深入浅出强化学习:原理入门
Artificial Intelligence (AI) has become a huge buzz word in the past 5 years or more, and more and more people are being clued up about Artificial Neural Networks that can be trained in two different ways, namely supervised learning and unsupervised learning. However, there is one more that doesn’t really fall under either of the two mentioned categories and this is called reinforcement learning.
在过去的5年或更长的时间里,人工智能(AI)已成为一个热门话题,越来越多的人开始关注可以通过两种不同方式进行训练的人工神经网络,即监督学习和非监督学习。 但是,还有另外一种并没有真正属于上述两种类别,这称为强化学习。
Reinforcement learning is generally used on already established neural network models to encourage specific behaviors to achieve more of a favored outcome. Reinforcement learning currently has been used as a buzz word, and in these cases, it just placed into a black box.
强化学习通常用于已经建立的神经网络模型上,以鼓励特定的行为获得更多的满意结果。 强化学习目前已被用作流行语,在这些情况下,它只是放在一个黑盒子中。
In this article, I want to give a surface level explanation of what reinforcement learning is, by opening this “black box” that has been thrown around and expected to do amazing things.
在本文中,我想通过打开这个“黑匣子”来对强化学习是一个表面层面的解释,该“黑匣子”被扔来扔去,并有望做奇妙的事情。
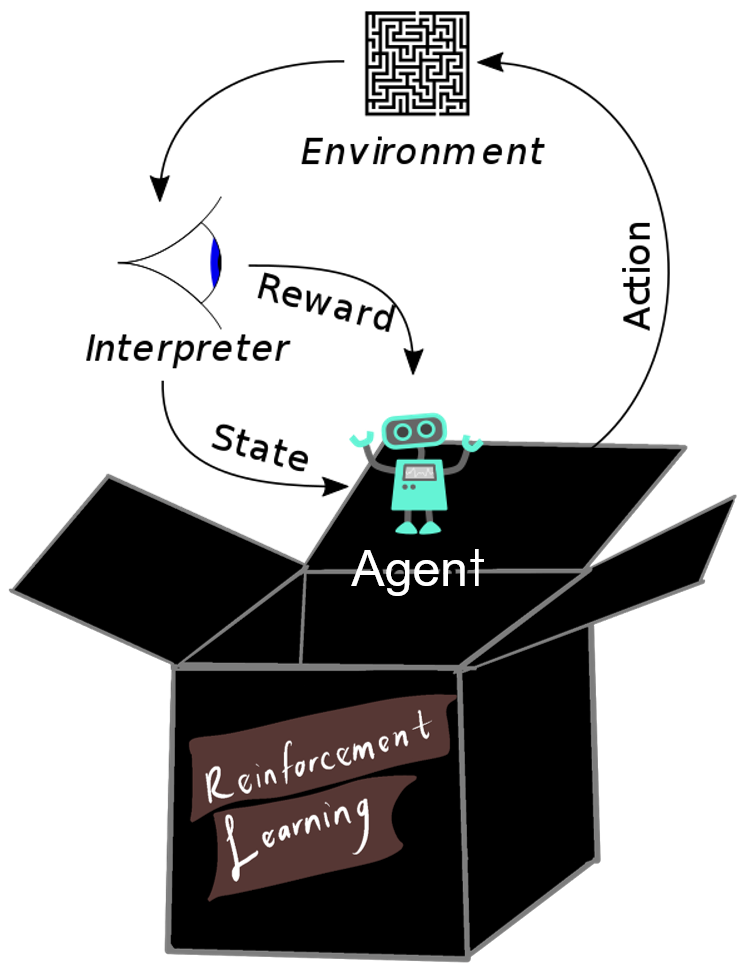