超级符号就是超级创意
重点(Top highlight)
让食物成为您的良药(Let food be thy medicine)
This post was co-authored with Kirill Veselkov and Gabriella Sbordone and is based on the TEDx Lugano 2019 talk and the paper published in Nature journal Scientific Reports.
这篇文章是与Kirill Veselkov和Gabriella Sbordone共同撰写的,其依据是TEDx Lugano 2019演讲以及《自然》杂志《科学报告》上发表的论文。
We now live longer than ever. Yet, we are not necessarily living healthier anymore: with a rapidly aging population, people are experiencing a continuous growth of chronic diseases such as cancer, metabolic, neurological, and heart disorders. This drives healthcare costs through the roof and puts a significant strain on the public health systems [1].
我们现在活得比以往更长。 但是,我们并不一定生活得更健康:随着人口的快速老龄化,人们正经历着诸如癌症,新陈代谢,神经系统疾病和心脏病等慢性疾病的持续增长。 这推动了医疗费用的上涨,给公共卫生系统带来了巨大压力[1]。
A large part of the problem resides in poor dietary choices. Unhealthy diets kill more than cigarettes and are responsible for 1 out of 5 deaths globally — in 2018, this amounted to nearly 11 million lives. Besides the obvious culprit — unhealthy highly processed food — a less obvious killer is the low intake of healthy foods such as whole grains, vegetables, fruits, nuts, seeds, and legumes [2].
问题的很大一部分在于不良的饮食选择。 不健康的饮食造成的死亡超过香烟,是造成全球五分之一死亡的原因-2018年,这将近1100万人的生命。 除了明显的罪魁祸首(不健康的高度加工食品)以外,另一个不太明显的杀手是低摄入健康食品,例如全谷物,蔬菜,水果,坚果,种子和豆类食品[2]。
Take cancer for example: rightfully considered the plight of the modern era, it will affect every second reader of this post at one point in their lifetime. Although the perspective seems gloomy, the good news is that nearly 40% of all oncological diseases could be prevented through dietary and lifestyle changes alone [3] — a finding that encourages us to look more carefully at what we eat, as diet is perhaps the single most important modifiable risk factor for cancer.
以癌症为例:理所当然地考虑到现代的困境,它将在一生中的某个时刻影响到第二位读者。 尽管观点似乎令人沮丧,但好消息是仅通过饮食和生活方式的改变就可以预防近40%的肿瘤疾病[3] –这一发现鼓励我们更加仔细地研究饮食,因为饮食可能是一个最重要的可改变的癌症危险因素。
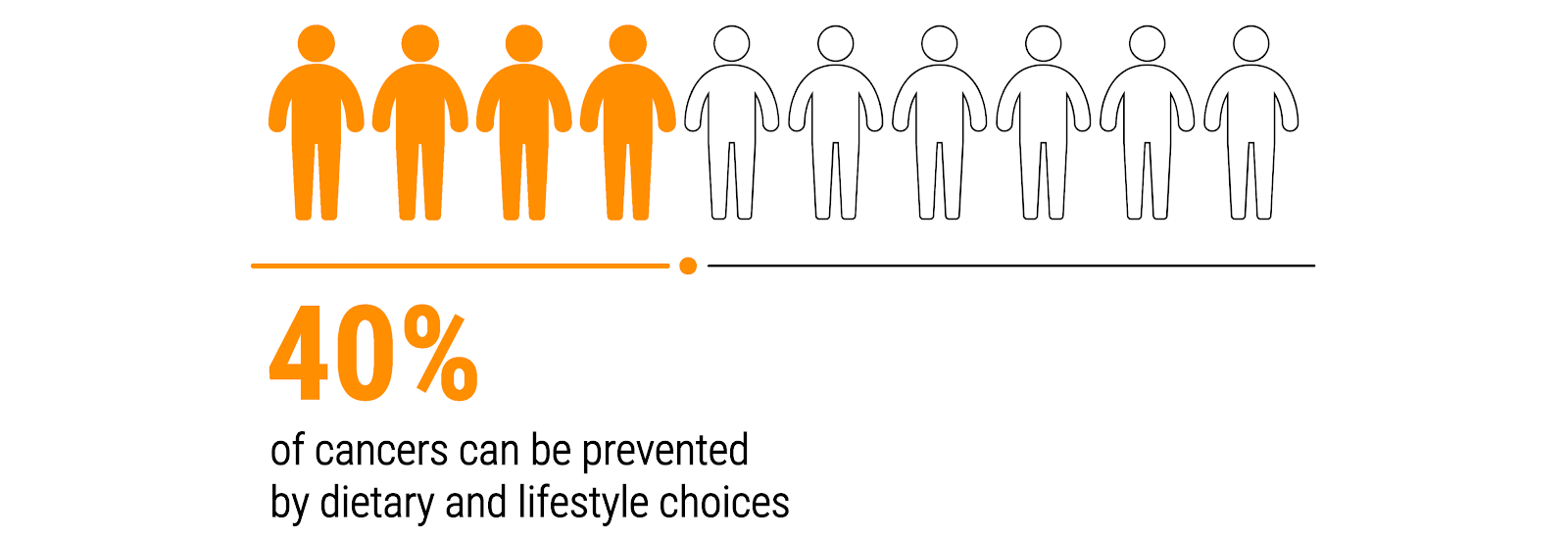
暗物质 (The dark matter)
In the past decades, nutrition science has made excellent progress in analysing the six major nutrition categories influencing human health and disease: proteins, carbohydrates, fats, minerals, vitamins, and water. National nutritional databases track about 150 components from these categories and they appear on every food package.
在过去的几十年中,营养科学在分析影响人类健康和疾病的六个主要营养类别中取得了卓越的进步:蛋白质,碳水化合物,脂肪,矿物质,维生素和水。 国家营养数据库跟踪这些类别中的大约150种成分,它们出现在每个食品包装中。
Yet, there is growing evidence that thousands of other molecules from a broad variety of chemical classes — such as polyphenols, flavonoids, terpenoids, and indoles that are abundant in plants and are often naturally responsible for their colour, taste, and odour — might help prevent and fight diseases [4]. Most of these compounds still remain largely unexplored by experts, not tracked by regulators, and unknown to the public at large, thus truly deserving the name “dark matter of nutrition” [5].
但是,越来越多的证据表明,植物中大量的多种化学类别的数以千计的其他分子(例如多酚,类黄酮,萜类和吲哚)通常有助于其颜色,味道和气味,它们可能会有所帮助预防和抵抗疾病[4]。 这些化合物中的大多数仍然在很大程度上未被专家探索,未被监管者追踪,并且对于整个公众都不为人所知,因此,真正应得的名称是“营养暗物质” [5]。
With every bite of food, we put in our mouth hundreds of such biologically active compounds. These molecules interact with each other the moment we swallow them, and as the food gets digested and metabolised, also react with other biomolecules in our body and with trillions of bacteria in our guts.
每一口食物,我们都会将数百种此类生物活性化合物放入口中。 这些分子在吞入它们的那一刻就会相互影响,随着食物的消化和代谢,它们还会与我们体内的其他生物分子以及肠道中的数万亿细菌发生React。
Many of the compounds found in plant-based foods belong to the same chemical classes as drugs. It is not surprising therefore that almost half of small molecules approved for anti-cancer therapies are derived from natural products. These drugs are generally more tolerated and less toxic to healthy cells [6].
植物性食品中发现的许多化合物与药物属于同一化学类别。 因此,毫不奇怪,几乎有一半的被批准用于抗癌治疗的小分子都来自天然产物。 这些药物通常对健康细胞具有更高的耐受性和毒性[6]。
药品和食品 (Drugs and foods)
Traditional drug molecules are designed to bind to biomolecular targets associated with particular disease processes, the most important of which involve proteins [7]. Classical drug therapy follows the “one disease–one drug–one target” paradigm, trying to identify one “druggable” protein associated with the disease that can be targeted by the drug. In reality, drugs are rarely so selective [8], and the intricate network (or “graph”) of interactions between proteins produces a “network effect” that can interfere with multiple biological processes — like one falling domino knocking off an entire row.
传统的药物分子被设计为与特定疾病过程相关的生物分子靶标结合,其中最重要的涉及蛋白质[7]。 古典药物疗法遵循“一种疾病-一种药物-一个靶点”的范例,试图识别一种与该药物相关的疾病相关的“可消耗”蛋白质。 实际上,药物很少具有选择性[8],蛋白质之间复杂的相互作用网络(或“图形”)产生“网络效应”,可以干扰多种生物过程,例如一个下降的多米诺骨牌击倒整行。
Protein-protein interactions (PPIs) [9] are considered as the next generation of therapeutic targets, and most pharmaceutical industries have now extended their drug discovery programs to PPIs [10]. And in order to leverage the big amounts of molecular interaction data produced by modern high-throughput technologies, machine learning (ML) is becoming increasingly prominent. However, unlike images and audio signals where ML has achieved breakthrough results in the past decade, network-structured data require a different type of methods called “graph ML”.
蛋白质-蛋白质相互作用(PPI)[9]被认为是下一代的治疗靶标,现在大多数制药行业已将其药物发现计划扩展到PPI [10]。 为了利用现代高通量技术产生的大量分子相互作用数据,机器学习(ML)变得日益重要。 但是,与图像和音频信号在过去十年中ML取得了突破性进展不同,网络结构化数据需要使用另一种类型的方法,称为“图ML”。
Graph ML, also known as “graph representation learning” or “geometric deep learning” is a recent hot topic in machine learning, which I have covered extensively in my Toward Data Science blog. Typical graph ML architectures, called graph neural networks implement some form of message passing on the graph allowing different nodes to exchange information. In the simplest formulation, message passing takes the form of linear diffusion or “random walk” on the graph [11].
图ML(也称为“图形表示学习”或“几何深度学习”)是机器学习中的一个近期热门话题,我已在Toward Data Science博客中进行了广泛讨论。 典型的图ML体系结构(称为图神经网络)在图上实现某种形式的消息传递,从而允许不同的节点交换信息。 在最简单的表述中,消息传递在图表上采取线性扩散或“随机游动”的形式[11]。
In a paper published last year in Nature journal Scientific Reports, we applied graph ML to hunt for anti-cancer molecules in food using protein-protein and drug-protein interaction graphs [12]. The drug-to protein interactions were represented as signals on the PPI graph, and a learnable diffusion process was applied to model the network-wide effect of a drug.
在去年发表在《自然》杂志《科学报告》上的一篇论文中,我们利用蛋白质-蛋白质和药物-蛋白质相互作用图将图ML应用于食品中的抗癌分子[12]。 药物与蛋白质的相互作用表示为PPI图上的信号,并且采用了可学习的扩散过程来模拟药物在网络范围内的作用。
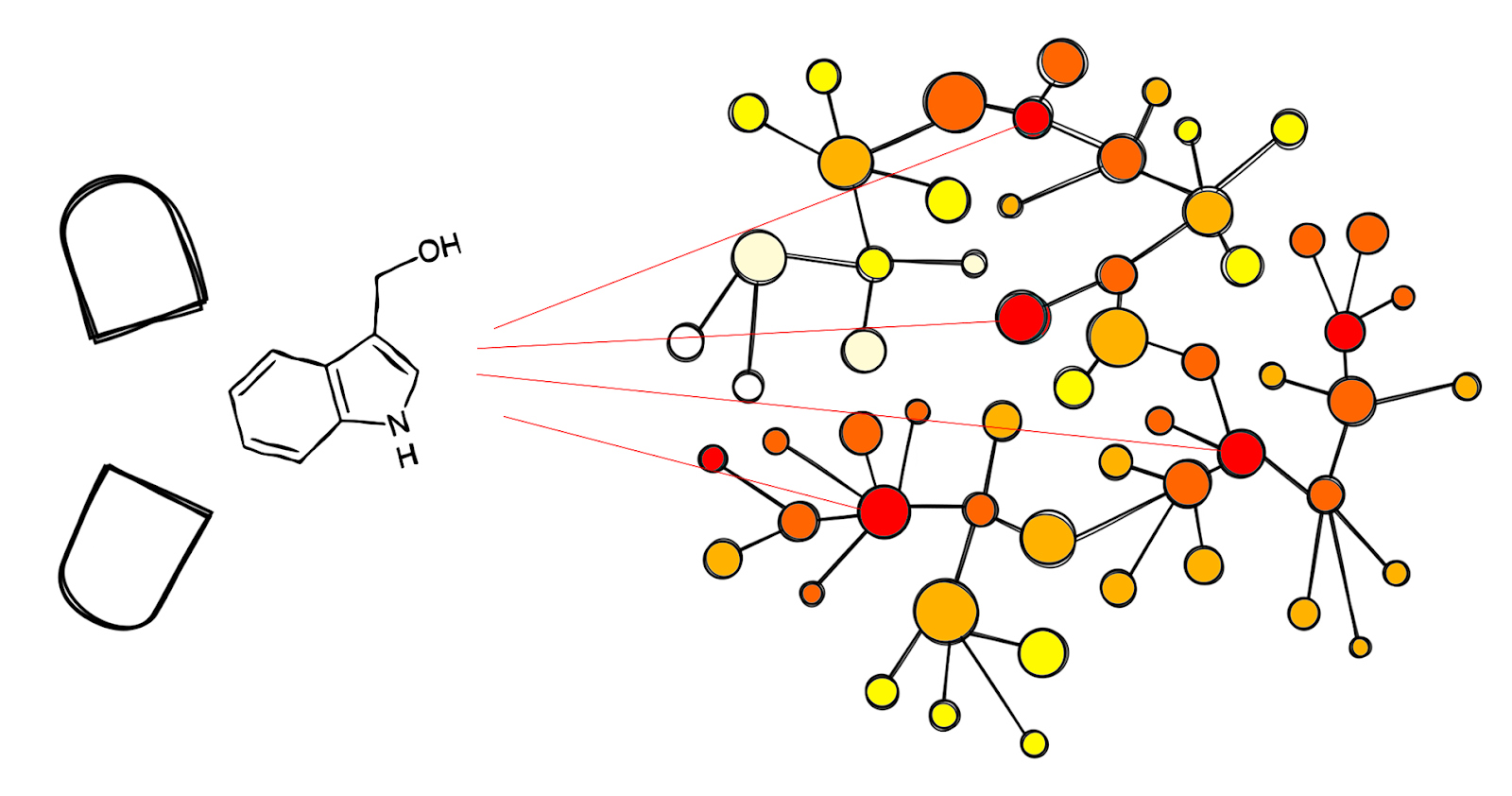
We used a training set of nearly 2000 clinically-approved drugs with about 10% labeled as anti-cancer in order to train a classifier that predicts the anti-cancer drug-likeness of new molecules from the way they interact with the PPI graph. We then fed the trained classifier with about 8000 food-based molecules for which protein interactions are known. Our model identified over a hundred anti-cancer drug-like candidates, which we called “cancer-beating molecules”. The main advantage of using an ML approach is that such molecules can be discovered automatically exploiting massive openly available datasets.
我们使用了将近2000种临床批准的药物训练集,其中约10%标记为抗癌药物,目的是训练一个分类器,该分类器根据新分子与PPI图的相互作用方式预测新分子的抗癌药物似然性。 然后,我们将经过训练的分类器喂给大约8000种基于食物的分子,这些分子之间的蛋白质相互作用是已知的。 我们的模型确定了一百多种抗癌药物样候选物,我们称之为“抗癌分子”。 使用ML方法的主要优点是可以利用大量公开可用的数据集自动发现此类分子。
We then resorted again to machine learning, using natural language processing (NLP) techniques to mine the trove of medical literature for experimental evidence of the anti-cancer effects of the identified molecules [13]. We also had to rule out compounds with excessive toxicity. This is the first validation step relying on the reported in vitro and in vivo experiments.
然后,我们再次诉诸于机器学习,使用自然语言处理(NLP)技术来挖掘医学文献资料库,以寻找所鉴定分子的抗癌作用的实验证据[13]。 我们还必须排除具有过度毒性的化合物。 这是依靠已报道的体外和体内实验的第一步验证步骤。
好东西 (The good stuff)
A key limitation of existing literature on food-based compounds is its focus on specific compounds, such as antioxidants, in isolation. You have certainly seen foods hailed as being rich in antioxidants and often marketed under the label of “superfoods”. Yet, while the regular consumption of such foods can reduce the risk of cancer formation (“oncogenesis”), the antiproliferative agents contained therein do not appear to consistently confer the same level of benefit when acting individually [14].
现有的有关食品基化合物的文献的一个关键限制是其对孤立的特定化合物(例如抗氧化剂)的关注。 您肯定已经看到食品富含抗氧化剂,并且通常以“超级食品”的标签出售。 然而,尽管定期食用此类食品可以降低罹患癌症(“肿瘤发生”)的风险,但是其中所含的抗增殖剂似乎在单独发挥作用时并未始终如一地带来相同的益处[14]。
This phenomenon is similar to the concurrent use of multiple drug substances in medical practice (technically known as “polypharmacy”), which can often lead to both undesired side effects as well as synergistic action more powerful than from each drug on its own [15]. The anti-cancer effect of some foods is thus the result of a combination of biologically active substances and is determined by both their antagonistic and synergistic actions and the way in which these simultaneously act on different oncogenic biological mechanisms.
这种现象类似于在医学实践中同时使用多种原料药(技术上称为“多药房”),这通常会导致不良副作用以及比每种药物单独使用更有效的协同作用[15] 。 因此,某些食物的抗癌作用是生物活性物质结合的结果,并取决于它们的拮抗作用和协同作用以及这些物质同时作用于不同致癌生物学机制的方式。
Tea and citrus fruits are examples of foods fulfilling both of these conditions: first, they contain multiple anti-cancer drug-like compounds identified by our ML model and confirmed from medical literature, and second, these compounds exert complementary anti-cancer effects [16].
茶和柑橘类水果是满足这两个条件的食物的示例:首先,它们包含多种由我们的ML模型鉴定并已得到医学文献证实的抗癌药物样化合物,其次,这些化合物发挥互补的抗癌作用[16 ]。
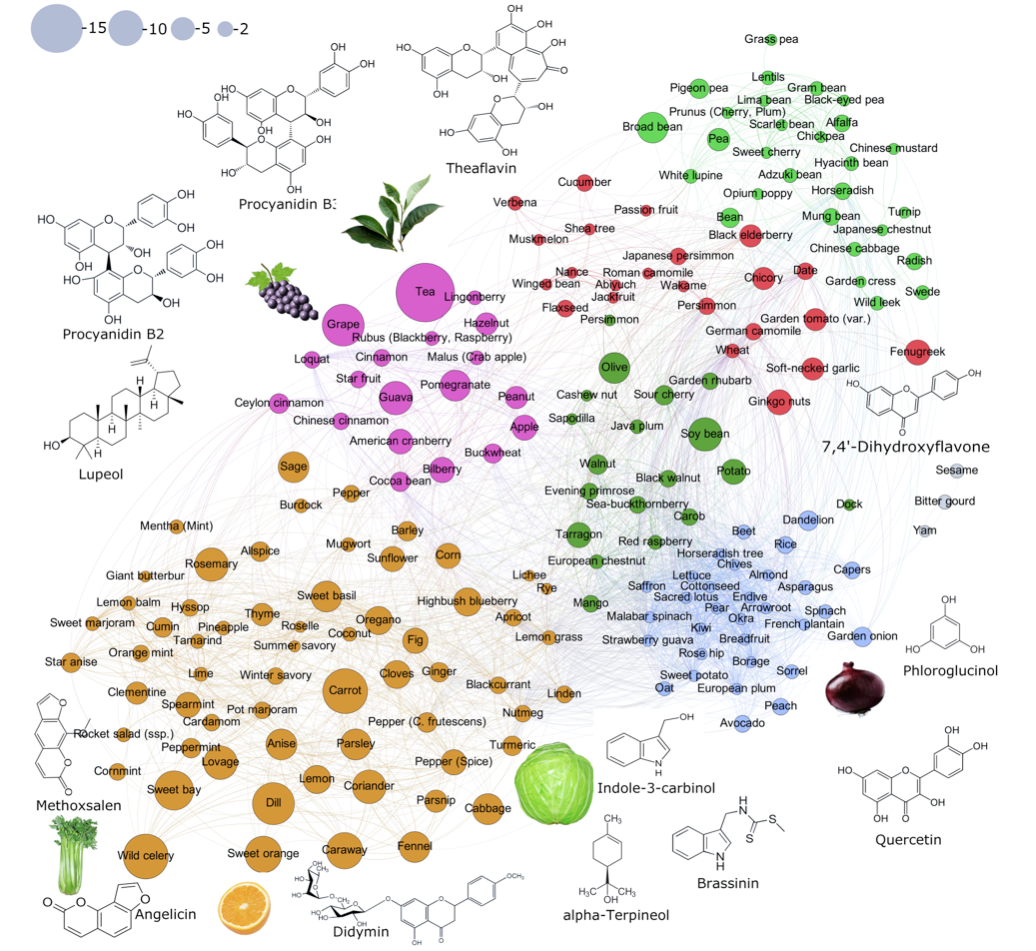
With this understanding, we constructed the anti-cancer molecular profiles of over 250 different food ingredients, highlighting prominent champions that we called “hyperfoods”. Besides the aforementioned tea and citruses, cabbage, celery, and sage are rather common, cheap, and broadly available hyperfoods. In a sense, this comes to no surprise, as many of these foods are advocated as healthy choices by nutrition experts and there is overwhelming evidence of their health benefits.
基于这种理解,我们构建了250多种不同食品成分的抗癌分子谱,突出了我们称为“超级食品”的杰出支持者。 除上述茶和柑桔外,白菜,芹菜和鼠尾草也很常见,便宜且广泛使用。 从某种意义上说,这不足为奇,因为营养专家主张将这些食物中的许多作为健康选择,并且有大量证据表明它们对健康有益。
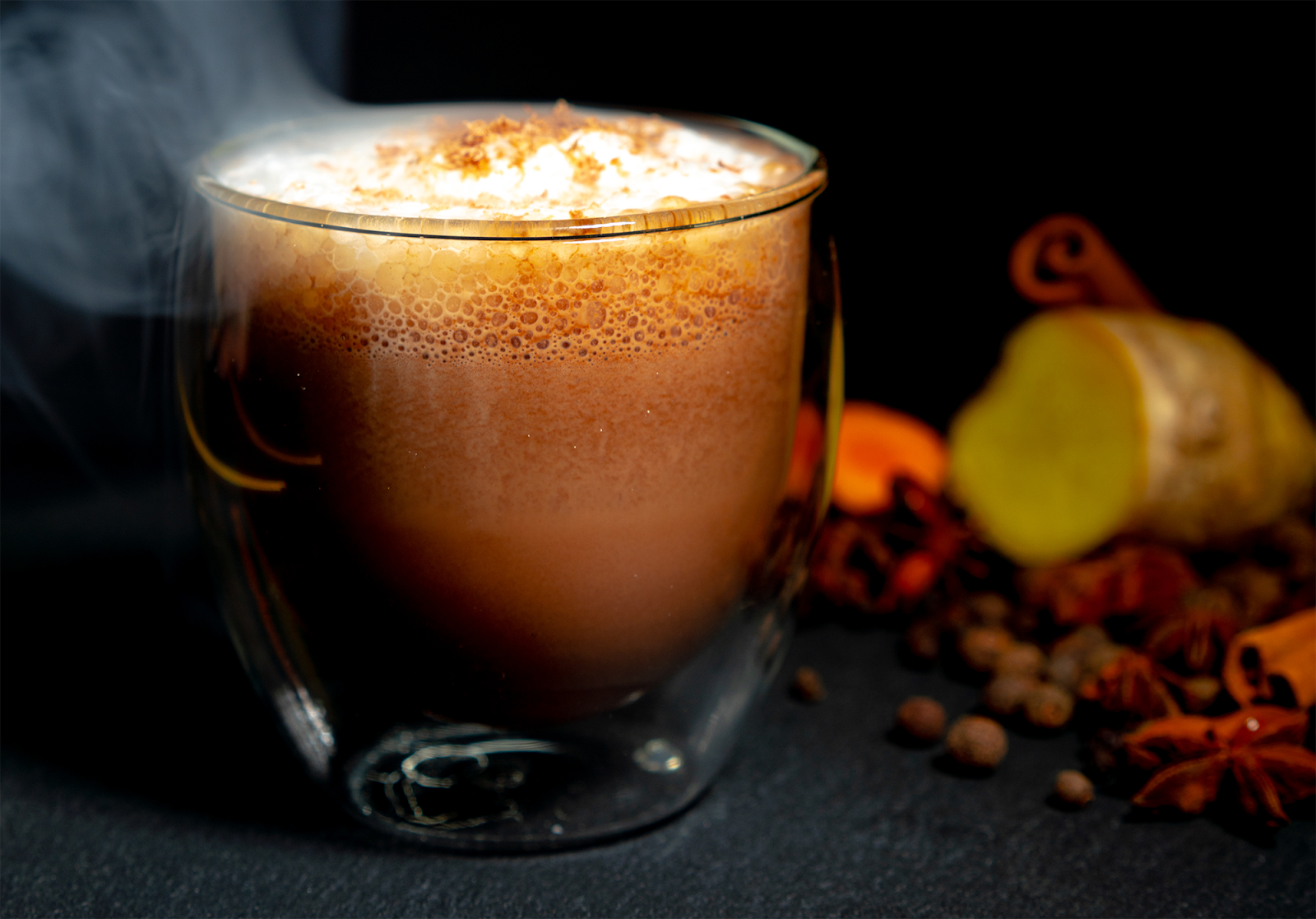
Do not however rush to make a cabbage smoothie after reading this post, as you will likely be disappointed — most chances are that it will taste awful. We are still missing a final step of putting the hyperfood ingredients together into recipes that taste and look great. This is where we availed to the help of Jozef Youssef, the founder and Chef Patron of Kitchen Theory [17], who created simple, affordable, and delicious recipes using our hyperfood ingredients. In fact, hyperfoods are not only for the Michelin restaurant-goers and fans of the haute cuisine: many simple, traditional, everyday recipes are already packed with cancer-beating ingredients.
但是,在阅读这篇文章后,不要着急制作卷心菜冰沙,因为您可能会感到失望-大多数机会是它的味道很糟糕。 我们仍然缺少将超级食物成分整合到味道和外观不错的食谱中的最后一步。 这就是我们在厨房理论[17]的创始人兼厨师长约瑟夫·尤塞夫(Jozef Youssef)的帮助下使用的,他们使用我们的超级食品成分制作了简单,实惠且美味的食谱。 实际上,超级食物不仅适合米其林餐厅的顾客和高级美食爱好者:许多简单,传统的日常食谱中都含有抗癌成分。
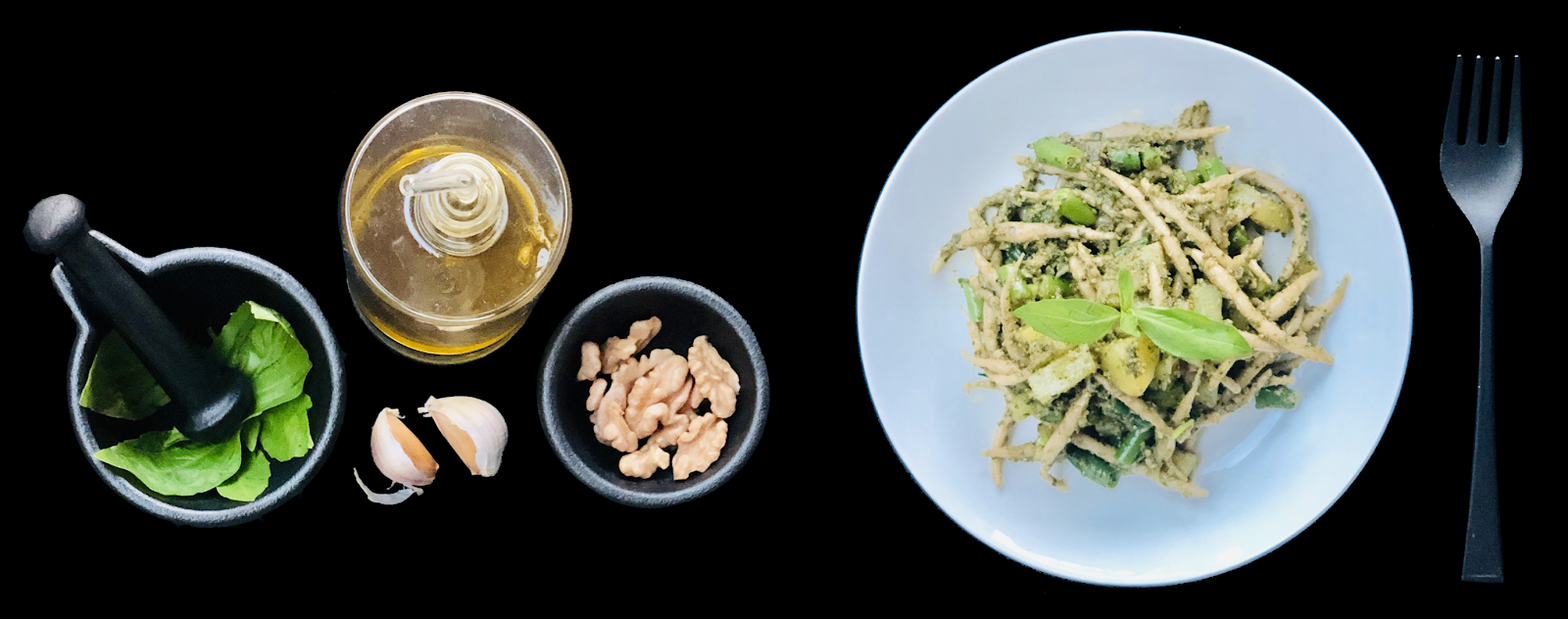
下一步 (Next steps)
We also need to bear in mind that food cooking involves physical and chemical processes that may change its molecular content. If for example, we fry our ingredients at high temperatures, many of the cancer-beating molecules are likely to disappear. We can represent food preparation as a computational graph with cooking transformations modeled as edges, and optimise it by choosing such operations that preserve in the best way the anti-cancer molecular composition.
我们还需要记住,食品烹饪涉及可能会改变其分子含量的物理和化学过程。 例如,如果我们在高温下油炸成分,许多抗癌分子可能会消失。 我们可以将食物制备表示为具有以边缘为模型的烹饪转换的计算图,并通过选择以最佳方式保留抗癌分子组成的操作对其进行优化。
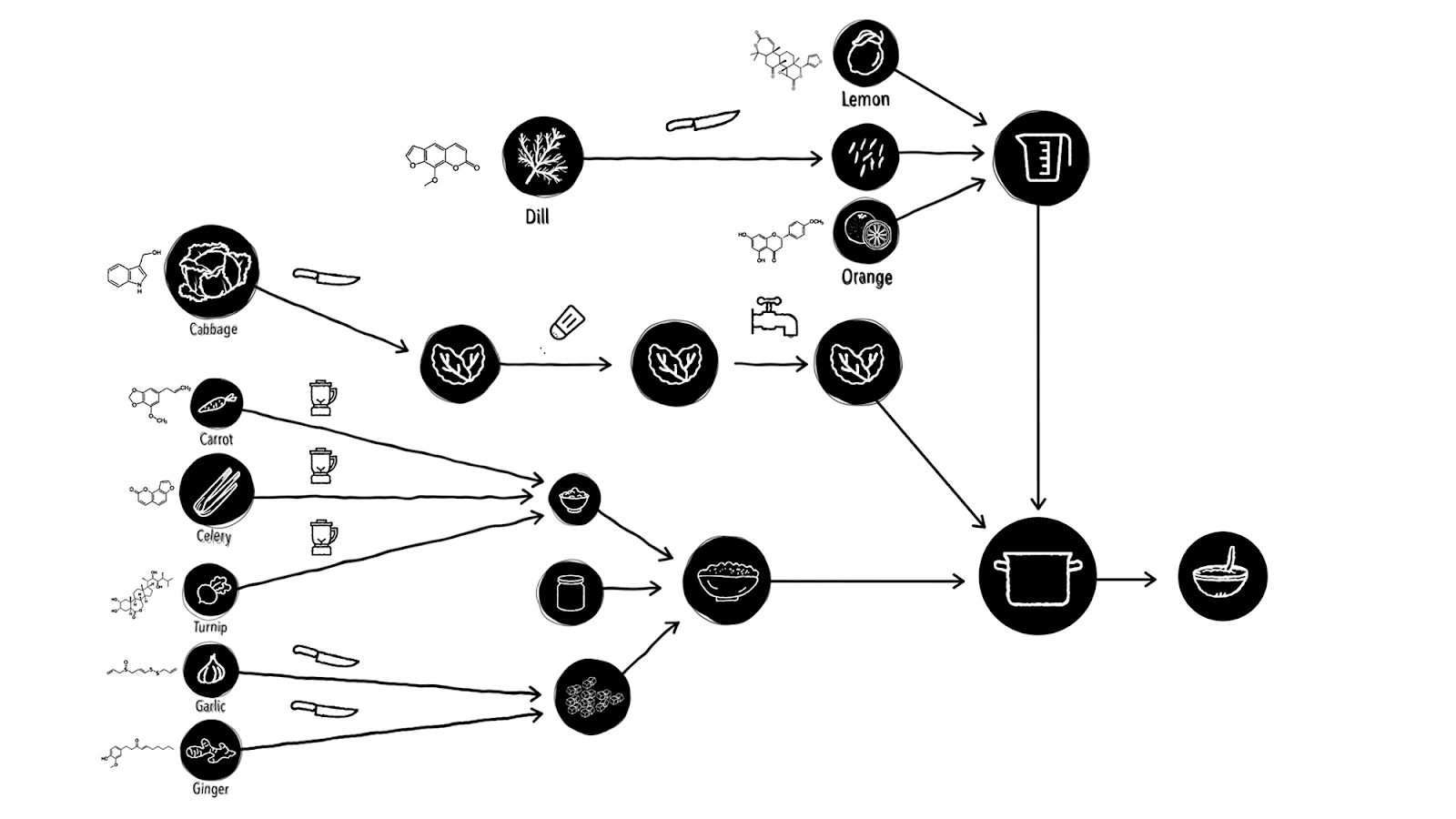
Second, in addition to cancer-beating molecules, food also contains molecules giving it taste, smell, and characteristic flavour [18]. Many foods share multiple such components: you would be surprised, for example, that garlic and tea have more than a hundred flavour molecules in common. The secret of food pairing is to combine ingredients with similar or complementary flavour molecular profiles [19]. And what was thought to be some kind of ”black magic” of top cuisiniers can now be automated — we can potentially use graph ML to generate recipes that strike the optimal balance between health, taste, and maybe even aesthetics. It is not impossible that one day our computer-generated recipes will even challenge Michelin-star chefs [20].
其次,除了抗癌分子之外,食物还包含赋予其味道,气味和特征风味的分子[18]。 许多食物具有多种这样的成分:例如,您会惊讶地发现大蒜和茶共有100多种风味分子。 食物配对的秘诀是将具有相似或互补风味分子特征的成分结合在一起[19]。 现在被认为是顶级美食家的某种“黑魔法”现在可以实现自动化-我们可以潜在地使用图ML生成在健康,口味甚至美观之间达到最佳平衡的食谱。 有一天我们的计算机生成的食谱甚至会挑战米其林星级厨师[20]。
Last but not least, when it comes to tastes, the only common truth is that de gustibus non est disputandum, as the Latin proverb goes. Recipe design must be highly personalised taking into account one’s taste preferences — but also many other parameters such as dietary restrictions, genetics, disease history, and the gut microbiome. We envision a future where everyone will have a digital “food passport” storing personal nutritional data, so when you order food online or eat out, your meal will be optimised for your health and food profile.
最后但并非最不重要的一点是,就口味而言,唯一的常识是,就像拉丁谚语所说的那样, de gustibus non est disputandum 。 食谱设计必须高度个性化,既要考虑个人的口味偏好,又要考虑许多其他参数,例如饮食限制,遗传学,疾病史和肠道微生物组。 我们设想了一个未来,每个人都将拥有数字“ 食品护照”存储个人营养数据,因此当您在线订购食物或外出吃饭时,您的餐食将针对您的健康和食物状况进行优化。
最后的想法 (Final thoughts)
Hyperfoods is the first attempt to apply graph-based ML methods in order to predict the health effects of biologically active molecules in foods by modelling the “network effects” of their interactions with biomolecules in our body. The use of graph ML methods allows us to identify which foods contain ingredients that might work in a similar way to medical drugs and have the potential to prevent or beat diseases. While cancer is an important class of diseases, the same approach can be applied to discover foods that can help prevent neurodegenerative, cardiovascular, or viral diseases [21].
Hyperfoods是首次尝试使用基于图的ML方法,以通过模拟食品中生物活性分子与人体中生物分子相互作用的“网络效应”来预测食品中的健康效应。 使用图ML方法可以使我们识别出哪些食物中的成分可能与医学药物相似,并且具有预防或预防疾病的潜力。 尽管癌症是一类重要的疾病,但可以采用相同的方法来发现有助于预防神经退行性疾病,心血管疾病或病毒性疾病的食品[21]。
In a longer perspective, our ambition is to provide a quantum leap in how our food is “prescribed”, designed, and prepared — to make us all live healthier, happier, and better lives.
从更长远的角度来看,我们的目标是在食物的“处方”,设计和准备方式上实现巨大飞跃,使我们所有人生活得更健康,快乐和美好。
[1] M. J. Prince et al., The burden of disease in older people and implications for health policy and practice (2015), Lancet 385:549–562.
[1] MJ Prince等人,《老年人的疾病负担及其对健康政策和实践的影响》(2015年),柳叶刀385:549-562。
[2] GBD 2017 Diet Collaborators, Health effects of dietary risks in 195 countries (2019), Lancet 393:1958–1972 found that 11 million deaths were attributable to dietary risk factors, of which 3 million are due to low intake of whole grains, and 2 million are due to low intake of fruits. For comparison, the WHO estimates that tobacco kills 8 million people each year.
[2] GBD 2017年饮食合作者,在195个国家中饮食风险对健康的影响(2019年),《柳叶刀》 393:1958–1972年发现,有1100万人死于饮食风险因素,其中300万人死于全谷物摄入量低,其中200万是由于水果摄入量少所致。 作为比较,世界卫生组织估计,烟草每年造成800万人死亡。
[3] M. S. Donaldson, Nutrition and cancer: a review of the evidence for an anti-cancer diet (2004), Nutrition Journal 3, estimates that 30%-40% of all cancers can be prevented by lifestyle and dietary measures alone and suggests that adhering to proposed dietary guidelines (that include recommended intake of cruciferous vegetables, flax seeds, and fruits) is likely to lead to a 60%–70% decrease in breast, colorectal, and prostate cancers and a 40%–50% decrease in lung cancer, along with similar reductions in cancers at other sites.
[3]唐纳森(MS Donaldson),《营养与癌症:抗癌饮食的证据综述》 (营养杂志3,2004年)估计,仅生活方式和饮食手段可预防所有癌症的30%-40%,并建议坚持建议的饮食指南(包括建议摄入十字花科蔬菜,亚麻籽和水果)很可能导致乳腺癌,结肠直肠癌和前列腺癌减少60%–70%,而乳腺癌减少40%–50%肺癌,以及其他部位癌症的类似减少。
[4] Experimental studies suggest that these molecules participate in multiple mechanisms contributing to the prevention or treatment of various cancers, including regulating the activity of inflammatory mediators and growth factors, suppressing cancer cell survival, proliferation, and invasion, as well as angiogenesis and metastasis. See e.g. A. K. Singh et al., Emerging importance of dietary phytochemicals in fight against cancer: Role in targeting cancer stem cells (2017), Critical Reviews in Food Science and Nutrition 57: 3449–3463 or R. Baena Ruiz and P. Salinas Hernandez, Cancer chemoprevention by dietary phytochemicals: Epidemiological evidence (2016), Maturitas 94:13–19.
[4]实验研究表明,这些分子参与多种机制来预防或治疗各种癌症,包括调节炎症介质和生长因子的活性,抑制癌细胞的存活,增殖和侵袭以及血管生成和转移。 。 参见例如AK Singh等人,《饮食中的植物化学物质在抗击癌症中的新兴重要性:在靶向癌症干细胞中的作用》(2017),《食品科学与营养评论》 57:3449–3463或R. Baena Ruiz和P. Salinas Hernandez ,饮食植物化学物质对癌症的化学预防:流行病学证据(2016年),Maturitas 94:13-19。
[5] This analogy to “dark matter” in physics appeared in R. R. da Silva et al., Illuminating the dark matter in metabolomics (2015), PNAS 112(41):12549–12550 as well as in A.-L. Barabási et al., The unmapped chemical complexity of our diet (2019), Nature Food 1:33–37.
[5]物理学上与“暗物质”的类比出现在RR da Silva等人的文章《代谢组学中的暗物质照亮》(2015),PNAS 112(41):12549–12550以及A.-L中。 Barabási等人,《我们饮食中未映射的化学复杂性》(2019年),《自然食品》 1:33–37。
[6] Many drugs are derived from plants, which is often reflected in their names: for example, ephedrine takes its name from the plant genus Ephedra, atropine from the belladonna plant (Atropa belladonna), and the acetylsalicylic acid, commonly known as aspirin, from the bark of the willow tree (Salix alba), whose medicinal properties are known since antiquity. In oncological medicine, prominent examples are the analogs of the natural compound camptothecin, which is extracted from the Camptotheca acuminata tree and also well-known in traditional medicine. Four such molecules — topotecan, irinotecan, belotecan, and trastuzumab deruxtecan — are widely used in cancer chemotherapy. D. J. Newman and G. M. Cragg, Natural products as sources of new drugs from 1981 to 2014 (2016), Journal of Natural Products 79(3):629–661 report that almost half of anti-cancer therapies are derived from natural products.
[6]许多药物都来自植物,通常会在名称中体现出来:例如,麻黄碱的名称来自植物麻黄属,其名称来自颠茄植物( Atropa belladonna )的阿托品,以及乙酰水杨酸(俗称阿司匹林) ,来自柳树( Salix alba )的树皮,其药用特性自上古以来就为人所知。 在肿瘤医学中,著名的例子是天然化合物喜树碱的类似物,它是从喜树树中提取的,也是传统医学中众所周知的。 四种这样的分子-拓扑替康,伊立替康,贝洛特坎和曲妥珠单抗德鲁替康-被广泛用于癌症化疗。 DJ Newman和GM Cragg,1981年至2014年的天然产物作为新药的来源(2016年),《天然产物杂志》 79(3):629-661报告说,几乎一半的抗癌疗法均来自天然产物。
[7] Proteins are the powerhouses of living cells and are literally the “molecules of life”, as we are currently unaware of any life forms that are not protein-based. In our body, proteins are responsible for catalysing chemical reactions (enzymes), giving structure to tissues (collagen), transporting oxygen (haemoglobin), and defending us against pathogens (antibodies), to mention a few. Proteins are synthesised in cells by a special chemical mechanism that reads out the genetic code and translates it into a sequence of aminoacids: short sequences of DNA nucleotides called “codons” encode the 20 proteinogenic aminoacids. We have around 20 thousands proteins encoded in our genome that interact with each other and with other molecules. Because of the crucial role of proteins in biochemical processes, they are used as drug targets: typical drugs are small molecules designed in such a way that they can chemically attach themselves (“bind”) to specific proteins.
[7]蛋白质是活细胞的强大力量,从字面上讲是“生命分子”,因为我们目前不知道任何不基于蛋白质的生命形式。 在我们的身体中,蛋白质负责催化化学React(酶),赋予组织结构(胶原蛋白),输送氧气(血红蛋白)并防御病原体(抗体),仅举几例。 蛋白质是通过一种特殊的化学机制在细胞中合成的,该机制读出遗传密码并将其翻译为氨基酸序列:称为“密码子”的DNA核苷酸短序列编码20种蛋白质氨基酸。 我们的基因组中编码着约2万种蛋白质,它们相互之间以及与其他分子相互作用。 由于蛋白质在生化过程中的关键作用,因此它们被用作药物靶标:典型的药物是小分子,设计方式使得它们可以将自身化学结合(“结合”)到特定蛋白质上。
[8] It is estimated that a drug molecule can bind to nearly 50 proteins, see B. Srinivasan et al. Experimental validation of FINDSITE(comb) virtual ligand screening results for eight proteins yields novel nanomolar and micromolar binders (2014), Cheminformatics 6:16, so the one drug–one target assumption is very far from reality.
[8]据估计,一种药物分子可以结合近50种蛋白质,请参见B. Srinivasan等。 对8种蛋白质的FINDSITE(comb)虚拟配体筛选结果进行实验验证可产生新颖的纳摩尔和微摩尔结合物(2014年),化学信息学6:16,因此,一种药物-一种目标假设与现实相距甚远。
[9] Protein-protein interactions are one example of a graph that are exploited in “network medicine”, a term coined and popularised in A.-L. Barabási, Network medicine — from obesity to the “diseasome” (2007), New England Journal of Medicine 357:404–407.
[9]蛋白质-蛋白质相互作用是在“网络医学”中使用的图形的一个示例,该术语是在A.-L中创造和普及的。 巴拉巴西(Barabási),网络医学-从肥胖到“两栖类”(2007年),新英格兰医学杂志357:404-407。
[10] A. Mullard, Protein-protein interaction inhibitors get into the groove (2012). Nature Review Drug Discovery 11(3):173–175 calls PPI targets an “unmined gold reserve”. Macrocycles are one example of drug-like small molecules that disrupt protein-protein interaction and accelerate cancer cell death. Despite their therapeutic relevance and untapped abundance, their adoption is hindered by technical hurdles, see T. L. Nero et al., Oncogenic protein interfaces: small molecules, big challenges (2014), Nature Review Cancer 14(4):248–262 and D. E. Scott et al., Small molecules, big targets: drug discovery faces the protein–protein interaction challenge (2016), Nature Review Drug Discovery 15:533–550.
[10] A. Mullard,蛋白质相互作用抑制剂(2012)。 Nature Review Drug Discovery 11(3):173-175称PPI目标为“未开采的黄金储备”。 大环化合物是破坏蛋白质与蛋白质相互作用并加速癌细胞死亡的类药物小分子的一个例子。 尽管它们具有治疗相关性和未开发的丰度,但它们的采用仍然受到技术障碍的阻碍,请参见TL Nero等人,《致癌蛋白界面:小分子,大挑战》(2014),《自然评论》癌症14(4):248–262和DE Scott等,小分子,大目标:药物发现面临蛋白质间相互作用的挑战(2016年),《自然评论药物发现》 15:533–550。
[11] See M. M. Bronstein et al. Geometric deep learning: going beyond Euclidean data (2017), IEEE Signal Processing Magazine 34(4):18–42 and my blog posts on this topic.
[11]参见MM Bronstein等。 几何深度学习:超越欧几里得数据(2017),IEEE信号处理杂志34(4):18–42和我有关此主题的博客文章。
[12] K. Veselkov et al., HyperFoods: Machine intelligent mapping of cancer-beating molecules in foods (2019), Scientific Reports 9.
[12] K. Veselkov等人,《 HyperFoods:食品中抗癌分子的机器智能作图》 (2019年),《科学报告》 9。
[13] The amount of scientific literature on cancer is enormous, with one paper published every 3 to 4 minutes on average, making it impossible to digest even for the most industrious human scientists. We used an NLP system for named entity recognition developed earlier by D. Galea et al., Exploiting and assessing multi-source data for supervised biomedical named entity recognition (2018), Bioinformatics 34(14):2474–2482. The supplementary materials to our paper provide a detailed list of compounds found in food and the experimental evidence of their anti-cancer effects.
[13]有关癌症的科学文献数量巨大,平均每3至4分钟发表一篇论文,即使对于最勤奋的人类科学家来说,也无法消化。 我们使用了D. Galea等人先前开发的NLP系统进行命名实体识别,利用和评估受监督的生物医学命名实体识别的多源数据(2018),生物信息学34(14):2474–2482。 我们论文的补充材料详细列出了食品中发现的化合物,并提供了其抗癌作用的实验证据。
[14] Apples are a good example of why one has to consider the antagonistic or synergistic effects of multiple compounds: apple extracts contain bioactive compounds that have been shown to inhibit tumor cell growth in vitro. Yet, the effect varies greatly depending on whether the peel is preserved: apples with peel inhibit colon cancer cell proliferation by 43% vs only 29% for apples without peel, see M. V. Eberhardt et al. Antioxidant activity of fresh apples (2000), Nature 405:903–904.
[14]苹果是一个很好的例子,说明了为什么必须考虑多种化合物的拮抗作用或协同作用:苹果提取物包含的生物活性化合物已被证明在体外抑制肿瘤细胞的生长。 然而,根据果皮是否被保存,其效果差异很大:有果皮的苹果抑制结肠癌细胞增殖43%,而无果皮的苹果则抑制29%,参见MV Eberhardt等。 新鲜苹果的抗氧化活性(2000年),《自然》 405:903–904。
[15] M. Zitnik et al., Modeling polypharmacy side effects with graph convolutional networks (2018), Bioinformatics 34(13):457–466, applied graph ML to protein-protein and protein-drug interaction graphs in order to predict the side effects of polypharmacy.
[15] M. Zitnik等人,利用图卷积网络建模多药副作用(2018),生物信息学34(13):457-466,将图ML应用于蛋白质-蛋白质和蛋白质-药物相互作用图,以预测药房的副作用。
[16] Tea is a rich source of catechins (epigallocatechingallate), terpenoids (lupeol), and tannins (procyanidin), which exert strong and complementary anti-cancer effects, by protecting reactive oxidative species induced DNA damage, suppressing inflammation, and inducing apoptosis and cancer cell cycle arrest, respectively. Several recent meta-analyses demonstrated that the consumption of green tea leads to delayed cancer onset, lower rates of cancer recurrence after treatment, and increased rates of long-term cancer remission, see V. Gianfredi et al. Green tea consumption and risk of breast cancer and recurrence-A systematic review and meta-analysis of observational studies (2018), Nutrients 10, and Y. Guo et al. Green tea and the risk of prostate cancer: A systematic review and meta-analysis (2017), Medicine 96(13). The second example, sweet orange, is a citrus fruit containing compounds dydimin (citrus flavonoid), obacunone (limonoid glucose) and β-elemene, known for their strong antioxidant, pro-apoptotic, and chemosensitization effects. The inverse association between citrus fruit intake and incidence of cancer was shown in S. Cirmi et al. Anticancer potential of citrus juices and their extracts: A systematic review of both preclinical and clinical studies (2017), Frontiers in Pharmacology 8.
[16]茶是儿茶素(epigallocatechingallate),萜类化合物(lupeol)和丹宁酸(原花青素)的丰富来源,它们通过保护React性氧化物种引起的DNA损伤,抑制炎症和诱导凋亡而发挥强大而互补的抗癌作用。和癌细胞周期阻滞。 最近的一些荟萃分析表明,食用绿茶会导致癌症发作延迟,治疗后癌症复发率降低以及长期癌症缓解率增加,请参见V. Gianfredi等。 绿茶的消费以及乳腺癌和复发的风险-观察性研究的系统综述和荟萃分析(2018),Nutrients 10和Y. Guo等。 绿茶与前列腺癌的风险:系统评价和荟萃分析(2017),医学96(13)。 第二个例子是甜橙,是一种柑橘类水果,含有化合物dydimin(柑橘类黄酮),obacunone(柠檬苦素葡萄糖)和β-榄香烯,它们以强大的抗氧化剂,促凋亡和化学增敏作用而闻名。 S. Cirmi等人(2006年)显示了柑橘类水果摄入量与癌症发生率之间的负相关关系。 柑橘汁及其提取物的抗癌潜力:对临床前和临床研究的系统评价(2017),药理学前沿8。
[17] I first met Kirill at the World Economic Forum meeting in 2015. We have quickly become friends in part due to our shared Russian background, and then colleagues once I joined Imperial College in 2018. Kirill met Jozef at the conference of Future of Computing and Food in 2018. Jozef is the Chef Patron of Kitchen theory, a high-end restaurant that looks like a chemical lab and where guests are invited to participate in psychophysical experiments such as eating jellyfish while listening to crunchy sounds (this changes the perception of food’s taste).
[17]我在2015年世界经济论坛会议上初次遇见基里尔。部分原因是由于我们具有共同的俄罗斯背景,因此我很快成为朋友。然后,当我于2018年加入帝国理工学院时,我的同事们。基里尔在未来世界会议上遇见了约瑟夫。 2018年的计算机和食品。Jozef是厨房理论的主厨,这是一家看起来像化学实验室的高端餐厅,邀请宾客参加心理物理实验,例如一边听脆脆的声音一边吃海el(这改变了人们的看法食物的味道)。
[18] FlavorDB is an online resource allowing us to explore over 25 thousand flavour molecule content in nearly 1000 foods.
[18] FlavorDB是一个在线资源,使我们能够探索近1000种食物中的25,000多种风味分子含量。
[19] Y.-Y. Ahn et al., Flavor network and the principles of food pairing (2011). Scientific Reports 1 showed by analysing the graph of shared flavour molecules, that Western cuisines tend to use ingredient pairs with common flavour compounds, whereas East Asian cuisines tend to avoid compound-sharing ingredients.
[19]是。 Ahn等人,《风味网络与食物配对的原理》 (2011年)。 《科学报告1》通过分析共享风味分子的图表显示,西方美食倾向于使用具有常见风味成分的配料对,而东亚美食则倾向于避免化合物共享的配料。
[20] We are not the first to work on automatic recipe generation: multiple attempts have been done, with the most notable being the IBM Cognitive Cooking project. However, we are the first, to the best of my knowledge, to go beyond flavour and try to account for bioactive molecules.
[20]我们并不是第一个致力于自动配方生成的公司:已经进行了多次尝试,其中最著名的是IBM Cognitive Cooking项目。 但是,就我所知,我们是第一个超越风味并尝试解释生物活性分子的公司。
[21] The use of graph ML appears very promising in repurposing existing drugs for new diseases (“drug repositioning”), which can dramatically cut the costs and times of developing new therapies. As part of projects DRUGS and CoronaAI, we are currently using the Vodafone distributed computing platform DreamLab to find antiviral compounds in food and combinations of existing drugs that could have a therapeutic effect on cancer and COVID-19. I also serve as a scientific advisor to the pharmaceutical startup Relation Therapeutic that, in collaboration with Mila and the Gates Foundation, is combining graph ML with active learning techniques to find combinatorial therapy against COVID-19.
[21]在重新使用现有药物治疗新疾病(“药物重新定位”)方面,使用图ML看起来非常有前途,这可以大大降低开发新疗法的成本和时间。 作为DRUGS和CoronaAI项目的一部分,我们目前正在使用Vodafone分布式计算平台DreamLab来寻找食品中的抗病毒化合物以及可能对癌症和COVID-19有治疗作用的现有药物组合。 我还担任制药创业公司Relation Therapeutic的科学顾问,该公司与Mila和盖茨基金会合作,正在将图ML与主动学习技术结合起来,以找到针对COVID-19的组合疗法。
I am grateful to Fabrizio Frasca, Luca Sbordone, and David Silver for proof-reading this post. Hyperfoods is a project at Imperial College led by Kirill Veselkov in collaboration with Vodafone Foundation and Kitchen Theory. Nothing in this post is intended as medical advice: the relationship between food and disease remains an emerging field of study and systematic clinical validation is still lacking. Recipes from Chef Jozef Youssef are available online in the first Hyperfoods Cookbook. For additional details about deep learning on graphs, see other posts on Medium, or follow me on Twitter.
我感谢Fabrizio Frasca,Luca Sbordone和David Silver校对了这篇文章。 Hyperfoods是帝国学院的一个项目,由Kirill Veselkov领导,与Vodafone Foundation和Kitchen Theory合作。 本文中没有任何内容可作为医学建议:食物与疾病之间的关系仍然是一个新兴的研究领域,而仍然缺乏系统的临床验证。 主厨Jozef Youssef的食谱可在第一本Hyperfoods Cookbook中在线获得。 有关图上深度学习的更多详细信息,请参阅Medium上的其他帖子,或在Twitter上关注我。
超级符号就是超级创意