以太网帧数据帧
Imagine you have a spreadsheet of employee data. You have their name, their age, their contact information, etc. This data changes over time as employees enter and/or leave the company, or simply update their information.
假设您有一个员工数据电子表格。 您可以输入他们的姓名,年龄,联系信息等。随着员工进入和/或离开公司,或者只是更新他们的信息,此数据会随着时间而变化。
In today’s tutorial, I will show you how to update a pandas DataFrame in this professional context to keep track of the employee status: if they are still present in the new data(frame), then we just update their information; otherwise we assume they have left the company. Brand new employees will simply be added to our dataset. We’ll assume we get a new listing of our employees in regular intervals and all current employees are included in that listing. We then use that listing to update our dataset with the latest data.
在今天的教程中,我将向您展示如何在这种专业背景下更新熊猫DataFrame以跟踪员工的状态:如果它们仍存在于新数据(框架)中,那么我们只更新其信息; 否则,我们假设他们已经离开公司。 只需将新员工添加到我们的数据集中即可。 我们假设我们会定期获取新的员工列表,并且当前所有员工都包含在该列表中。 然后,我们使用该清单用最新数据更新我们的数据集。
For instance, according to the dataset at this point in time
例如,根据此时的数据集
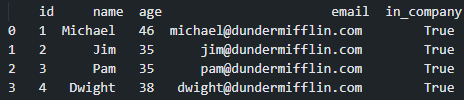
The company only has four employees.
该公司只有四名员工。
However, we just got a new employee listing and it looks like this
但是,我们刚得到一个新员工列表,它看起来像这样
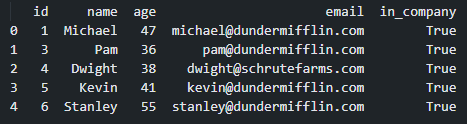
Over time we got two new employees (Kevin and Stanley with ids 5 and 6), but the employee with id 2 is missing. In the context of this demo we’ll interpret this as that employee leaving the company. We’ll update the first DataFrame with the new data, but the ids won’t reset. So at the end, the employee dataset will look like this
随着时间的流逝,我们有两名新员工(编号分别为5和6的凯文和斯坦利),但缺少编号为2的员工。 在本演示的上下文中,我们将其解释为该雇员离开公司。 我们将使用新数据更新第一个DataFrame,但ID不会重置。 所以最后,员工数据集将如下所示
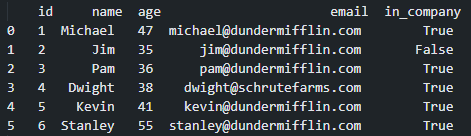
So that we know who has been a part of our company over time, we see that Jim (id 2) is still in the dataset, and Kevin and Stanley are now a part of the company. However, Jim, the only “original” employee that was not in the latest listing, now has a “in_company” value of False
, that is, he left the company. Notice also that Michael and Pam are one year older and Dwight has changed his email address. For employees that leave the company we’ll keep whatever was the last data we had about them (age and email in this demo).
为了弄清谁是我们公司的一员,我们看到Jim(id 2)仍在数据集中,而Kevin和Stanley现在已成为公司的一部分。 但是,吉姆(Jim)是唯一不在最新列表中的“原始”员工,现在的“公司内”值为False
,也就是说,他离开了公司。 另请注意,Michael和Pam已满一岁,而Dwight更改了他的电子邮件地址。 对于离开公司的员工,我们将保留关于他们的最新数据(此演示中的年龄和电子邮件)。
代码 (The code)
Okay, now that you have seen the results of the code, let’s dive into the code.
好的,既然您已经看到了代码的结果 ,那么让我们深入研究一下代码。
import pandas as pd
import numpy as np
# Current employee dataset
original = pd.DataFrame({
"id": [1, 2, 3, 4],
"name": ["Michael", "Jim", "Pam", "Dwight"],
"age": [46, 35, 35, 38],
"email": ["michael@dundermifflin.com", "jim@dundermifflin.com", "pam@dundermifflin.com", "dwight@dundermifflin.com"],
"in_company": [True, True, True, True]
})
# The latest employee listing. It has two new employees (ids 5 and 6)\
# and is missing one person from the previous data (id 2). Some employees\
# also updated their data
new_data = pd.DataFrame({
"id": [1, 3, 4, 5, 6],
"name": ["Michael", "Pam", "Dwight", "Kevin", "Stanley"],
"age": [47, 36, 38, 41, 55],
"email": ["michael@dundermifflin.com", "pam@dundermifflin.com", "dwight@schrutefarms.com", "kevin@dundermifflin.com", "stanley@dundermifflin.com"],
"in_company": [True, True, True, True, True]
})
# Update the employment status of employees missing in the new listing
original.loc[~original["id"].isin(new_data["id"]), "in_company"] = False
# Append the new listing to the original to create a single DF
updated = original.append(new_data, ignore_index=True)
# For the employees in both DFs, keep only their latest information\
# (rows are considered duplicate based on the "id")
updated = updated.drop_duplicates(["id"], keep="last")
# Sort the employees by their id
updated.sort_values("id", inplace=True)
# Reset the DF index after all the transformations (don't keep the\
# previous index a.k.a. `drop=True`)
updated.reset_index(drop=True, inplace=True)
print("---Updated employee data:\n", updated)
As you can see in the code gist above, we only need a handful of lines to update the DataFrame. A good chunk of the script is to create the DataFrames since we are not loading any external files to keep the demo simple.
正如您在上面的代码要点中看到的那样,我们只需要少量的行即可更新DataFrame。 该脚本的很大一部分是创建DataFrame,因为我们没有加载任何外部文件来简化演示。
So, the DataFrames are exactly the same you’ve seen in the beginning, but to remind ourselves, this is the original employee dataset
因此,DataFrame与您一开始所看到的完全相同,但是提醒自己,这是原始的员工数据集
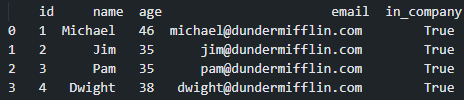
And this is the latest listing we got, that is, the data used to update the dataset
这是我们得到的最新清单,即用于更新数据集的数据
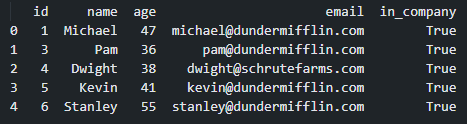
Again, notice how Jim is not in the new listing, that is, we assume he has left the company. On the other hand, new people have joined and others have changed their information.
再一次,请注意吉姆如何不在新的上市中,也就是说,我们假设他已离开公司。 另一方面,新人加入了,其他人改变了他们的信息。
Moving on to the important code: line 23 of the code gist. This is probably the most important line, as we locate all rows whose employee id is in the “id” column of both the original and the new DataFrames. Since the data we want to change for employees that left the company is in the “in_company” column, we also specify that column to change the values of all matching cells to False
.
转到重要的代码:代码要点的第23行。 这可能是最重要的一行,因为我们找到了雇员ID在原始DataFrame和新DataFrame的“ id”列中的所有行。 由于我们要为离开公司的员工更改的数据位于“ in_company”列中,因此我们还指定了该列,以将所有匹配单元格的值更改为False
。
To make that code clearer, the original["id"].isin(new_data["id"])
part returns a pandas Series of boolean values where True
means the employee id is present in both DataFrames and False
otherwise.
为了使代码更清晰, original["id"].isin(new_data["id"])
部分返回pandas系列的布尔值,其中True
表示员工ID同时出现在DataFrames中,否则为False
。

As expected, only Jim, the second employee of the four original employees, returns False
because his id is only present in the first DataFrame. Michael, Pam and Dwight are in both DataFrames, so the id check returns True
. The tilde (~) that precedes this check
不出所料,只有四个原始雇员中的第二个雇员Jim,返回False
因为他的ID仅出现在第一个DataFrame中。 Michael,Pam和Dwight都在DataFrame中,因此id检查返回True
。 检查之前的波浪号(〜)
~original["id"].isin(new_data["id"])
“inverts” the values of the check. In other words, only Jim has a value of True
now. This is important because the values of the “in_company” column will only be changed for rows that return True
in this check. In other words, we find which employees are in both DataFrames, and select the other ones to update the employment status accordingly.
“反转”检查的值。 换句话说,只有Jim现在具有True
的值。 这很重要,因为“ in_company”列的值仅会针对在此检查中返回True
行进行更改。 换句话说,我们找到两个数据框架中的哪些雇员,然后选择其他雇员以相应地更新雇佣状态。
The rest of the code is pretty simple. On line 27 we append the new DataFrame to the original DataFrame that now has the employment statuses updated. Keep in mind we can safely append the rows of new data because the DataFrames’ columns match, most importantly their column names and data types. However, this operation creates duplicate entries for the employees that were already recorded.
其余代码非常简单。 在第27行,我们将新的DataFrame追加到现在已更新了雇佣状态的原始DataFrame中。 请记住,我们可以安全地附加新数据的行,因为DataFrame的列匹配,最重要的是它们的列名和数据类型。 但是,此操作为已经记录的员工创建重复的条目。

This is the purpose of line 30. It looks at the “id” column to find duplicate ids and only keeps their last occurrence. Since the new DataFrame had the up to date information of all employees, we assume the age and email in that new DataFrame is what we want to keep about them.
这是第30行的目的。它查看“ id”列以查找重复的id,并仅保留其最后一次出现。 由于新的DataFrame具有所有雇员的最新信息,因此我们假设新DataFrame中的年龄和电子邮件是我们想要保留的员工信息。
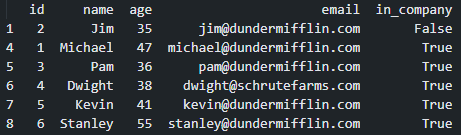
The operations on lines 32 and 35 are just some housekeeping. We sort the resulting DataFrame by employee “id” from oldest to most recent employee, and reset the DataFrame index (not the ids!) to have a nice default index.
32和35行的操作只是一些内部管理。 我们将按员工“ id”从最早到最近的员工对生成的DataFrame进行排序,然后重置DataFrame索引(而不是ID!)以拥有一个不错的默认索引。
One last time, this is our employee data, updated with the most recent information and employment status
上一次,这是我们的员工数据,已更新为最新信息和雇佣状态
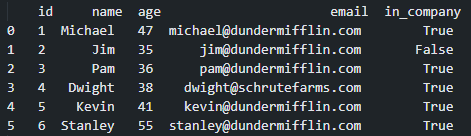
Based on the latest listing, Jim is no longer part of the company, but Kevin and Stanley joined at some point. Michael, Pam and Dwight also updated their information.
根据最新的上市信息,吉姆已不再是公司的一员,但凯文和斯坦利在某个时候加入了。 Michael,Pam和Dwight也更新了他们的信息。
结论 (Conclusion)
I had a specific scenario in mind and this was the demo I created as a proof of concept. I think it is an important data transformation in pandas, to change the values of a column in a DataFrame, based on the presence of the values of another column in a different DataFrame.
我有一个特定的场景,这是我作为概念证明创建的演示。 我认为,根据不同DataFrame中另一列的值的存在来更改DataFrame中列的值,这对熊猫来说是重要的数据转换。
I’ve struggled to put this transformation into words when searching it online, but I hope this article now offers you a solution if you come across this problem in the future.
在网上搜索时,我一直在努力地将这种转换表达出来,但是我希望本文现在为您提供解决方案,如果您将来遇到此问题的话。
As usual, the code is available on my GitHub repository.
和往常一样,该代码在我的GitHub存储库中可用。
翻译自: https://medium.com/swlh/update-a-dataframe-based-on-common-values-of-two-dataframes-eb4676edece0
以太网帧数据帧