Authors: Zhidong Ke, Utsavi Benani, Heng Zhang, Kevin Terusaki, Yifeng Liu, Percy.Mehta, Priyadarshini Mitra, Jayanth Parayil Kumarji
作者:Kezhidong Ke,Utsavi Benani,Heng Zhang,Kevin Terusaki,Liu Feng,Percy.Mehta,Priyadarshini Mitra,Jayanth Parayil Kumarji
介绍 (Introduction)
To support our customers using High Velocity Sales to intelligently convert leads and create new opportunities, we built the engagement activity platform to automatically capture and store user engagement activities. The engagement delta lake is one of the key components supporting Einstein Analytics for creating powerful reports and dashboards and Sales Cloud Einstein for training machine learning models.
为了使用高速销售支持客户以智能方式转换销售线索并创造新的机会,我们构建了参与活动平台来自动捕获和存储用户参与活动。 参与三角洲湖是支持爱因斯坦分析(用于创建功能强大的报表和仪表板)和销售云爱因斯坦(用于培训机器学习模型)的关键组件之一。
Unlike data in our other data lakes, engagement activity is mutable and the mutation ratio is high, which creates a huge challenge for us. We explored a couple of solutions and ultimately decided that the open source tool Delta Lake met all of our requirements. In this blog, we will walk through our journey through the following sections:
与我们其他数据湖中的数据不同,参与活动是可变的,突变率很高,这给我们带来了巨大的挑战。 我们探索了两个解决方案,最终决定开源工具Delta Lake满足了我们的所有要求。 在此博客中,我们将通过以下部分逐步完成我们的旅程:
- Engagement activity ingestion 参与活动摄取
- Incremental Read增量读
- Support exact once write across tables一次支持跨表写入
- Handle mutation with cascading changes处理具有级联更改的突变
- Normalize tables in data lake在数据湖中标准化表
- Performance tuning性能调优
参与活动摄取(Engagement Activity Ingestion)
高层建筑(High Level Architecture)
Our engagement data lake is a distributed key-value object store which is similar to our existing data lake known as Shared Activity Store. To support the basic requirement from Delta Lake, the ingestion pipeline starts when data is pushed to our internal Kafka queue. Then we use Spark Streaming Framework to consume engagement in micro-batches and write the data to Delta Lake table(s),as Figure 1 shows below.
我们的参与数据湖是一个分布式键值对象存储,它与我们现有的数据湖类似,称为共享活动存储。 为了满足Delta Lake的基本要求,当数据被推送到我们的内部Kafka队列时,提取管道就会启动。 然后,我们使用Spark Streaming Framework消耗微批次中的参与并将数据写入Delta Lake表,如下图1所示。
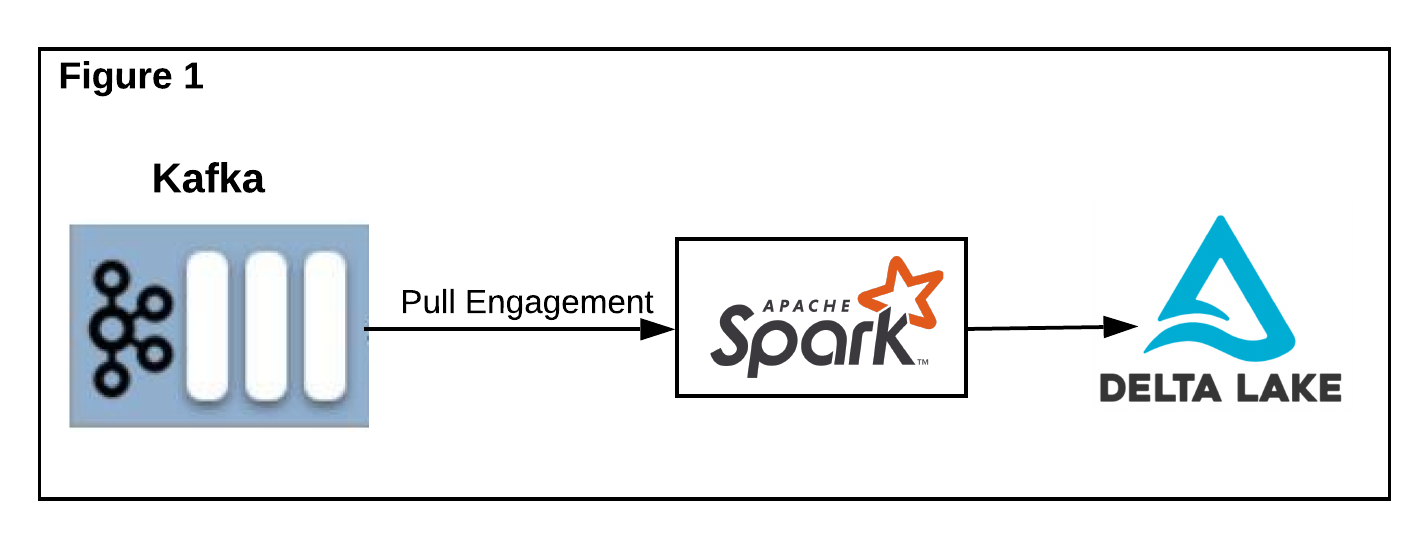
We start with a single table called Data Table, partitioned by organization ID and engagement activity timestamp to support basic queries, such as a time range query for our downstream consumers. However, our customers requested incremental read support. Delta Lake’s native API requires tables to be append-only, but ours is mutable, so we had to implement incremental read support on our own.
我们从一个称为数据表的表开始,该表按组织ID和参与活动时间戳划分,以支持基本查询,例如针对下游消费者的时间范围查询。 但是,我们的客户要求增加读取支持。 Delta Lake的本机API要求表只能是追加的,但是我们的表是可变的,因此我们必须自己实现增量读取支持。
增量读 (Incremental Read)
We created a separate table called Notification Table that was partitioned by organization ID and ingestion timestamp. When new engagement is inserted into Data Table or when existing engagement is updated or deleted, we notify downstream consumers of those changes by inserting a new entry with the engagement metadata into the notification table, as Figure 2 shows below.
我们创建了一个单独的表,称为通知表,该表按组织ID和摄取时间戳进行了分区。 当新的活动插入到数据表中或现有的活动被更新或删除时,我们通过将带有活动元数据的新条目插入通知表来通知下游消费者这些更改,如下图2所示。
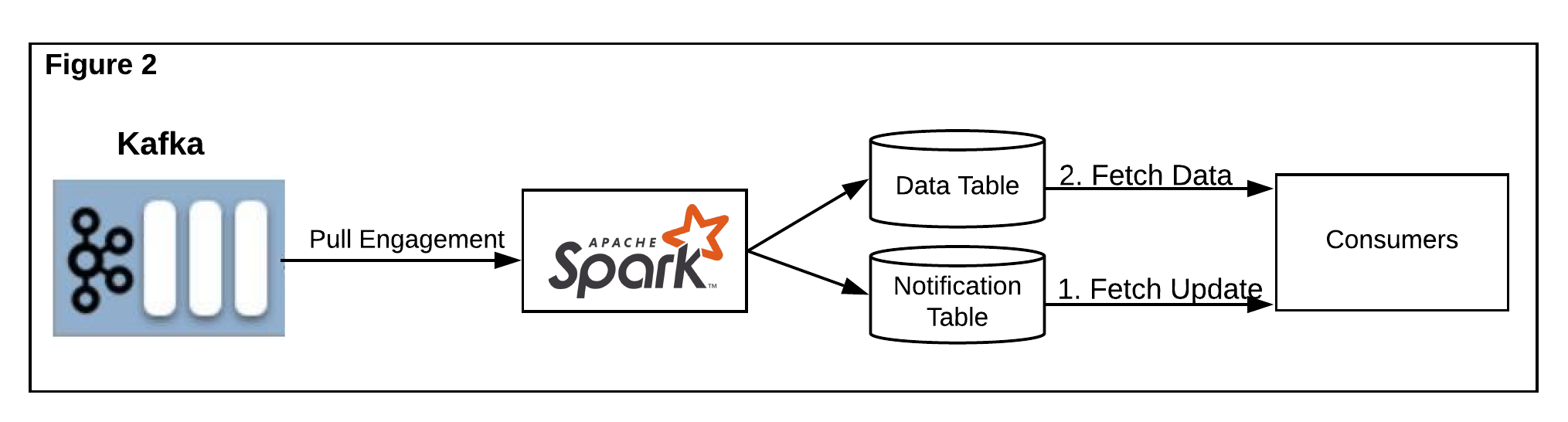
The downstream consumers can either use streaming mode to listen to Notification Table, or they can periodically pull new notifications from the Notification Table. Based on the value of the data, e.g. organization ID or engagement date, they are able to fetch the actual engagement data from our Data Table.
下游使用者可以使用流模式来侦听通知表,也可以定期从通知表中提取新通知。 基于数据的值,例如组织ID或参与日期,他们能够从我们的数据表中获取实际参与数据。
跨表精确写入 (Exact Once Write Across Tables)
While introducing the Notification Table solves our incremental read problem, it creates another challenge for us. We found that when our job tries to process and write data to two tables, Delta API only supports ACID transactions within each table; there is no batch transaction support across tables. For example, if the job writes to Data Table successfully but fails to write to Notification Table, the downstream completely misses this batch since it doesn’t get a notification. This becomes a common problem across our project when a job needs to write to multiple tables in a batch.
在引入通知表解决了我们的增量读取问题的同时,它给我们带来了另一个挑战。 我们发现,当我们的工作尝试处理数据并将其写入两个表时,Delta API仅支持每个表内的ACID事务; 没有跨表的批处理事务支持。 例如,如果作业成功写入数据表,但未能写入通知表,则下游将完全错过该批处理,因为它没有得到通知。 当作业需要批量写入多个表时,这成为我们项目中的常见问题。
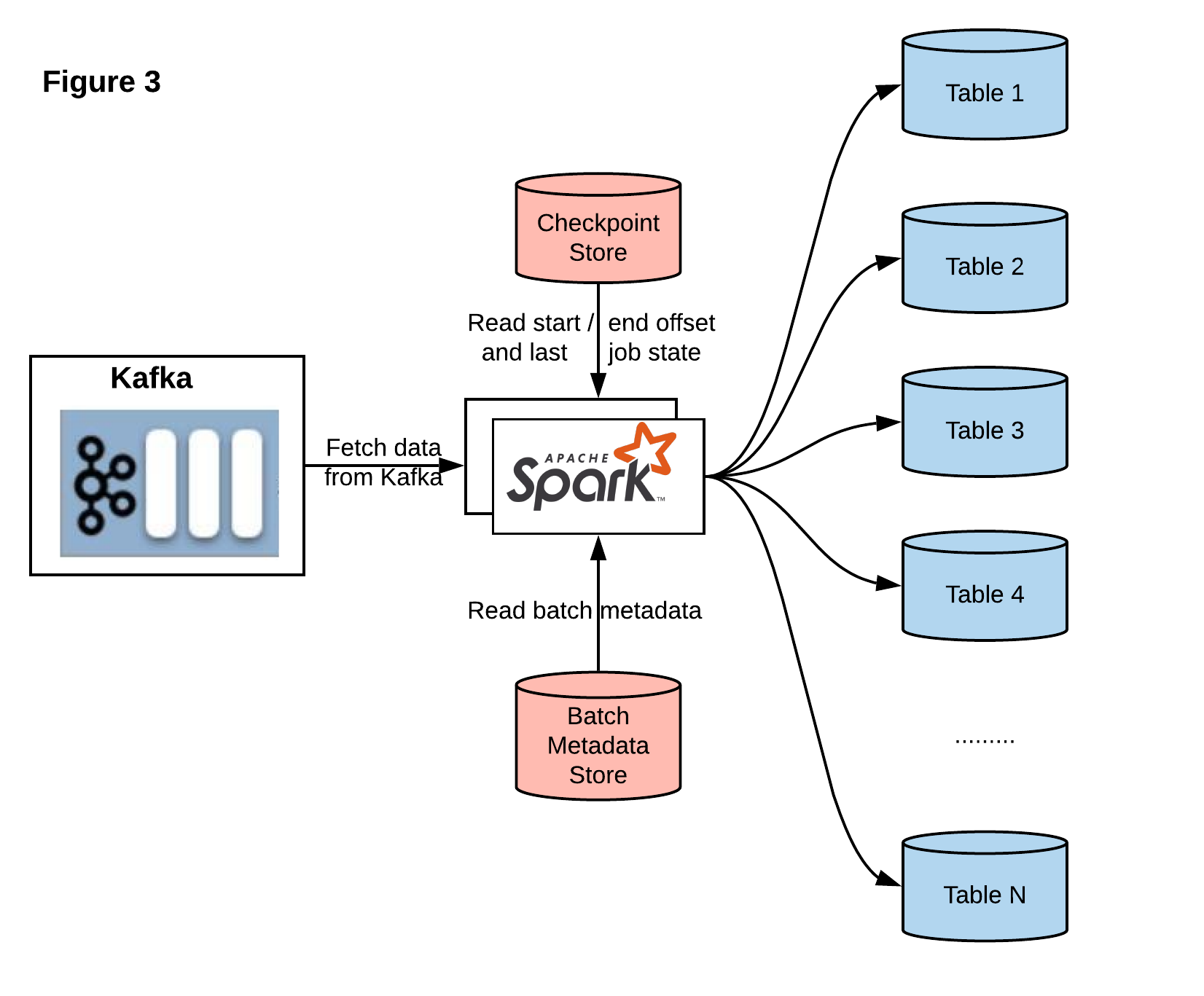
So, we created a Checkpoint Store that stores the start, end offset, Kafka metadata, and last job state for a given checkpoint. The first task of the Spark job, then, is to read this store to get the last checkpoint metadata and the last job state. Since our job executes in a micro-batch mode, and each batch includes multiple processes (process or write data to Table 1, Table 2, Table 3, Table 4 and so on), we also need to store the batch state. We created a Batch Metadata Store to store job name, batch ID (the last succeed batch ID provided by the Spark foreachBatch API), process name, and timestamp of last modified time. After reading from the Checkpoint Store, the Spark job will get the last job state. If the last batch completed successfully, the Spark job will increase the last batch ID; otherwise, it will keep the last batch ID. Then the Spark job fetches the Batch metadata based on the job name. By comparing the batch ID provided from the Spark job to the one from our Batch Metadata Store, we will know which process succeeded or failed. (If batch ID matches, it indicates success; otherwise, it indicates a failure.) If all processes succeed, we will read the data from Kafka based on the start offset and fetch new data. Otherwise, we will read a certain amount of data based on the start and end offset from Kafka, and resume from the last failed process by skipping the ones with matched batch ID. Our job will execute the processes in sequence across tables: process/write data to Table 1, Table 2, Table 3, Table 4 and so on. After each process completes, it will update the Batch Metadata Store with the current batch ID and timestamp. After the completion of all processes, the job will update the Checkpoint Store with the offset and the job state including the batch ID. The following Figure 4 shows the complete workflow:
因此,我们创建了一个检查点存储,用于存储给定检查点的开始,结束偏移,Kafka元数据和最后一个作业状态。 然后,Spark作业的第一个任务是读取此存储,以获取最后的检查点元数据和最后的作业状态。 由于我们的工作是在微批处理模式下执行的,并且每个批处理都包含多个进程(将数据处理或写入表1,表2,表3,表4等),所以我们还需要存储批处理状态。 我们创建了一个批处理元数据存储,以存储作业名称,批处理ID(Spark foreachBatch API提供的最后一个成功的批处理ID),进程名称和上次修改时间的时间戳。 从Checkpoint存储读取后,Spark作业将获得上一个作业状态。 如果最后一批成功完成,Spark作业将增加最后一批ID;否则,Spark作业将增加最后一批ID。 否则,它将保留最后的批次ID。 然后,Spark作业将根据作业名称获取批处理元数据。 通过将Spark作业提供的批次ID与我们的批次元数据存储中的批次ID进行比较,我们将知道哪个进程成功或失败。 (如果批次ID匹配,则表示成功;否则,则表示失败。)如果所有进程都成功,则我们将基于起始偏移量从Kafka读取数据并获取新数据。 否则,我们将根据Kafka的开始和结束偏移量读取一定数量的数据,并通过跳过匹配批次ID的数据从上一个失败的进程中恢复。 我们的工作将跨表依次执行这些过程:将数据处理/写入表1,表2,表3,表4等。 每个过程完成后,它将使用当前的批次ID和时间戳更新批次元数据存储。 所有过程完成后,作业将使用偏移量和作业状态(包括批次ID)更新Checkpoint存储。 下图4显示了完整的工作流程:
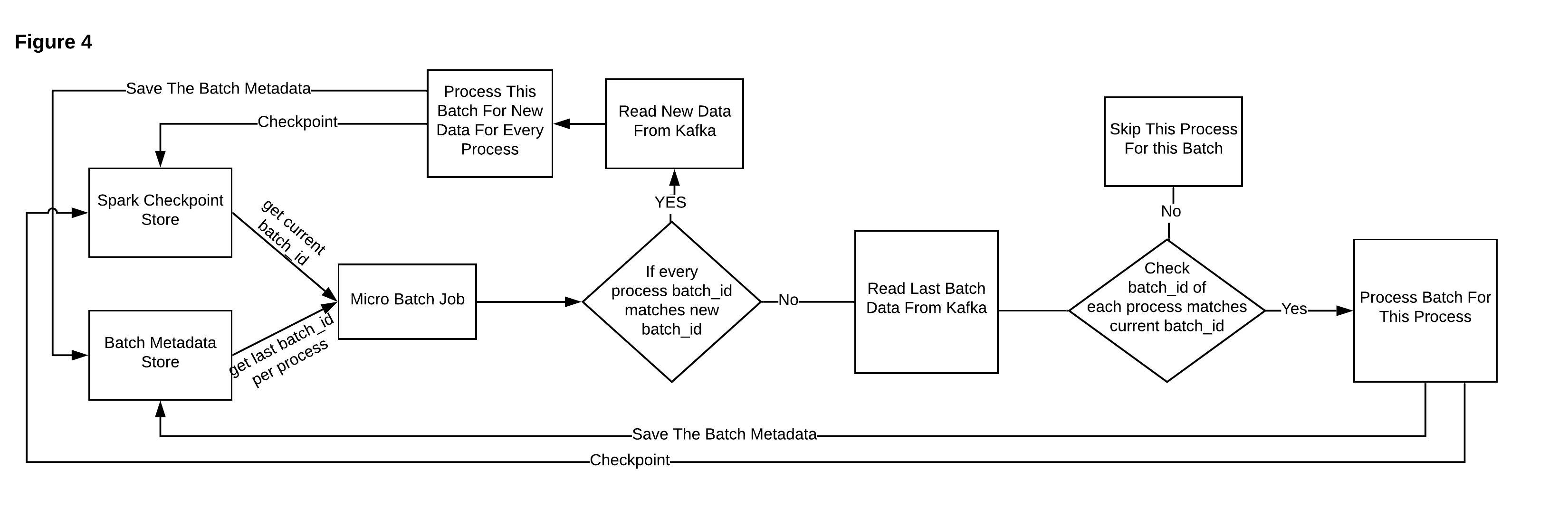
在Data Lake中处理突变 (Handling Mutation In Data Lake)
Our engagement data is mutable, which means we not only need to support insertion but also need to support update and deletion in data lake. As we all know, mutation operation is a much more expensive operation than insertion in batch jobs. Furthermore, due to the nature of the engagement data, the mutation request could be cascaded, and our mutation volume is almost 20% of insertion volume. So the goal for us is to reduce the mutation operation as much as possible in each batch.
我们的参与数据是可变的,这意味着我们不仅需要支持插入,而且需要支持数据湖中的更新和删除。 众所周知,变异操作比在批处理作业中插入要昂贵得多。 此外,由于参与数据的性质,突变请求可以级联,我们的突变量几乎是插入量的20%。 因此,我们的目标是尽可能减少每批中的突变操作。
使用图形检测级联突变 (Use Graph To Detect Cascading Mutation)
One simple example for cascading mutation is id1→id2, id2→id3, id3→id4. If we receive such a request in one batch and just execute the update query, the output will be incorrect, e.g. id1 will be id2, but we expect it to be id4. In order to execute them in a batch against our data lake, we will need to pre-process mutation requests. As Figure 5 shows, we break it down into several steps:
级联突变的一个简单示例是id1→id2,id2→id3,id3→id4。 如果我们分批收到这样的请求,而只是执行更新查询,则输出将不正确,例如id1将是id2,但我们希望它是id4。 为了针对我们的数据湖批量执行它们,我们将需要预处理变异请求。 如图5所示,我们将其分为几个步骤:
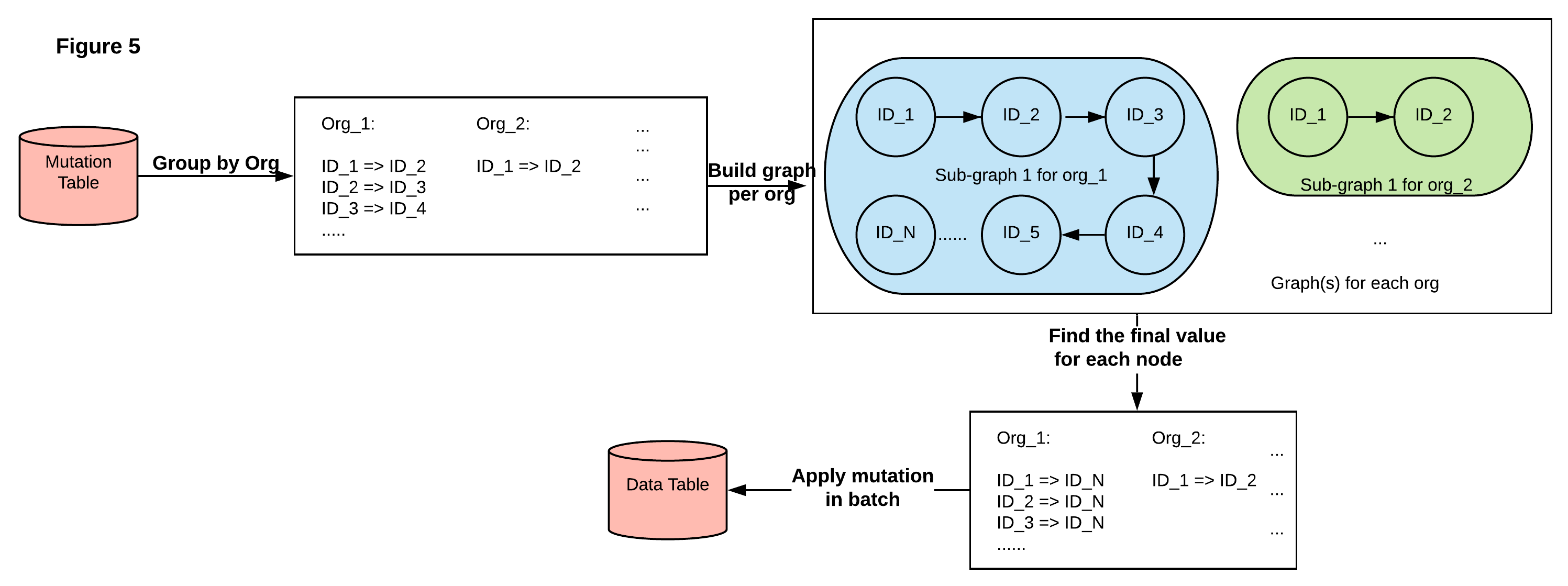
- We read the mutation request from the mutation table in a batch and group them by organization and sorted by mutation execution time. 我们从突变表中批量读取突变请求,并按组织将它们分组并按突变执行时间进行排序。
- For each group, we use Spark Graph API to build direct graph(s) where the node is the IDs and the edge is the request, and we find all connected components. 对于每个组,我们使用Spark Graph API构建直接图形,其中节点是ID,边缘是请求,我们找到所有连接的组件。
- We find the final state of each node; the process goes through each node in each sub-graph, and, based on the execution time, finds its corresponding final node. 我们找到每个节点的最终状态; 该过程遍历每个子图中的每个节点,并根据执行时间找到其对应的最终节点。
- Finally, we convert each connected component into a list request for each organization, then apply the components in a batch. 最后,我们将每个连接的组件转换为每个组织的列表请求,然后批量应用这些组件。
在Data Lake中标准化表 (Normalize Table In Data Lake)
As we mentioned, the mutation request volume is high, which impacts our performance a lot. We took a closer look into our data shape and found that a lot of records need to be mutated for any one request. For example, a request to change t_name_1 to t_name_2 can touch thousands of records because we store the t_name for each record (thanks to our “flatten data” scheme design).
正如我们提到的,变异请求量很大,这对我们的性能有很大影响。 我们仔细研究了数据的形状,发现对于任何一个请求都需要对许多记录进行突变。 例如,将t_name_1更改为t_name_2的请求可能涉及成千上万条记录,因为我们为每条记录存储了t_name(这要归功于“扁平化数据”方案设计)。
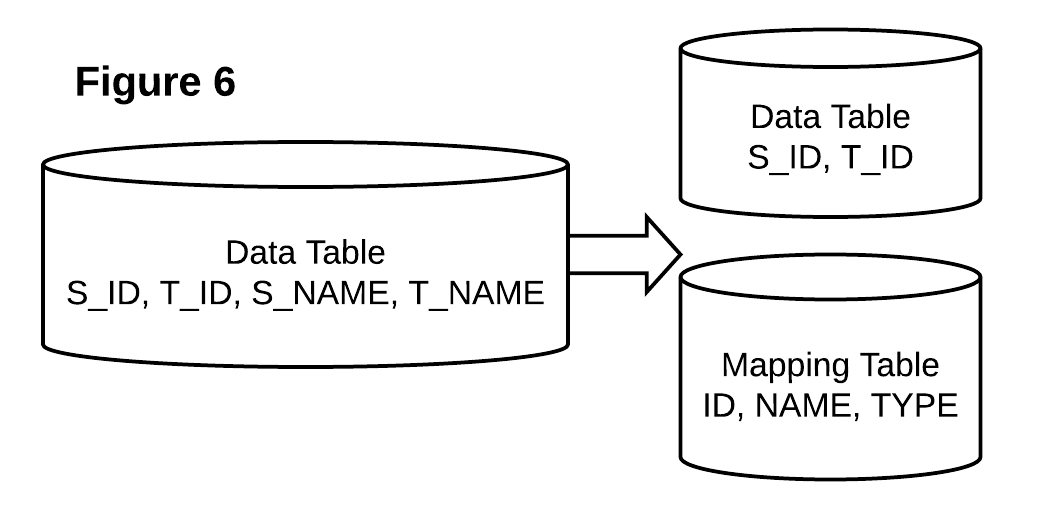
As the Figure 6 shows, we extract out the “NAME” fields from our data table into a separate table called mapping table, and, in this table, we store the mapping from ID to name. By changing our original design, we also need to add an extra write in the ingestion job and an extra update in the mutation job for both data table and mapping table. But we do see a big performance jump since we significantly reduce the number of records/files updates in each mutation batch.
如图6所示,我们从数据表中提取“ NAME”字段到另一个称为映射表的表中,并在此表中存储从ID到名称的映射。 通过更改原始设计,我们还需要为数据表和映射表在摄取作业中添加额外的写入,并在变异作业中添加额外的更新。 但是我们确实看到了性能上的飞跃,因为我们大大减少了每个突变批次中的记录/文件更新数量。
性能调优 (Performance Tuning)
Finally, we’ve solved most of our design challenges and have reached the performance tuning stage. Based on the performance reports for our ingestion and mutation jobs, we achieved product SLA on the ingestion part. But addressing the performance issues in the mutation job took much longer than we expected. So we looked into the DeltaLog and ran the analysis against our tables and found the following issues:
最后,我们已经解决了大多数设计难题,并且已经进入性能调整阶段。 根据摄入和突变工作的绩效报告,我们在摄入部分获得了产品SLA。 但是解决变异工作中的性能问题所需的时间比我们预期的要长得多。 因此,我们调查了DeltaLog并针对我们的表进行了分析,发现了以下问题:
- Due to the partition scheme (organization_id/engagement_date) and traffic across many thousands of organizations, there are many small files (in MBs or KBs) in certain partitions, which caused the slow mutation operation. 由于分区方案(organization_id / engagement_date)和跨数千个组织的流量,某些分区中有许多小文件(以MB或KB为单位),这导致了缓慢的变异操作。
- Our mutation job doesn’t repartition by the partition key before it writes, so many tasks are writing to the same partition and slowing down the write process. 我们的变异作业在写入之前不会按分区键重新分区,因此许多任务正在写入同一分区,从而减慢了写入过程。
- Spark set the default spark.default.parallelism to 200, which limited our join and read parallelism. Spark将默认的spark.default.parallelism设置为200,这限制了我们的联接和读取并行度。
To address those issue, we applied some fixes:
为了解决这些问题,我们应用了一些修复程序:
- We compacted those small files by running the “OPTIMIZE” table command provided by Delta Lake. 我们通过运行Delta Lake提供的“ OPTIMIZE”表命令来压缩这些小文件。
- We added one more stage at the end of our mutation job to partition by organization_id and engagement_date to make sure only one task is writing to a partition. 在变异工作结束时,我们又增加了一个阶段来按organization_id和engagement_date进行分区,以确保只有一个任务正在写入分区。
- We increased the spark.default.parallelism to 400. 我们将spark.default.parallelism增加到400。
After those changes, we saw a performance gain while running the performance test, but it still didn’t meet our goal, so further optimization is required. We started experimenting with the partition scheme to only partition by organization_id, since this is the only way we can improve the file distribution, but we also needed to pay attention to downstream consumer read performance (most queries are based on organization_id and date). We ran an AB test against two partition schemes:
进行这些更改之后,我们在运行性能测试时看到了性能提升,但仍未达到我们的目标,因此需要进一步优化。 我们开始尝试分区方案以仅按organization_id进行分区,因为这是我们可以改善文件分发的唯一方法,但是我们还需要注意下游使用者的读取性能(大多数查询基于organization_id和date)。 我们针对两个分区方案进行了AB测试:
- We created a new table with organization_id as the partition key and loaded the same amount of data as the original table. 我们创建了一个以organization_id作为分区键的新表,并加载了与原始表相同的数据量。
- We ran the OPTIMIZE command with z-ordering by engagement_date to improve read performance. 我们对engagement_date进行了z排序的OPTIMIZE命令,以提高读取性能。
- We analyzed the file size per organization and compared the read/update/insert operation time. 我们分析了每个组织的文件大小,并比较了读取/更新/插入操作时间。
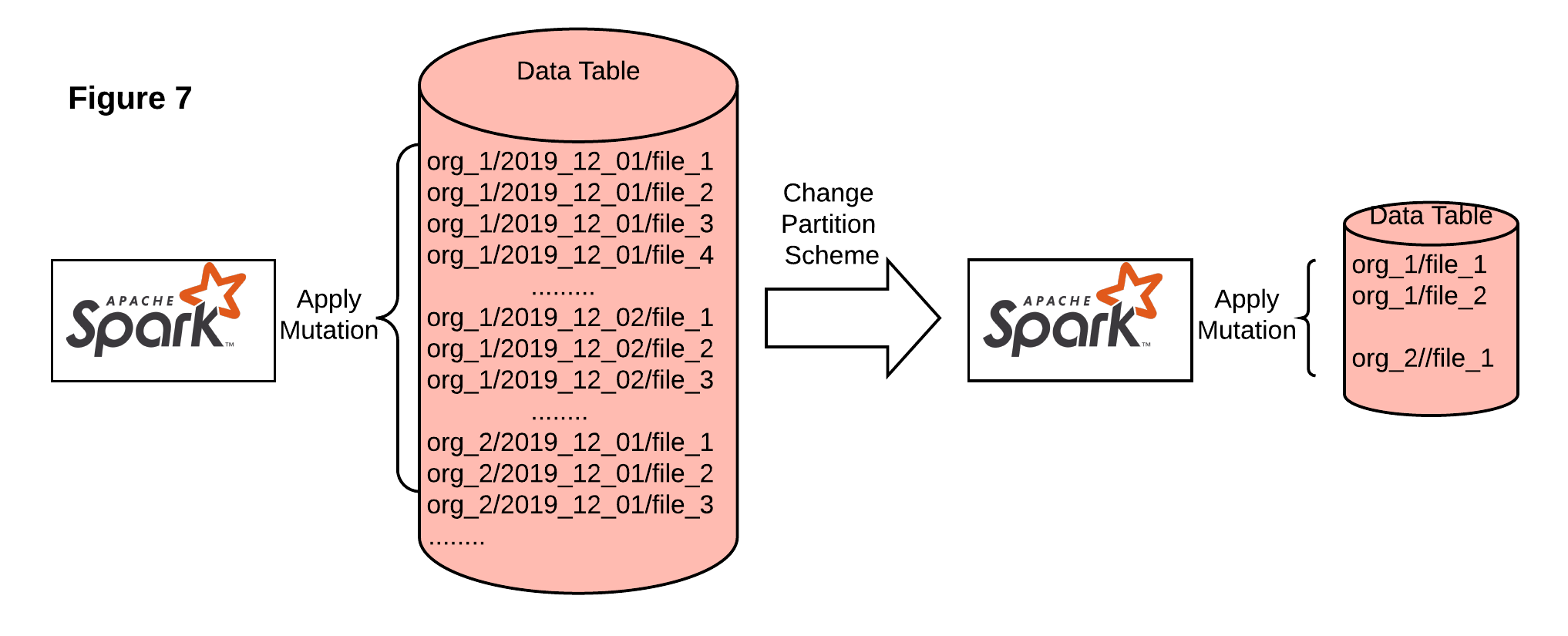
To our surprise, we saw a more than 50% read performance gain, more than 300% update performance improved, and 10% more time for insert (since we added an extra job for OPTIMIZE). This is a huge performance improvement for our data lake, and we summarized the reasons as follows:
令我们惊讶的是,我们看到读取性能提高了50%以上,更新性能提高了300%以上,插入时间增加了10%(因为我们为OPTIMIZE添加了额外的工作)。 对于我们的数据湖来说,这是巨大的性能改进,我们总结了以下原因:
- The mutation requests in each batch are going to touch a big time window, which means they will need to touch a large amount of partitions if we have date as sub-partition. 每个批次中的突变请求都将涉及一个较大的时间窗口,这意味着如果我们将日期作为子分区,则它们将需要触摸大量分区。
- By changing the partition scheme to organization_id, it highly reduces the I/O in both read/write/update. 通过将分区方案更改为organization_id,它极大地减少了读/写/更新的I / O。
- The Delta Lake is using the Data Skipping and Z-Ordering algorithm for I/O pruning where it supports our read query pattern. Delta Lake正在使用数据跳过和Z排序算法进行I / O修剪,它支持我们的读取查询模式。
结论 (Conclusion)
Building our Engagement Activity Delta Lake was a fun process. We hope our journey may help those who are designing a data lake that supports batch/incremental read, want to support mutation of the data in data lake, want to scale up data lake with performance tuning, or want to support exact once write across tables with Delta Lake.
建立我们的参与活动Delta Lake是一个有趣的过程。 我们希望我们的旅程可以帮助那些正在设计支持批量/增量读取的数据湖,希望支持数据湖中数据的突变,希望通过性能调整来扩展数据湖,或者希望在表之间进行精确写入的人提供帮助。与三角洲湖。
翻译自: https://engineering.salesforce.com/engagement-activity-delta-lake-2e9b074a94af