机器学习 预测模型
数据科学 , 机器学习 (Data Science, Machine Learning)
前言 (Preface)
Cardiovascular diseases are diseases of the heart and blood vessels and they typically include heart attacks, strokes, and heart failures [1]. According to the World Health Organization (WHO), cardiovascular diseases like ischaemic heart disease and stroke have been the leading causes of deaths worldwide for the last decade and a half [2].
心血管疾病是心脏和血管疾病,通常包括心脏病发作,中风和心力衰竭[1]。 根据世界卫生组织(WHO)的研究,在过去的15年中,缺血性心脏病和中风等心血管疾病已成为全球死亡的主要原因[2]。
动机 (Motivation)
A few months ago, a new heart failure dataset was uploaded on Kaggle. This dataset contained health records of 299 anonymized patients and had 12 clinical and lifestyle features. The task was to predict heart failure using these features.
几个月前,一个新的心力衰竭数据集被上传到Kaggle上 。 该数据集包含299名匿名患者的健康记录,并具有12种临床和生活方式特征。 他们的任务是使用这些功能来预测心力衰竭。
Through this post, I aim to document my workflow on this task and present it as a research exercise. So this would naturally involve a bit of domain knowledge, references to journal papers, and deriving insights from them.
通过这篇文章,我旨在记录我有关此任务的工作流程,并将其作为研究练习进行介绍。 因此,这自然会涉及到一些领域知识,对期刊论文的引用以及从中得出的见解。
Warning: This post is nearly 10 minutes long and things may get a little dense as you scroll down, but I encourage you to give it a shot.
警告:这篇文章将近10分钟,当您向下滚动时,内容可能会变得有些密集,但我建议您试一试。
关于数据 (About the data)
The dataset was originally released by Ahmed et al., in 2017 [3] as a supplement to their analysis of survival of heart failure patients at Faisalabad Institute of Cardiology and at the Allied Hospital in Faisalabad, Pakistan. The dataset was subsequently accessed and analyzed by Chicco and Jurman in 2020 to predict heart failures using a bunch of machine learning techniques [4]. The dataset hosted on Kaggle cites these authors and their research paper.
该数据集最初由Ahmed等人在2017年发布[3],作为他们对巴基斯坦费萨拉巴德心脏病研究所和联合王国费萨拉巴德联合医院心力衰竭患者生存率分析的补充。 随后,Chicco和Jurman于2020年访问并分析了该数据集,以使用一系列机器学习技术预测心力衰竭[4]。 Kaggle托管的数据集引用了这些作者及其研究论文。
The dataset primarily consists of clinical and lifestyle features of 105 female and 194 male heart failure patients. You can find each feature explained in the figure below.
该数据集主要由105位女性和194位男性心力衰竭患者的临床和生活方式特征组成。 您可以找到下图中说明的每个功能。
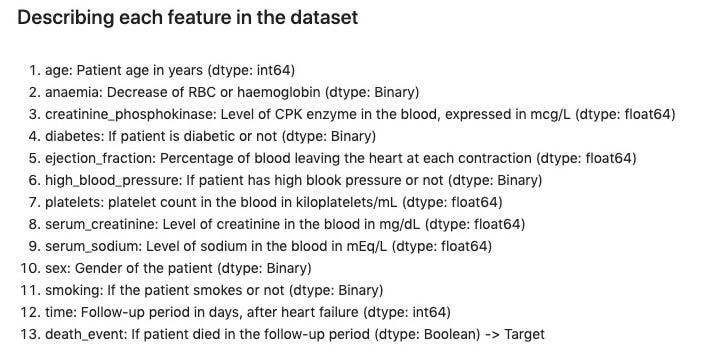
项目工作流程 (Project Workflow)
The workflow would be pretty straightforward —
工作流程将非常简单-
Data Preprocessing — Cleaning the data, imputing missing values, creating new features if needed, etc.
数据预处理-清理数据,估算缺失值,根据需要创建新功能等。
Exploratory Data Analysis — This would involve summary statistics, plotting relationships, mapping trends, etc.
探索性数据分析-这将涉及摘要统计,绘制关系,绘制趋势等。
Model Building — Building a baseline prediction model, followed by at least 2 classification models to train and test.
建立模型—建立基线预测模型,然后建立至少两个分类模型以进行训练和测试。
Hyper-parameter Tuning — Fine-tune the hyper-parameters of each model to arrive at acceptable levels of prediction metrics.
超参数调整-微调每个模型的超参数,以达到可接受的预测指标水平。
Consolidating Results — Presenting relevant findings in a clear and concise manner.
合并结果—清晰,简明地陈述相关发现。
The entire project can be found as a Jupyter notebook on my GitHub repository.
整个项目都可以在我的 GitHub 存储库中 找到,作为Jupyter笔记本 。
让我们开始! (Let’s begin!)
数据预处理 (Data Preprocessing)
Let’s read in the .csv file into a dataframe —
让我们将.csv文件读入数据框-
df = pd.read_csv('heart_failure_clinical_records_dataset.csv')
df.info()
is a quick way to get a summary of the dataframe data types. We see that the dataset has no missing or spurious values and is clean enough to begin data exploration.