数据结构堆栈 内存堆栈
We live in an era of uncertainty. It is uncertain how economy will go on the aftermath of COVID-19 (fast/slow recovery, where and which industry sectors will be more affected, etc.). It is uncertain how technical people will be working from now on (physical vs. remote sites). And, of course, it is still uncertain what exactly a Data Scientist (DS) is and should be (and should be not) doing in the industry.
我们生活在一个不确定的时代。 尚不确定COVID-19之后的经济将如何发展(快速/缓慢的复苏,哪里以及哪个行业受到更大的影响,等等)。 目前尚不确定技术人员在物理站点或远程站点上将如何工作。 而且,当然,仍然不确定数据科学家(DS)在行业中到底应该做什么,应该做什么(应该做什么)。
数据科学? 不是我最喜欢的命名法... (Data Science? Not my favorite nomenclature…)
The well-known academic researcher Peter Flach (10 years Editor-in-Chief of the Machine Learning journal) has recently published an article where he says that Data Science is not a very good nomenclature for the field. The main reason behind such statement is that “Data Science” is prone to misguided interpretations on assuming that Physicians, Biochemistricians or Civil Engineers as Data Scientists if they work intensively with Data (aka being Data Driven). Thus, Prof. Flach prefers the term “Science of Data”, defining it as follows: “ (…)subject that studies data in all its manifestations, together with methods and algorithms to manipulate, analyse, visualize and enrich data. It is methodologically close to computer science and statistics, combining theoretical, algorithmic and empirical work (…)”. And, it is important to stress this out: I fully agree with him.
著名的学术研究人员Peter Flach (《机器学习》杂志总编辑已10年)最近发表了一篇文章,他说数据科学并不是该领域的很好的术语。 这种说法背后的主要原因是,“数据科学”倾向于假设医师,生物化学家或土木工程师为数据科学家,如果他们与数据紧密合作(又称为数据驱动),则容易产生误解。 因此,Flach教授更喜欢“数据科学”一词,其定义如下:“ (…)主题研究数据的所有表现形式,以及操纵,分析,可视化和丰富数据的方法和算法。 它在方法上接近于计算机科学和统计学,结合了理论,算法和经验工作(…) ”。 而且,重要的是要强调这一点:我完全同意他的看法。
Nevertheless, there is a trend in the industry on pushing to have “full stack data scientists”. The number of articles out there that support this trend are numerous…but I leave you with an interesting shortlist for your reference:
但是,行业中有一种趋势,要求拥有“全栈数据科学家”。 支持这一趋势的文章数量众多……但我为您提供了一个有趣的候选清单供您参考:
https://thefullstackdatascientist.com/blog/what-is-a-full-stack-data-scientist/
https://thefullstackdatascientist.com/blog/what-is-a-full-stack-data-scientist/
https://www.laurencegellert.com/2012/08/what-is-a-full-stack-developer/
https://www.laurencegellert.com/2012/08/what-is-a-full-stack-developer/
https://blog.dominodatalab.com/become-full-stack-data-science-company/
https://blog.dominodatalab.com/become-full-stack-data-science-company/
https://multithreaded.stitchfix.com/blog/2019/03/11/FullStackDS-Generalists/
https://multithreaded.stitchfix.com/blog/2019/03/11/FullStackDS-Generalists/
“全栈数据科学家”只是AI宣传的另一个方面。 (“Full Stack data scientists” are just yet another facet of the AI hype.)
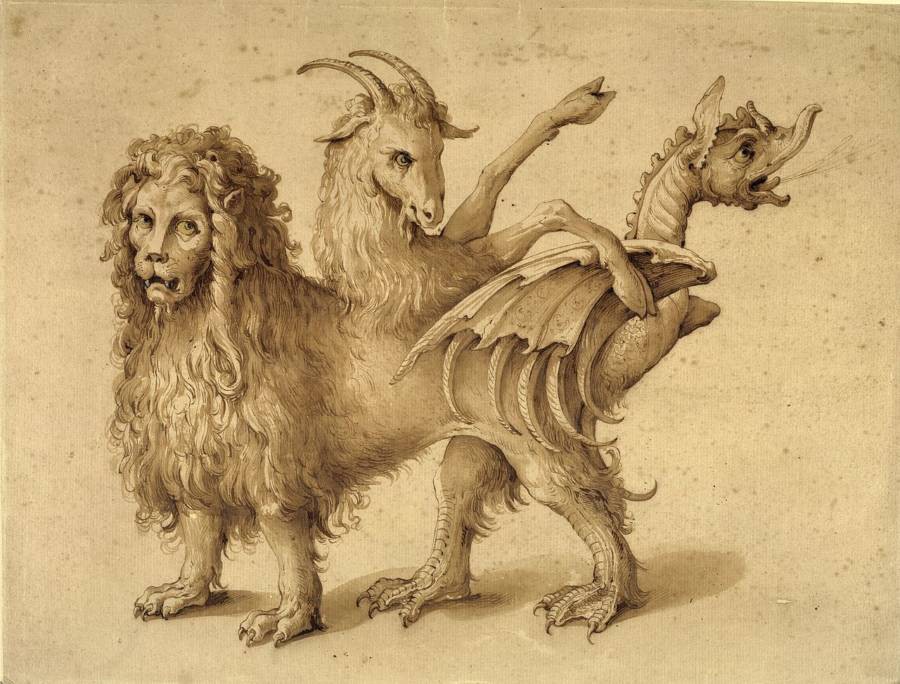
According to this trend, these mythological individuals should be capable of understanding the business problem, perform root cause analysis and derive hypothesis (as a generic Big3 strategy consultant would do), prepare all the data that they will need + the data pipelines needed to put something in production in the cloud, create model(s), validate model(s), deploy model(s), monitor the model(s) in production — from a DevOps perspective (is the service working/scaling properly?), from a business perspective (is it delivering the expected target KPIs?) from a scientist perspective (is it generalizing well? is there any concept drift?), from an engineer perspective (has the data input the expected format?) — and, of course, be able to present the expected/obtained results to an heterogeneous audience of stakeholders in a concise and yet understandable fashion. Finally — and this one is the most important skill — a data scientist must be able to fly! :)
根据这种趋势,这些神话人物应该能够理解业务问题,进行根本原因分析并得出假设(就像Big3战略顾问所做的那样),准备他们将需要的所有数据+放置数据所需的数据管道。从DevOps角度(服务是否在正常工作/扩展?),从DevOps的角度来看,云中生产中的某些事物,创建模型,验证模型,部署模型,监视生产中的模型。从科学家的角度(是否很好地概括了吗?有没有概念上的偏差?),从工程师的角度(是否输入了预期的格式?),从业务角度(是否提供了预期的目标KPI?)—当然, ,能够以简洁但易于理解的方式向不同利益相关方的受众群体展示预期/获得的结果。 最后-这是最重要的技能-数据科学家必须具备飞行能力! :)
Naturally, this generalist DS view is not shared by me — or, at least, not fully, as you will understand in later parts of this post — as these people tend to be very rare (and, if they do exist, they should not be staff/team member level data scientists but leaders instead). This new full-stack DS hype goes in the direction of raising the expectations of what AI Experts/Data Scientists (terms which I here use interchangeably as a writer convenience but are not quite the same thing) can and should deliver to unrealistic levels. In a short sentence, “full stack data scientists” are just yet another facet of the AI hype. And, as other sectors of our society have been showing to us, history tends to repeat itself — in this case, the risk of being facing yet another AI Winter soon.
自然,我不会分享这种多才多艺的DS视图,或者至少不会完全分享(正如您将在本文后面的部分中所理解的那样),因为这些人往往非常稀有(并且,如果确实存在,则不应成为员工/团队成员级别的数据科学家,但要成为领导者)。 这种新的全栈DS炒作旨在提高人们对AI专家/数据科学家(我在这里可以互换使用,以方便编写者但又不完全相同)的期望,并且应该达到不切实际的水平。 简而言之,“全栈数据科学家”只是AI宣传的另一个方面。 而且,正如我们社会的其他部门向我们展示的那样,历史往往会重演-在这种情况下,很快就要面临另一个AI Winter的风险。
五月天,五月天…我们需要数据科学家来做数据科学! (Mayday, mayday…we need Data Scientists to do Data Science!)
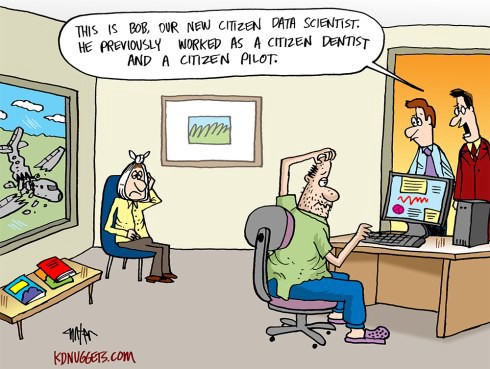
Data Scientists must be good on doing Data Science. And Data Science problems are already difficult enough to solve per se…imagine if you a) are not a specialist and b) you still need to care for all those stuff around production data science all by yourself. It looks pretty tough…doesn’t it?
数据科学家必须擅长进行数据科学。 与数据科学问题都已经足够困难的解决本身......想象一下,如果你一)不是专家和b)你仍然需要照顾周边的生产数据的科学所有的东西全部由自己。 看起来很艰难……不是吗?
“If I had an hour to solve a problem, I’d spend 55 minutes thinking about the problem and 5 minutes thinking about solutions.” Albert Einstein
“如果我有一个小时来解决问题,那么我将花55分钟思考问题,花5分钟思考解决方案。” 艾尔伯特爱因斯坦
Usually, I do not devote time to blog writing. When I see other Data Science Leaders doing one LinkedIn post per day, multiple blog posts per month and even several books every year…I wonder if they either do not sleep or if, in alternative, they simply are not working on Data Science at all(!!!). Whenever I feel to have something to contribute to the DS community, I prefer to do it on the technical side of things, by participating (as an author, committee member or track chair) on the top peer-reviewed venues in the area. However, this hype issue affects the industry so much that I believe that a contribution like this will help other colleagues (both at staff level and at leadership one) on organizing better their career and/or learning paths, workload, teamwork and, ultimately, throughput and business impact.
通常,我不花时间写博客。 当我看到其他数据科学负责人每天在LinkedIn上发表一篇文章,每月发表多篇博客文章,甚至每年发表几本书时,我想知道他们是否要么不睡觉,要么根本不从事数据科学工作? (!!!)。 每当我觉得对DS社区有贡献时,我都喜欢在技术方面,通过参加(作为作者,委员会成员或主席)在该地区经过同行评审的顶级场所来做。 但是,这一炒作问题对整个行业产生了巨大影响,我相信这样的贡献将有助于其他同事(无论是在员工级别还是在领导级别)更好地组织其职业和/或学习路径,工作量,团队合作,最终,吞吐量和业务影响。
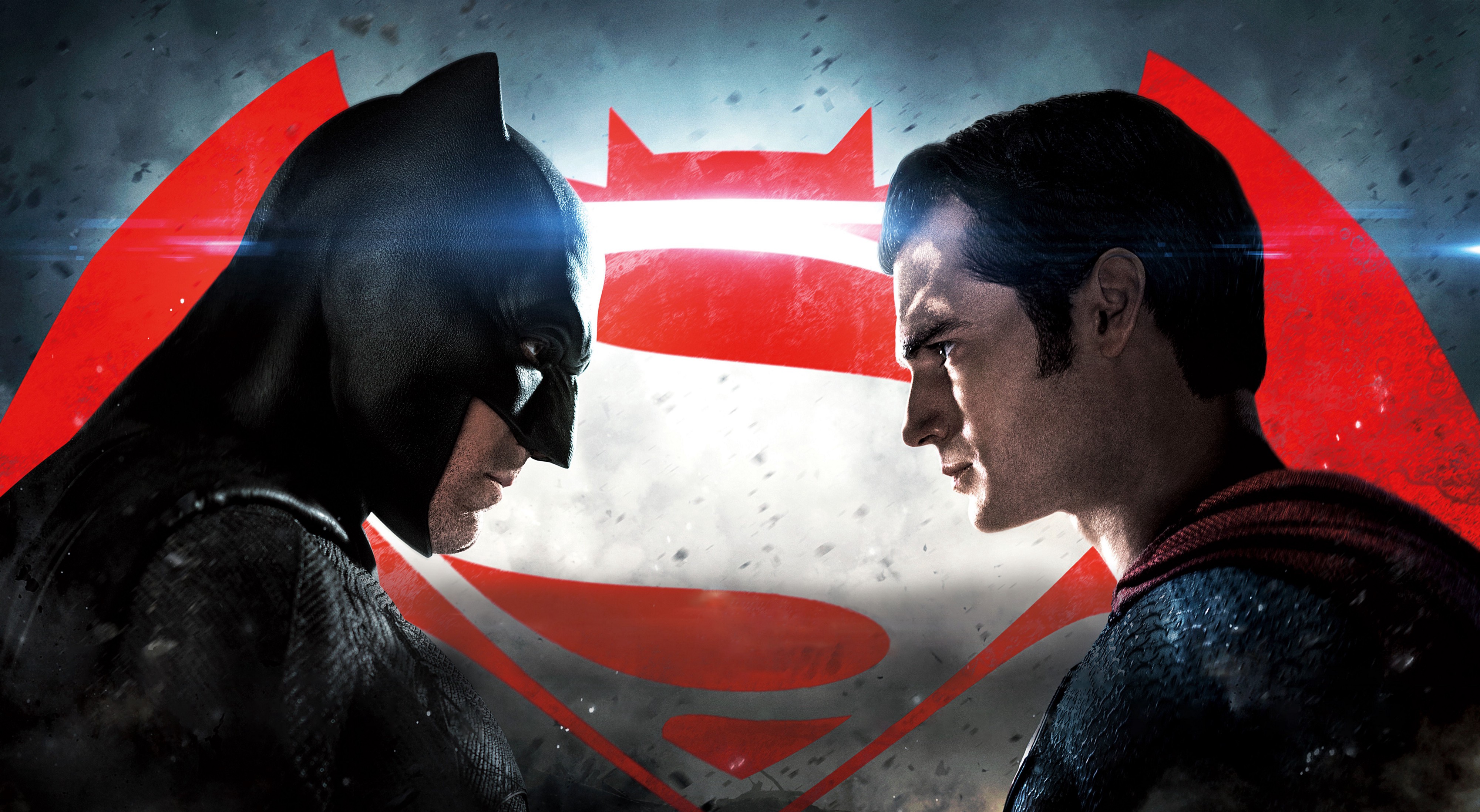
The purpose of this post is to explain why yet another fancy term to define the role of a data scientist is not such a great idea. Moreover, I also point out where are the pitfalls of such hype and which are the real problems that need to be tackled in order to push the widespread industrial adoption of data science. The ultimate goal (of all of us, I believe) is to raise the bar of ML-driven success business cases (predictive and prescriptive analytics) to a business-as-usual standard. At least, that is my main motivation.
这篇文章的目的是解释为什么定义数据科学家角色的另一个幻想术语不是一个好主意。 而且,我还指出了这种炒作的陷阱在哪里,以及哪些真正的问题需要解决,以推动数据科学在工业上的广泛采用。 (我相信,我们所有人的最终目标是将ML驱动的成功业务案例(预测性和规范性分析)的标准提高到通常水平。 至少,这是我的主要动机。
Besides the present post entitled Beginnings, this post has two more parts. In part two, The Fall, I will be deconstructing the four key arguments (I-IV) of those who argue that the DS generalist is the way to go (vs. the specialists). Finally, within part III, The Rise, I will present three key ideas to address the real issues behind those problems, including a definition of what a modern data scientist should be doing. And yes, albeit I prefer Batman to Super-Man, the blog post’s title have little to do with the Dark Knight trilogy movies.
除了当前标题为Beginnings的帖子外,该帖子还有两个部分。 在第二部分“ The Fall”中,我将解构那些认为DS通才是前进之路(相对于专家)的四个关键论点(I-IV)。 最后,在第三部分“崛起”中,我将提出三个关键思想来解决这些问题背后的实际问题,包括定义现代数据科学家应该做什么。 是的,尽管我比蝙蝠侠更喜欢蝙蝠侠,但博客文章的标题与《黑暗骑士》三部曲电影无关。
Curious for more? Wait for the next two parts…in a blog near you.
想知道更多吗? 等待下两个部分……在您附近的博客中。
P.S.: I would like to personally thank to Fernando Costa and Sven Thies the time they devoted on reviewing these posts. Kudos to the two of them.
PS:我要亲自感谢Fernando Costa和Sven Thies花费的时间来审查这些帖子。 对他们两个表示敬意。
翻译自: https://medium.com/@luis.moreira.matias/zero-stack-data-scientist-part-i-beginnings-1691afa2b510
数据结构堆栈 内存堆栈