数据结构堆栈 内存堆栈
In Hollywood, it is known that the sequels are rarely better than the original movie/parts. Batman: The Dark Knight Trilogy is a notorious exception. I believe that this post is yet another.
在好莱坞,众所周知,续集很少比原始电影/部分更好。 蝙蝠侠:黑暗骑士三部曲是一个臭名昭著的例外。 我相信这是另一篇文章。
After explaining what many claim to be a “Full-Stack Data Scientist” in Part I of this post, I am going to discuss the main claims that sustain “the generalist” as a way to go for a Data Scientists (DS) in industry. In this post, I present, discuss and deconstruct the four key arguments that “the generalist” fans use to support their point of view.
在解释了这篇文章的第一部分中许多人声称是“全栈数据科学家”之后,我将讨论维持“通才”的主要主张,以此作为行业中数据科学家(DS)的一种方法。 在这篇文章中,我介绍,讨论和解构“通才”支持者用来支持他们观点的四个关键论点。
“全栈数据科学家”的四大错误Struts (The four false pillars of the “full-stack data scientist”)
(I)根本原因分析是端到端的-因此,DS也应该如此!((I) Root Cause Analysis is end-to-end — so, a DS also should be!)
Root Cause Analysis is something strategy consultants have been doing for decades. One of the main pro-generalist argument is that the causes for the low performance of Machine Learning models lies outside the modelling stage. And, by experience, I can classify that statement as truthful. Now, two questions arise: (1) should RCA be done by data scientists? And, if yes, (2) would it help them to be more effective on finding the root causes if they own the entire process? And, so far, I still follow with them: yes and yes for the two questions. But quickly, another question arises: how would it be if data scientists owned this project end-to-end? Well, the most likely answer would involve delays on project completion, lower user acceptance, troubles on scalability…i.e., more root causes to find and a bigger problem. Why? Because they are not experts (e.g.: in UX design, devops or data engineering)! When you try to do something that is difficult and you are not an expert on it, you will take more time to do it and you will be more likely to do a mistake…or several. Ab uno disce omnes.
根本原因分析是战略顾问数十年来一直在做的事情。 普遍主义者的主要论据之一是,机器学习模型性能低下的原因不在建模阶段。 而且,根据经验,我可以将该陈述归类为“真实” 。 现在,出现两个问题:(1)RCA是否应由数据科学家完成? 并且,如果是,(2)如果他们拥有整个流程,是否可以帮助他们更有效地找到根本原因? 而且,到目前为止,我仍然跟随他们:对两个问题,是和是。 但是很快出现了另一个问题:如果数据科学家端到端拥有该项目,情况会如何? 好吧,最可能的答案将涉及项目完成的延迟,用户接受度的降低,可伸缩性的问题……即,更多的根本原因被发现和更大的问题。 为什么? 因为他们不是专家(例如:UX设计,devop或数据工程专家)! 当您尝试做一些困难的事情而又不是专家时,您将花费更多的时间来做,而且您更有可能犯错误,甚至是犯几个错误。 不可否认的全能。
What is the problem that usually happens when specialized DS try to run RCA? They stumble onto other team’s walls. Why? Three classics are: lack of ownership ,aka Social Loafing, lack of communication and lack of data driven awareness. If other teams are not available to step up or their technical/communication mistakes and/or are not aware of what the data science modules do (input/output) and of the possible consequences that their (bad) work may have there…your organization has a problem of Culture, Values and, ultimately, Leadership. And, if that happens, you can have whatever type of Data Scientists you want…as you will always be closer to failure than to… well, pretty much anything else.
专用DS尝试运行RCA时通常会发生什么问题? 他们跌倒在另一支球队的墙上。 为什么? 三个经典是:缺乏所有权,又名社交闲逛,缺乏沟通和缺乏数据驱动的意识。 如果其他团队无法加强工作或他们的技术/通信错误,并且/或者不知道数据科学模块的工作(输入/输出)以及他们的(不良)工作可能带来的后果……您的组织有文化,价值观和最终领导力的问题。 而且,如果发生这种情况,您可以拥有所需的任何类型的数据科学家……因为您总是比失败更接近失败……嗯,几乎还有其他任何事情。
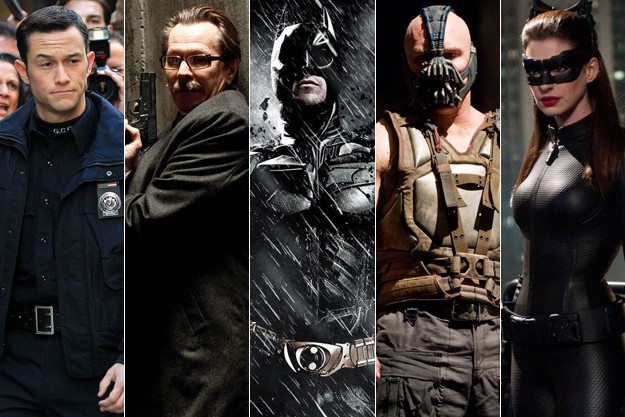
(II)多个角色带来沟通负担 ((II) Multiple Roles bring Communication Overhead)
I have to admit this is the one that makes less sense for me. If you work to