边缘云与中心云
Typically, Deep Learning — the subspecies of AI application that have gained the spotlight in the past few years thanks to their success in deploying real-world working AI at scale — is offered as Cloud-supported Software-As-A-Service (SaaS) platforms.
通常,深度学习-过去几年中由于在大规模部署实际工作的AI方面的成功而在AI应用程序中引起关注的子类-作为云支持的软件即服务(SaaS)提供平台。
This does not work for many manufacturers, who tend to avoid the security and latency issues from Cloud-reliant software to manage the day-to-day complex workflows.
对于许多制造商而言,这是行不通的,他们倾向于避免使用依赖云的软件来管理日常复杂工作流的安全性和延迟问题。
Fortunately, technology evolving, and in a 2020 that has otherwise been challenging for the sector, a paradigm-changing evolution of Deep Learning and AI is coming to benefit the need for manufacturing.
幸运的是,技术在不断发展,并且在2020年对于该行业而言是充满挑战的,深度学习和AI的范式变革将使制造需求受益。
But first, let’s understand what we mean by Deep Learning and how hardware — Cloud vs Edge — plays such an important role.
但是首先,让我们了解深度学习的含义,以及硬件(云与边缘)如何发挥如此重要的作用。
引擎盖下:AI (Under the hood: AI)
AI’s generic name hides a variety of approaches that span from huge Artificial Intelligence models crunching data on a distributed cloud infrastructure, to tiny Edge-friendly AI that analyzes and mines data on small processors.
AI的通用名称隐藏了多种方法,从在分布式云基础设施上处理数据的大型人工智能模型到在小型处理器上分析和挖掘数据的小型Edge-friendly AI。
Let’s simplify the landscape and split AI into two main classes, the ‘heavy’ and ‘light’ types. Heavy AI requires large compute substrates to run, while the Light AI can do what Heavy AI is capable of on a small compute footprint.
让我们简化环境并将AI分为两个主要类别,“重”和“轻”类型。 重型AI需要运行大型计算基板,而轻型AI可以在较小的计算空间上完成重型AI的功能。
The introduction of commodity processors such as GPUs, and later their portability, has made it technically and economically viable to bring AI/Deep Learning/DNN/Neural Networks algorithms to the Edge.
诸如GPU之类的商用处理器的引入以及后来的可移植性,使得将AI /深度学习/ DNN /神经网络算法引入Edge在技术上和经济上都是可行的。
Edge AI computing is, intuitively, a great idea: mother nature has figured this out over eons of evolutionary time by moving some biological compute to the periphery, from our sensors (e.g., eyes, ears, skins) to our may organs and muscles, where much of the world ‘data’ is produced as organisms explore their world.
从直觉上讲,边缘AI计算是一个好主意:大自然在进化时代就已经解决了这一问题,方法是将一些生物计算移动到外围,从我们的传感器(例如,眼睛,耳朵,皮肤)到我们可能的器官和肌肉,随着生物探索世界,世界上许多“数据”都在这里产生。
Similarly, it makes tons of sense for manufacturers to exploit this feature. Take, for instance, quality control cameras in industrial machines, where a typical machine can be processing at dozens of frames per seconds, hundreds of products per hour. It would be extremely wasteful and inefficient for these cameras to ship all the captured frames to a centralized Cloud for AI processing. Rather, a more intelligent strategy would be to process them at the Edge and occasionally send the pertinent, highly important frames (e.g., with a possible product defect) to a centralized location or to a human analyst.
同样,制造商利用此功能很有意义。 以工业机器中的质量控制摄像机为例,在一台典型的机器中,每秒可以处理数十个帧,每小时可以处理数百种产品。 这些相机将所有捕获的帧运送到集中的云以进行AI处理将非常浪费并且效率低下。 相反,更明智的策略是在Edge上进行处理,并偶尔将相关的,非常重要的框架(例如,可能存在产品缺陷)发送到集中位置或人工分析人员。
Issues such as bandwidth, latency, and cost dictate the need for Edge processing.
带宽,等待时间和成本等问题决定了对Edge处理的需求。
There is an important caveat though: running AI on small compute Edge — what in jargon is called inference, or ‘predictions’ (e.g., I see a normal product vs. a defective one) is different from learning at the Edge. Namely, using the acquired information to change, improve, correct and refine the Edge AI is not only difficult, but extremely important for manufacturers to be able to customize their AI quickly to achieve flexibility
但是,有一个重要的警告:在小型计算边缘上运行AI –用术语来说就是推理或“预测”(例如,我看到一个正常的产品相对于一个有缺陷的产品)与在Edge上学习有所不同。 即,使用获取的信息来更改,改进,校正和完善Edge AI不仅困难,而且对于制造商能够快速自定义其AI以实现灵活性非常重要。
边缘(与云)学习:现实 (Edge (vs Cloud) Learning: a reality)

I first realized the huge difference working with NASA back in 2010 when me and my colleagues implemented a small brain emulation to control a Mars-rover like device with AI that needed to be capable to run and learn at the Edge.
早在2010年,我和我的同事就实现了一种小型大脑仿真功能,并通过AI来控制类似Mars-rover的设备,而这种设备需要能够在Edge上运行和学习,我首先意识到与NASA的巨大区别。
For NASA, it was important that a robot would be capable of learning ‘new things’ completely independently of any compute power available on Earth. Data bottleneck, latency, and a plethora of other issues made it vital to explore different breeds of AI than the one developed since that time. Algorithms that were capable to digest and learn — namely, adapt the behavior of AI the available data — without requiring huge amount of compute power, data, and time. Traditional Deep Neural Network (DNN) models were not capable to do that.
对于NASA而言,重要的是,机器人能够完全独立于地球上任何可用的计算能力来学习“新事物”。 数据瓶颈,延迟和许多其他问题使得探索与自那时以来开发的AI品种不同的AI至关重要。 能够消化和学习(即使AI适应可用数据的行为)的算法,而无需大量的计算能力,数据和时间。 传统的深度神经网络(DNN)模型无法做到这一点。
We built such and AI, which we dubbed Lifelong Deep Neural Network (Lifelong-DNN) for its ability to learn throughout its lifetime (vs traditional DNN that can only learn once, before deployment).
我们构建了这样的AI,我们将其称为终身深度神经网络( Lifelong-DNN ),因为它具有终身学习的能力(与传统的DNN相比,在部署之前只能学习一次)。
Little we know that this AI would turn useful more on Earth than on Mars.
我们几乎不知道,这种AI在地球上比在火星上更有用。
边缘学习对工业制造商的影响 (The power of Edge Learning for Industrial Manufacturers)
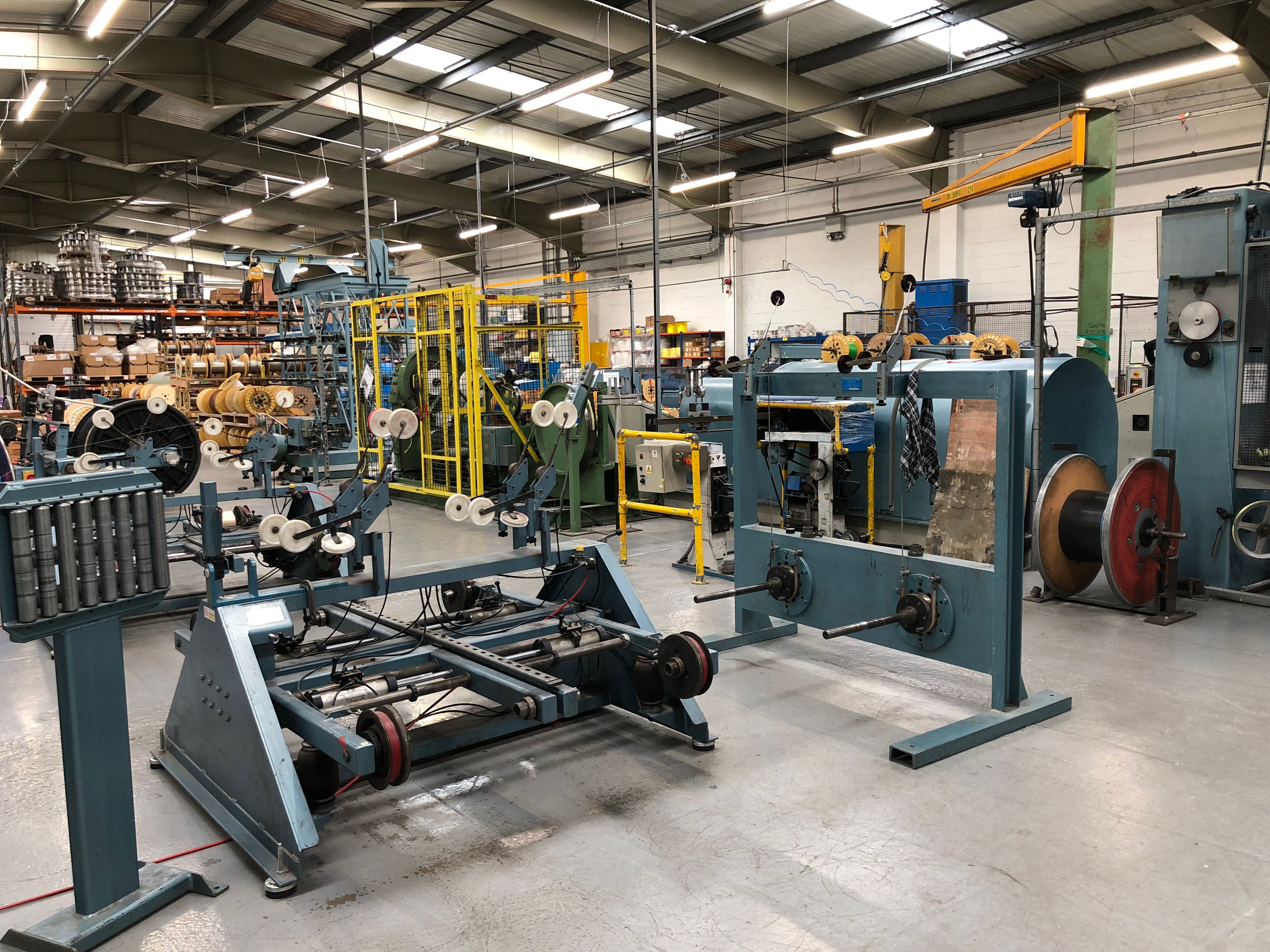
Edge learning solves one of the burning issues of today’s AI implementation: its inflexibility and lack of adaptability. AI algorithms can be trained on huge amount of data, when available, and be fairly robust if all data is captured for their training beforehand. Unfortunately, this is not how manufacturing works, because data — e.g., bad product — is not usually available until the few bad products come out, unpredictably, from a line. AI needs to be able to quickly exploit this disparate, rare data to adapt, but traditional DNNs can’t work in these realistic conditions.
边缘学习解决了当今AI实施中的一个紧迫问题:它的灵活性和缺乏适应性。 当可用时,可以对大量数据训练AI算法,并且如果事先捕获所有数据进行训练,则AI算法将相当健壮。 不幸的是,这不是制造业的工作方式,因为通常只有很少的不良产品从生产线中出来之前,通常无法获得数据(例如不良产品)。 人工智能需要能够快速利用这些分散的稀有数据来进行适应,但是传统的DNN无法在这些现实条件下工作。
Novel approaches such as Lifelong-DNN — a Deep Learning paradigm that enables learning at the compute Edge, e.g., a CPU — enable AI-powered cameras not only to understand the data coming to them, but also adapt and learn. For example, in the industrial machine described above, Edge learning would enable its dozens cameras to learn new products types, and defects, in a real-world scenario where new items are introduced all the time, and new, previously unseen defects show up on the production floor.
诸如Lifelong-DNN之类的新颖方法-一种深度学习范例,可以在诸如CPU之类的计算边缘进行学习-使AI供电的相机不仅能够理解传给他们的数据,而且还能适应和学习。 例如,在上述工业机器中,边缘学习将使其数十个相机能够学习新产品的类型和缺陷,而在这种情况下,始终会引入新的物品,并在屏幕上显示以前看不见的新缺陷。生产车间。
No AI could exist that can be pre-trained on newly created products. Data simply does not exist: AI needs to be trained on the spot!
不可能存在可以在新创建的产品上进行预训练的AI。 数据根本不存在:需要对AI进行现场培训!
With Edge Learning, AI can learn to recognize new defects, without having to ‘reprogram’ the AI from scratch, directly at the Edge where it is needed.
借助Edge Learning,AI可以学会识别新缺陷,而无需直接在需要的Edge上从头对AI进行“重新编程”。
AI that learns at the Edge is a paradigm-shifting technology that will finally empower AI to truly serve its purpose: shifting intelligence to the compute Edge where it is needed, at speeds, latency, and costs that make it affordable for every device.
在Edge上学习的AI是一种范式转换技术,它将最终使AI真正实现其目标:以速度,延迟和成本将智能转移到需要它的计算Edge,从而使每台设备都能负担得起。
This will enable manufacturers to build a fundamental brick of their Industry 4.0 strategy inexpensively, quickly, and directly where it counts: at the Edge.
这将使制造商能够在Edge上廉价,快速,直接地建立起自己的Industry 4.0战略的基础。
翻译自: https://towardsdatascience.com/cloud-vs-edge-an-industrial-manufacturing-dilemma-44472f01cf45
边缘云与中心云