关的语音编码器(PASE [13])。 后者使用情感识别作为将相关信息推入表示形式的工作者之一。
对比预测编码 (Contrastive Predictive Coding)
An overview of CPC is given in Figure 1 and this section describes how it works. I have provided some screenshots of PyTorch code to illustrate the method— these are simplified compared to the full code given in the project repository.
CPC的概述如图1所示,本节介绍其工作方式。 我提供了一些PyTorch代码的屏幕快照以说明该方法,与项目存储库中提供的完整代码相比,这些屏幕快照得到了简化。
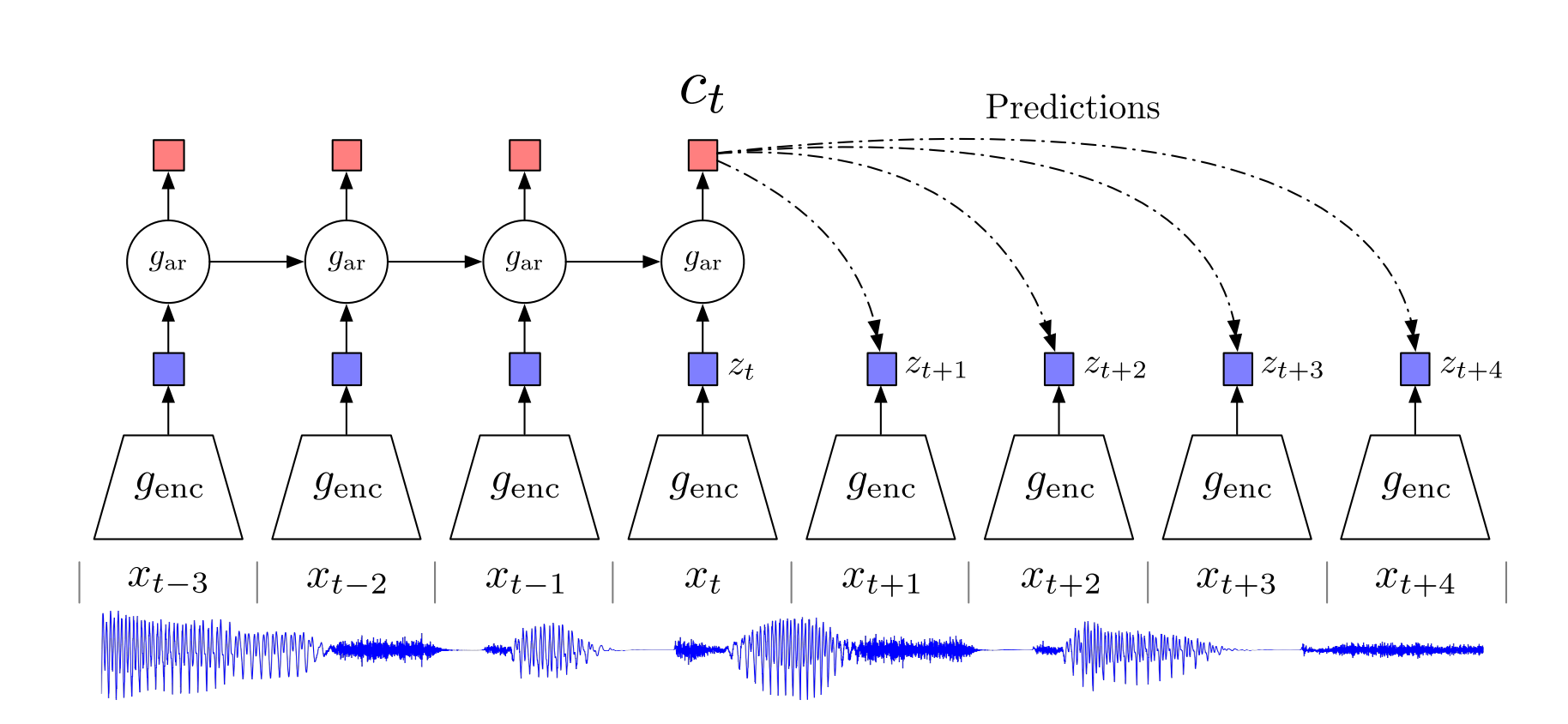
Firstly, raw audio samples, x, at 16kHz are passed through an encoder (g_enc) which down-samples the audio by a factor of 160 with multiple convolutional layers. Therefore, the output frequency of the encoder is 100Hz. Alternatively, the encoder can instead be replaced with a multi-layer perceptron that operates on Fbank features that are already at 100Hz. The second method is used as a baseline in [9] and is used in this work since I found it gives small gains in performance when using the learnt features for downstream tasks.
首先,原始音频样本x在16kHz处通过编码器(g_enc),该编码器使用多个卷积层对音频进行160倍的下采样。 因此,编码器的输出频率为100Hz。 另外,编码器也可以用多层感知器代替,该感知器可在已经以100Hz频率运行的Fbank功能上运行。 第二种方法在[9]中用作基准,并且在本工作中使用,因为我发现在将学习到的功能用于下游任务时,该方法的性能会有很小的提高。
The output of the encoder, z, in the latent space are fed into an autoregressive model g_ar (such as an RNN). This stage outputs c at each time step which combines the information in all previous latents. The forward method in Figure 2, illustrates how this is implemented in PyTorch.
潜在空间中的编码器z的输出被馈送到自回归模型g_ar(例如RNN)。 此阶段在每个时间步输出c,它将所有先前潜在信息合并在一起。 图2中的forward方法说明了如何在PyTorch中实现该方法。
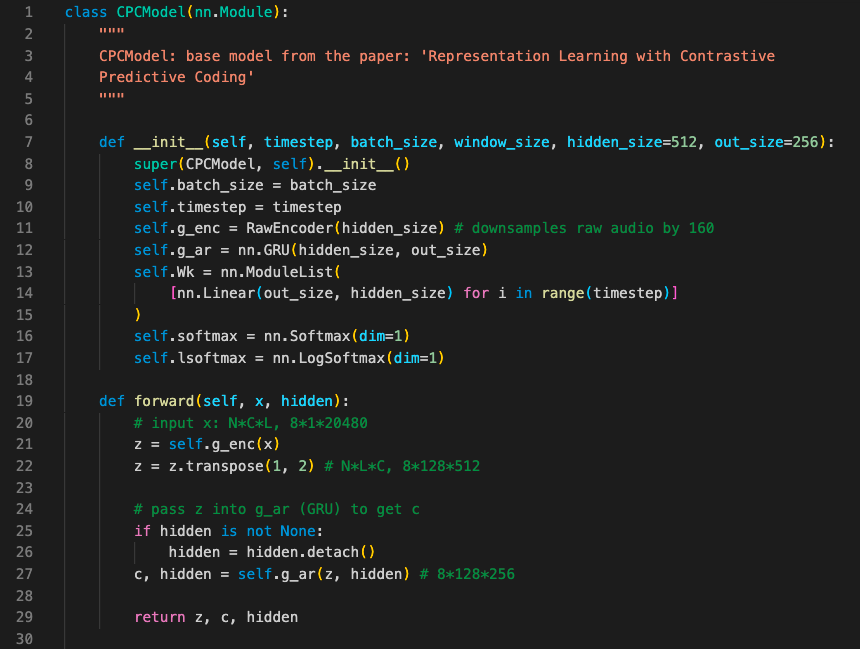
Now, at a specific time step t, a linear transform of c is applied with a weight matrix associated with how far ahead you are predicting (shown by the dotted lines in Figure 1 and by the code on line 36 in Figure 3). Next, these linear transforms are multiplied by the actual future latent z to give a log density ratio. The density ratio is defined in the equation below:
现在,在特定的时间步长t处,对c进行线性变换,并将权重矩阵与您预测的前进距离相关联(如图1中的虚线和图3中的第36行的代码所示)。 接下来,将这些线性变换乘以实际的未来潜在z,以得出对数密度比。 密度比在以下公式中定义:

A Softmax layer is applied over the log density ratio from a positive sample and many negative samples. The aim is to increase the probability of the positive sample or, in other-words, be able to understand that the positive latent is the one that is most related to the history. This is repeated k times as shown by the loop over each time step in Figure 3.
在正样本和许多负样本的对数密度比上应用Softmax层。 目的是增加阳性样本的可能性,或者换句话说,能够了解阳性潜伏期与历史最相关。 如图3中每个时间步的循环所示,将其重复k次。
“The training objective requires identifying the correct quantized latent audio representation in a set of distractors” — excerpt from wav2vec 2.0 [14]
“训练目标要求在一组分散器中识别正确的量化潜在音频表示形式” — wav2vec 2.0摘录[14]
The loss in CPC is known as InfoNCE given in the equation below and it is the same as the categorical cross-entropy of classifying the positive sample correctly.
CPC的损失称为下式中给出的InfoNCE,它与正确分类正样本的分类交叉熵相同。

In practice the loss is calculated by summing the log probability of the positive samples within the batch (line 57 in Figure 3). The encoder, RNN and weight matrices are all trained in parallel when maximising this loss.
在实践中,损失是通过将批次中阳性样本的对数概率相加来计算的(图3中的第57行)。 当使这种损失最大化时,将并行训练编码器,RNN和权重矩阵。
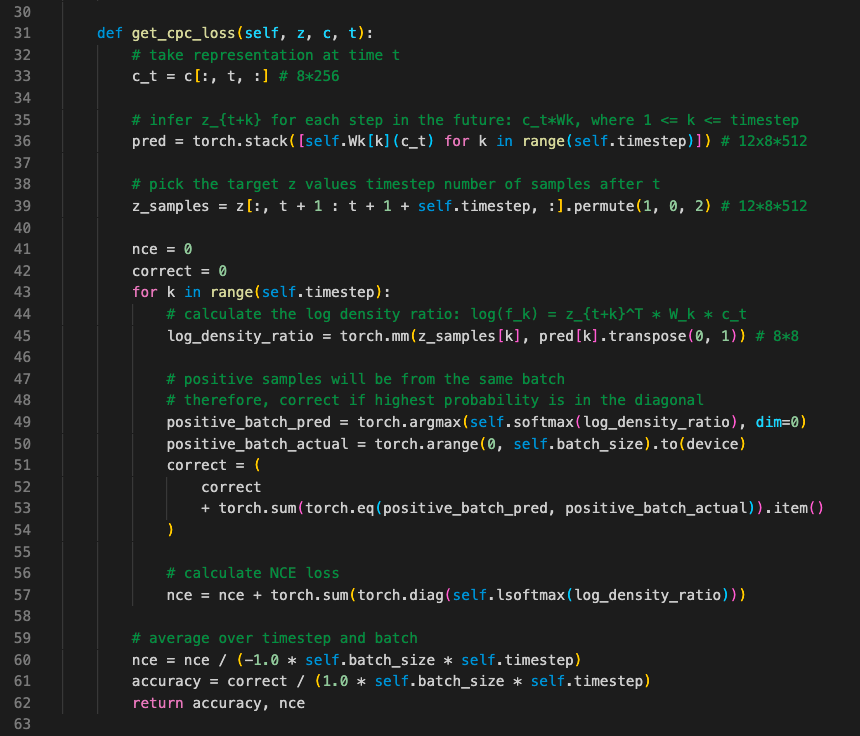
The code given in Figure 4, gives an example of the model being initialised, how data is passed through and calculating InfoNCE loss at multiple times in the sequence. It is worth understanding that there are loops over the time ‘t’ in the sequence to predict from and also the number of time steps ‘k’ to predict ahead for.
图4中给出的代码给出了一个示例,该模型正在初始化,如何通过数据并按顺序多次计算InfoNCE损失。 值得理解的是,序列“ t”中存在循环,可以从中进行预测,还可以预测时间步长“ k”。

数据集 (Datasets)
The CPC pre-training is trained on a 100-hour subset of the Librispeech dataset [17] comprising of 16kHz English speech.
CPC预训练是在Librispeech数据集[17]的100小时子集中进行训练的,该子集包含16kHz英语语音。
The dataset used for the emotion recognition task is called “The Ryerson Audio-Visual Database of Emotional Speech and Song” (RAVDESS, [16]). Only the speech dataset is considered in my work. It comprises of 24 speakers with an even split of male and female actors. There are eight emotions used to read out specific sentences, namely: neutral, calm, happy, sad, angry, fearful, surprise, and disgust.
用于情感识别任务的数据集称为“ 情感语音和歌曲的Ryerson视听数据库 ”(RAVDESS,[16])。 在我的工作中只考虑语音数据集。 它由24位发言人组成,男女演员均分。 有八种情绪可以用来读出特定的句子,即:中立,镇定,快乐,悲伤,愤怒,恐惧,惊奇和厌恶。
I chose to split the final two actors evenly between the validation and test set. In addition, audio files are randomly chosen from the other actors and added to ensure 80% of the data is used in the training set, leading to a classic 80:10:10 split.
我选择在验证和测试集之间平均分配最后两个参与者。 此外,音频文件是从其他演员中随机选择并添加的,以确保训练集中使用了80%的数据,从而实现了经典的80:10:10分割。
Related work in [6] uses the RAVDESS data and chooses to classify male and female emotions separately. Furthermore, they illustrate results when limiting the number of emotion classes, which makes the task easier. In this work, I kept the task true to the original data, so the models are trained to classify all eight emotions.
文献[6]中的相关工作使用RAVDESS数据,并选择对男性和女性情绪分别进行分类。 此外,它们还说明了限制情感类别数量时的结果,这使任务更加容易。 在这项工作中,我将任务与原始数据保持一致,因此训练了模型以对所有八种情绪进行分类。
方法 (Method)
CPC系统 (CPC system)
The self supervised pre-training step used to generate features for emotion recognition training is outlined in [9] as the CPC baseline. This is used instead of the convolutional feature encoder that operates on the raw waveform as in [8] since I found it marginally improves results. The standard 80 dimensional Fbanks were used as input features, which are passed through a 3 layer MLP encoder with a hidden size of 512, batch norm and ReLU activations. The output from the feature encoder (z) is fed through a single GRU layer with an output size of 256 to give the contextual feature vectors (c). These are used as representations for training the emotion recognition model.
[9]中概述了用于生成用于情感识别训练的特征的自我监督的预训练步骤,将其作为CPC基准。 因为我发现它在一定程度上改善了结果,所以它代替了[8]中对原始波形进行操作的卷积特征编码器。 标准的80维Fbank被用作输入要素,这些要素通过3层MLP编码器传递,其隐藏大小为512,批处理规范和ReLU激活。 来自特征编码器(z)的输出通过单个GRU层馈入,输出大小为256,以提供上下文特征向量(c)。 这些用作训练情绪识别模型的表示。
The CPC system was trained with a window size of 128 (corresponding to 1.28s since Fbanks are at 100Hz), a batch size of 64 and 500k steps. This amounts to approximately 114 epochs of the Librispeech 100 hour dataset (used in [8]). The RAdam optimizer was used with a flat learning rate of 4e-4 for the first two thirds of training before being cosine annealed to 1e-6. A total horizon of 12 timesteps in the future (k) was used since it has been shown to give the highest accuracy of discriminating the positive from the negative samples in the CPC task [8].
每次点击费用系统的训练窗口大小为128(Fbank频率为100Hz时对应于1.28s),批量大小为64和500k步长。 这大约相当于Librispeech 100小时数据集的114个纪元(用于[8])。 在进行余弦退火到1e-6之前的前三分之二的训练中,使用RAdam优化器以4e-4的固定学习率进行训练。 由于已显示在CPC任务中可以最好地区分正样本和负样本,因此使用了未来12个时间步长(k)的总水平[8]。
情绪识别系统 (Emotion recognition system)
In addition, I used a variety of architectures for the emotion recognition model in order to investigate the accessibility of representations as well as pushing the performance of the system. The list below gives more details on the 7 types of architecture used. All models have global normalisation applied to the input features and the code can be found here.
此外,我对情绪识别模型使用了多种体系结构,以便研究表示的可访问性并提高系统的性能。 下面的列表提供了有关所使用的7种体系结构的更多详细信息。 所有模型都将全局规范化应用于输入功能,并且代码可以在此处找到。
- Linear — single linear layer. 线性-单个线性层。
- MLP-2 — multi layer perceptron with 2 blocks. Each block contains a linear layer (hidden size 1024), batch norm, ReLU activation and dropout (prob 0.1). MLP-2 —带有2个块的多层感知器。 每个块包含一个线性层(隐藏大小为1024),批处理规范,ReLU激活和退出(概率0.1)。
- MLP-4 — same as above but with 4 blocks. MLP-4-与上面相同,但有4个块。
- RNN (uni-dir) — 2 layers, not bi-directional, hidden size 512, dropout prob 0.1 RNN(uni-dir)-2层,非双向,隐藏大小512,丢失概率0.1
- Convolutional — same structure as [6], with 6 convolutional layers, ReLU activations, dropout prob 0.1 and a max pooling layer. 卷积-与[6]相同的结构,具有6个卷积层,ReLU激活,辍学概率0.1和最大池化层。
- WaveNet — dilated convolutional structure that grows exponentially as in [15]. Hyper parameters are hidden size 64, dilation depth 6, number of repeats 5, kernel size 2. WaveNet —膨胀的卷积结构,如[15]所示呈指数增长。 超参数的隐藏大小为64,扩展深度为6,重复次数为5,内核大小为2。
- RNN (bi-dir) — same as RNN but bi-directional. RNN(bi-dir)-与RNN相同,但是双向的。
Each model was trained using a window size of 1024 (10.24s), batch size of 8 and a total of 40k steps. A frame-wise cross entropy loss was used over the eight emotions. The optimizer and learning rate is unchanged compared to CPC training, however, the learning rate schedule off is turned off.
使用1024(10.24s)的窗口大小,8的批量大小和总共40k步长训练每个模型。 在八种情绪上使用了逐帧交叉熵损失。 与CPC培训相比,优化程序和学习率没有变化,但是关闭了学习率计划。
A baseline emotion recognition model without CPC pre-training, that uses Fbanks as feature vectors, is used for comparison throughout my analysis.
在我的整个分析过程中,都使用了不使用CPC预训练的基线情感识别模型(以Fbanks作为特征向量)进行比较。
结果 (Results)
每次点击费用的影响 (Impact of CPC)
A linear architecture is often used in self-supervised learning literature to illustrate the accessibility of representations. In this work, I wanted to show that there is a boost even for more complex architectures, such as a WaveNet style model with dilated convolutions or a bi-directional RNN. Table 1 shows the frame-wise accuracy when using Fbanks and CPC features for each of the proposed architectures. In each case, CPC features result in an increase in accuracy when classifying the emotions in speech, irrespective of the architecture. The average decrease in relative error is 21.7%; in other words over a fifth of the errors disappear.
自我指导学习文献中经常使用线性体系结构来说明表示的可访问性。 在这项工作中,我想表明即使对于更复杂的体系结构(例如具有扩展卷积的WaveNet样式模型或双向RNN)也有促进作用。 表1列出了针对每个建议的体系结构使用Fbanks和CPC特征时的逐帧准确性。 在每种情况下,CPC功能都可以在对语音中的情感进行分类时提高准确性,而与架构无关。 相对误差平均下降21.7%; 换句话说,错误的五分之一消失了。
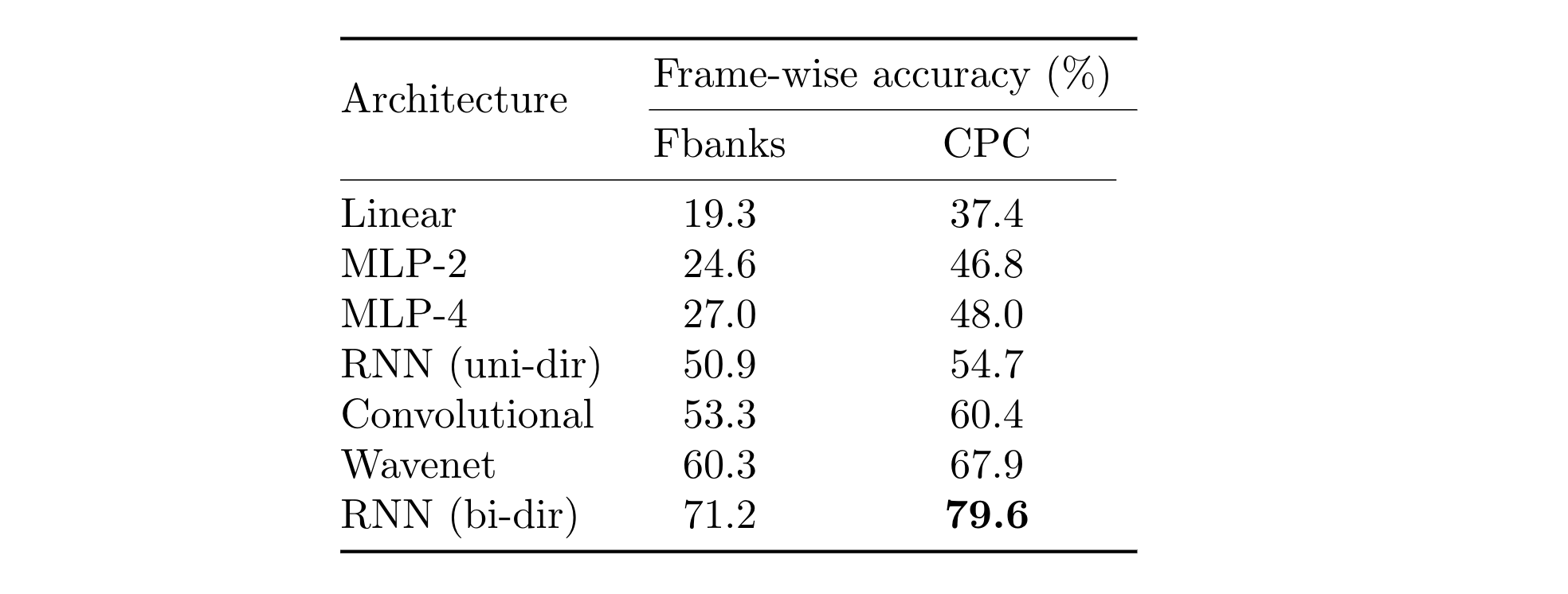
It is worth noting that, since the CPC representations have a larger feature dimension compared to Fbanks, emotion recognition models trained with CPC have an increased parameter count. However, after running some tests matching parameter counts, I established that the trend of Fbanks being outperformed still holds and the gap only narrowed a small amount.
值得注意的是,由于与Fbanks相比,CPC表示具有更大的特征维度,因此使用CPC训练的情绪识别模型具有增加的参数数量。 但是,在运行了一些与参数计数匹配的测试之后,我确定Fbanks表现优于大市的趋势仍然成立,差距仅缩小了很小的一部分。
建筑的影响 (Impact of architecture)
The three worst performing models in Table 1 do not utilise information across time — they attempt to classify the emotion given the representation of one frame only. Models that use a uni directional RNN or convolutional layers can take extra context into account, which makes a large difference, especially when using Fbanks. The WaveNet style model has a much larger receptive field compared to the normal convolutional model and this boosts performance further. One reason could be because it can look into the future since the convolutions are unmasked. Similarly to the WaveNet model, the bi-directional RNN can use context from the future and this architecture leads to the best emotion recognition performance when coupled with the CPC features. The frame-wise accuracy was 79.6% on the RAVDESS test set. As far as I am aware, this is state of the art for this task when classifying all eight emotions.
表1中三个性能最差的模型没有跨时间使用信息-它们仅根据一帧的表示尝试对情感进行分类。 使用单向RNN或卷积层的模型可以考虑额外的上下文,这有很大的不同,尤其是在使用Fbanks时。 与普通卷积模型相比,WaveNet样式模型具有更大的接收场,这进一步提高了性能。 原因之一可能是,由于卷积被掩盖了,它可以展望未来。 与WaveNet模型类似,双向RNN可以使用将来的上下文,并且当与CPC功能结合使用时,此体系结构可带来最佳的情感识别性能。 RAVDESS测试仪的逐帧准确性为79.6%。 据我所知,这是对所有八种情绪进行分类时的最新技术。
个人情绪 (Individual emotions)
Table 2 shows the frame-wise F1 scores for each of the emotions classified in the test set. The model is best at recognising the actors speaking in disgusted and surprised voices. Happy and neutral are the emotions it performs the worst on. This is likely because the latter are less expressive so the model finds it more difficult to classify.
表2列出了测试集中分类的每种情绪的逐帧F1得分。 该模型最适合识别演员以恶心和惊讶的声音讲话。 快乐和中立是它表现最差的情绪。 这可能是因为后者的表现力较差,因此该模型发现很难进行分类。

未来的工作 (Future work)
Future work could include swapping out the RNN in the CPC system with a transformer (this is done in [14]). This would allow me to scale up the CPC model and utilise more unlabelled data from a range of sources beyond Librispeech. In addition, data augmentation could be added to the emotion recognition data to improve robustness and perhaps boost results further.
未来的工作可能包括用变压器替换CPC系统中的RNN(在[14]中完成)。 这将使我能够扩展CPC模型并利用Librispeech以外的其他来源的更多未标记数据。 此外,可以将数据增强添加到情绪识别数据中,以提高鲁棒性,并可能进一步提高结果。
结论 (Conclusion)
Self-supervised learning, such as CPC, can be used to significantly reduce errors in the domain of emotion recognition in speech. A variety of architectures were tested in my work and I found the bi-directional RNN — which can utilise future context — led to the best performing model.
自我监督学习(例如CPC)可用于显着减少语音情感识别领域的错误。 在我的工作中测试了多种体系结构,我发现可以利用未来上下文的双向RNN导致了最佳性能模型。
This work is useful for benchmarking and improving speech representations trained using CPC, as well as boosting performance when classifying multiple emotions. A reason why all this is exciting is that it provides the building blocks for systems that can more reliably predict the sentiment of a person speaking. For example, this can lead to significantly improved quality of analysis tools in call centres used to help agents build their skills and improve the customer experience.
这项工作对于基准测试和改进使用CPC训练的语音表示形式,以及在对多种情感进行分类时提高性能很有用。 所有这些令人兴奋的原因在于,它为系统提供了构建模块,这些模块可以更可靠地预测讲话者的情绪。 例如,这可以显着提高呼叫中心中用于帮助座席提高技能并改善客户体验的分析工具的质量。
dsp出价