ai模型
The Latest in AI Technologies Reinvent Media and Marketing Analytics @ Allergan; Excerpt of the white paper
最新的AI技术重塑了媒体和营销分析@ Allergan; 白皮书摘录
Authors:
作者:
Akhil Sood, Associate Director @ Marketing Sciences, Allergan; Dr. Michael Proksch, Senior Director @ H2o.ai; Vijay Raghavan, Associate Vice President @ Marketing Sciences, Allergan
Allergan市场科学副总监Akhil Sood ; H2o.ai高级总监Michael Proksch博士; Allergan市场科学副总裁Vijay Raghavan
介绍 (Introduction)
The call for accountability in marketing has been growing over recent years to justify total investments in the area. Furthermore, to allocate budgets across different marketing channels, managers have been trying to better understand their contribution to sales. To model the impact of marketing on sales, time-series approaches have been proven to be successful. However, Allergan’s experience and academic research shows that marketing attribution models are challenging as the complex relationships between variables need to be identified and model structures need to be defined. […] This is a very cumbersome, manual process, which takes weeks or even months to accurately define even just one marketing attribution model for one brand, given that companies usually have a broad product/brand portfolio or different customer segments to analyze. But there is good news! New AI algorithms and techniques cover those weaknesses, such as Gradient Boosted Machines (GBMs) and Shapley values that make marketing mix modelling approaches more accurate and scalable for a higher number of stores, brands, products, or customer segments.
近年来,对市场营销负责的呼声越来越高,以证明该地区的总投资是合理的。 此外,为了在不同的营销渠道之间分配预算,经理们一直在努力更好地了解他们对销售的贡献。 为了模拟营销对销售的影响,时间序列方法已被证明是成功的。 但是,Allergan的经验和学术研究表明,营销归因模型具有挑战性,因为需要识别变量之间的复杂关系并需要定义模型结构。 […]这是一个非常繁琐的手动过程,鉴于公司通常具有广泛的产品/品牌组合或不同的客户群,需要花费数周甚至数月的时间才能准确地为一个品牌定义一个营销归因模型。 但是有个好消息! 新的AI算法和技术弥补了这些弱点,例如梯度提升机(GBM)和Shapley值,这使得营销组合建模方法对于更多的商店,品牌,产品或客户群来说更加准确和可扩展。
Allergan Case — the promising journey of AI-driven marketing analytics:
Allergan Case — AI驱动的营销分析的美好旅程:
The Allergan team traditionally utilized GLM models for estimating the impact of promotion campaigns and to optimize the marketing mix of dozens of products across a variety of marketing channels. We started our AI-marketing journey two years ago together with H2O.ai to improve our marketing analytics. One of our recent challenges was to apply new machine learning algorithms to estimate the impact of a radio campaign for one of our products. For this project, both GLMs and H2O.ai’s Driverless AI were utilized and compared. […] We were very excited to see how new machine learning algorithms perform as we all know how much effort it is to define traditional linear marketing mix models and that they had a lot of problems with complex real-world cases.
传统上,Allergan团队使用GLM模型来评估促销活动的影响,并优化各种营销渠道中数十种产品的营销组合。 两年前,我们与H2O.ai一起开始了AI营销之旅,以改善我们的营销分析。 我们最近面临的挑战之一是应用新的机器学习算法来评估无线电活动对我们产品之一的影响。 对于此项目,GLM和H2O.ai的无人驾驶AI均得到了利用和比较。 […]我们很高兴看到新的机器学习算法如何执行,因为我们都知道定义传统的线性营销组合模型需要付出很大的努力,并且它们在复杂的实际案例中存在很多问题。
Read more about all challenges that can be overcome and the Allergan Case in the free white paper
在免费的白皮书中详细了解所有可以克服的挑战和Allergan案例
传统线性营销组合模型与新算法(Traditional Linear Marketing Mix Models vs. new Algorithms)
General Linear Models (GLMs) have been around since 1972 and have been used extensively in the area of marketing mix modelling as they allow for an additive approach (sales = baseline + TV spend + Radio spend + other channels). This, in combination with clear model specifications, provides analysts with the opportunity to identify incremental contributions of marketing channels to sales. However, new algorithms outperform linear models not just in accuracy but also in explainability by accounting for non-linearity between a predictor and dependent variable and interactions between predictor variables. Tree-based models in particular score high on all those characteristics mentioned above, and among them Gradient Boosted Machines (GBMs). Gradient boosting is a machine learning technique, typically based on decision trees, that uses an ensemble of weak prediction models. Those models usually show a higher accuracy in out of sample predictions than GLM and are more robust than single decision trees. This difference can lead to a significant business impact compared to linear Marketing Mix Models. The attribution of sales to marketing channels in GBMs can be done through a methodology called Shapley values. […]
通用线性模型(GLM)始于1972年,已被广泛用于市场营销组合建模领域,因为它们允许采用加性法(销售=基准+电视支出+广播支出+其他渠道)。 结合明确的模型规格,这为分析师提供了确定营销渠道对销售的增量贡献的机会。 但是,通过考虑预测变量和因变量之间的非线性以及预测变量之间的相互作用,新算法不仅在准确性上而且在可解释性方面均优于线性模型。 基于树的模型尤其在上述所有这些特征上得分很高,其中包括梯度提升机器(GBM)。 梯度提升是一种通常基于决策树的机器学习技术,它使用一组弱预测模型。 这些模型在样本外预测中通常显示出比GLM更高的准确性,并且比单个决策树更可靠。 与线性营销组合模型相比,这种差异可能会对业务产生重大影响。 可以通过一种称为Shapley值的方法将销售归因于GBM中的营销渠道。 […]
Allergan Case — the holy grail of marketing attribution analytics:
Allergan Case —营销归因分析的圣杯:
The concept of Shapley values combined with new machine learning models is intriguing — it promises the holy grail of marketing analytics. It combines highly accurate out-of-the box machine learning models with high explainability leading to accurate marketing mix attribution models. Historically, we used linear models and knew their weaknesses from an accuracy perspective, but they are easily explainable and therefore provided a great value in marketing attribution analytics. Hence, we usually accepted all of their weaknesses in accuracy and adjusted the models in multiple iterations to ensure that model outputs align with our business understanding.
Shapley价值观与新的机器学习模型相结合的概念很有趣-它有望成为营销分析的圣杯。 它结合了高度精确的现成机器学习模型和高度可解释性,从而形成了准确的营销组合归因模型。 从历史上看,我们使用线性模型并从准确性的角度了解它们的弱点,但是它们很容易解释,因此在营销归因分析中具有很大的价值。 因此,我们通常会接受他们在准确性方面的所有弱点,并在多次迭代中调整模型,以确保模型输出符合我们的业务理解。
Read more about the benefits of Shapley Values and GBMs compared to the traditional GLM approach in the free white paper
在免费的白皮书中详细了解Shapley值和GBM与传统GLM方法相比的优势
克服线性营销组合模型的缺点(Overcoming the weaknesses of Linear Marketing Mix Models)
Traditional linear time-series models have known weaknesses we are attempting to overcome with GBMs and Shapley values. First, the short-term impact of media on sales over several weeks can be low and is therefore very hard to estimate with linear models if the error of the model is higher than their impact.
传统的线性时间序列模型具有已知的弱点,我们正在尝试使用GBM和Shapley值克服这些弱点。 首先,媒体在几周内对销售的短期影响可能很小,因此如果模型的误差大于其影响,则很难使用线性模型进行估计。
Further challenges include the problem of group aggregations and the time to calibrate a model correctly […],non-linearity in market response functions (see Figure 1) […], variable interactions […], and asymmetries of market responses over time […].
进一步的挑战包括群体聚集问题和正确校正模型的时间[…],市场响应函数的非线性(请参见图1)[…],变量交互作用[…]和市场响应随时间的不对称性[ …]。
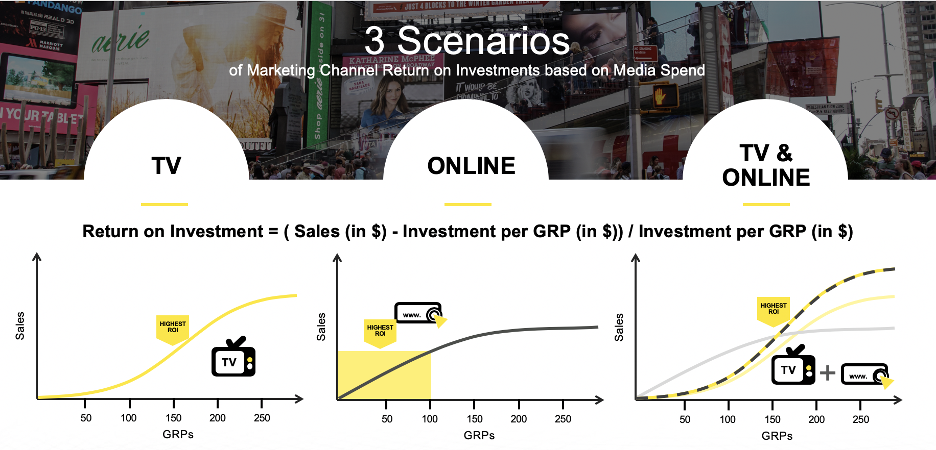
Allergan Case — the comparison between both approaches:
Allergan Case —两种方法之间的比较:
We wanted to compare the traditional linear marketing mix with the new machine learning based approach to experience the differences firsthand. We started off with our traditional linear marketing mix approach. Over several weeks we developed a fixed effect linear model in SAS and ran multiple iterations to ensure that model output aligns with our business understanding e.g. coefficients of promotions are expected to be positive and specific consumer control variables are expected to be significant. […] We applied H2O.ai’s Driverless AI to run a series of tree-based models (including GBMs) and extracted Shapley values via its Python client to calculate the contribution for each channel. We were able to do that within hours, not weeks.
我们希望将传统的线性营销组合与基于机器学习的新方法进行比较,以亲身体验这些差异。 我们从传统的线性营销组合方法开始。 在数周的时间里,我们在SAS中开发了固定效应线性模型,并进行了多次迭代,以确保模型输出与我们的业务理解保持一致,例如,促销系数预计为正,特定消费者控制变量预计为重要。 […]我们使用H2O.ai的无人驾驶AI来运行一系列基于树的模型(包括GBM),并通过其Python客户端提取Shapley值来计算每个通道的贡献。 我们能够在几小时内,而不是几周内做到这一点。
Read more about how to overcome the weaknesses of GLMs and Allergan’s outcome of the comparison of the approaches in the free white paper
在免费的白皮书中详细了解如何克服GLM的缺点以及Allergan的方法比较结果
Once an impact of a media channel has been identified via a linear model, it is usually assumed that this holds for the entire time sample, unless there are clearly identifiable structural breaks. However, even when there are no clear structural breaks, we know that market responsiveness may not be the same over time. For example, advertising effectiveness might decline over the life cycle of a product, seasonal differences of a media channel’s impact, the sales shock of an established brand after the introduction of a competing product or the own-price effects on media effectiveness (see Figure 2 below). […]
一旦通过线性模型确定了媒体渠道的影响,通常会假定在整个时间样本中这种影响都成立,除非存在明显可识别的结构性断裂。 但是,即使没有明确的结构性突破,我们也知道随着时间的流逝,市场的响应能力可能会有所不同。 例如,广告效果可能会在产品的生命周期内下降,媒体渠道影响的季节性差异,引入竞争性产品后老牌子的销售冲击或对媒体效果的自身价格影响(请参见图2)下面)。 […]
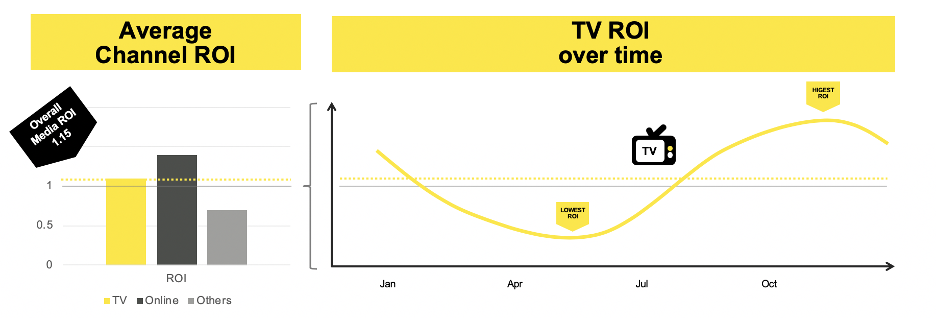
最佳预算分配(Optimal Budget Allocation)
The final goal of marketing mix modelling is to allocate budgets across channels, products, customer segments and brands. A common approach to allocate marketing budgets among marketing practitioners has usually been upon the combination of insights from more or less static Linear Marketing Models and market statistics of media spending’s of competitors to increase the share of voice for a company’s media campaigns. Unfortunately, this will not lead to the expected long-term effects and will only show a short-term impact on sales. […]
营销组合建模的最终目标是在渠道,产品,客户群和品牌之间分配预算。 在营销从业者之间分配营销预算的一种常用方法通常是将来自或多或少静态的线性营销模型的见解与竞争对手的媒体支出的市场统计数据相结合,以增加公司媒体活动的声音份额。 不幸的是,这不会导致预期的长期影响,而只会对销售产生短期影响。 […]
Read more about how apply the GBM marketing mix model for optimal budget allocation in the free white paper
在免费白皮书中详细了解如何将GBM营销组合模型应用于最佳预算分配
概要(Summary)
Budget Allocation has always been a complicated topic in the field of Marketing as it is a complex optimization problem taking into account not just a variety of marketing effects (product, price, place, promotion), context effects (such as trend, seasonality), market actions (competitor actions, new product launches, competitive price changes) and groups that might show different market response patterns (different brands, regions, customer segments). We hope that we could make a good case for the move to AI/ML by implementing GBMs and Shapley values in your marketing analytics to provide the unique opportunity to build more holistic marketing mix models. Your final models provide a production ready environment for a marketing application that can help marketers better understand budget allocation and help them to improve the impact of marketing spend.
预算分配一直以来都是 市场营销领域的复杂主题,因为它是一个复杂的优化问题,不仅要考虑各种营销影响(产品,价格,位置,促销),上下文影响(例如趋势,季节性),市场行为(竞争对手的行为,新产品发布,具有竞争力的价格变化)和可能显示不同市场响应模式的组(不同的品牌,地区,客户群)。 我们希望通过在您的营销分析中实施GBM和Shapley值,为向AI / ML过渡提供良好的案例,从而为构建更全面的营销组合模型提供独特的机会。 您的最终模型为营销应用程序提供了生产就绪的环境,可以帮助营销人员更好地了解预算分配并帮助他们改善营销支出的影响。
Allergan Case — the comparison between both approaches:
Allergan Case —两种方法之间的比较:
Marketing mix/attribution analytics has always been a highly complicated topic and brought value only with a high effort in data preparation, testing of single variable impacts and variable interactions, functional forms for important response curves, defining ad-stock effects and applying business knowledge to get to logical results. With all that effort, we could get to comparable results the new Machine Learning approach was providing in a shorter amount of time. […]
营销组合/属性分析一直是一个非常复杂的话题,只有在数据准备,测试单个变量影响和变量交互,重要响应曲线的功能形式,定义广告库存效果以及将业务知识应用于得到合乎逻辑的结果。 通过所有这些努力,我们可以在更短的时间内获得新的机器学习方法的可比结果。 […]
Read more about the outcome of Allergan’s pilot project in the free white paper
在免费白皮书中了解有关Allergan试点项目结果的更多信息
ai模型