贝叶斯深度神经网络
Recently I came across an interesting Paper named, “Deep Ensembles: A Loss Landscape Perspective” by a Laxshminarayan et al.In this article, I will break down the paper, summarise it’s findings and delve into some of the techniques and strategies they used that will be useful for delving into understanding models and their learning process. It will also go over some possible extensions to the paper. You can also find my annotations on the paper down below.
最近,我碰到了Laxshminarayan等人写的一篇有趣的论文,名为“ 深度合奏:一种迷失的景观视角” 。在本文中,我将对该论文进行分解,总结其发现,并深入研究他们使用的一些技术和策略。有助于深入了解模型及其学习过程。 它还将介绍本文的一些可能扩展。 您也可以在下面的论文中找到我的注释。
理论 (The Theory)
The authors conjectured (correctly) that Deep Ensembles (an ensemble of Deep learning models) outperform Bayesian Neural Networks because “popular scalable variational Bayesian methods tend to focus on a single mode, whereas deep ensembles tend to explore diverse modes in function space.”
作者推测(正确),深度集成(深度学习模型的集合)优于贝叶斯神经网络,因为“流行的可扩展变分贝叶斯方法倾向于专注于单一模式,而深度集成则倾向于探索功能空间中的多种模式。”
In simple words, when running a Bayesian Network at a single initialization it will reach one of the peaks and stop. Deep ensembles will explore different modes, therefore reducing error when put in practice. In picture form:
简而言之,在一次初始化中运行贝叶斯网络时,它将到达其中一个峰值并停止。 深度合奏将探索不同的模式,因此在实际操作中会减少错误。 图片形式:
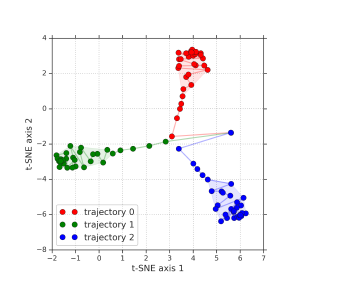
Depending on it’s hyperparameters, a single run of a bayesian network will find one of the paths (colors)and it’s mode. Therefore it won’t explore the set of parameters. On the other hand, a deep ensemble will explore all the paths, and therefore get a better understanding of the weight space (and solutions). To understand why this translates to better understanding consider the following illustration.
取决于它的超参数,一次运行贝叶斯网络将找到路径(颜色)及其模式之一。 因此,它不会探索参数集。 另一方面,一个深层次的合奏将探索所有路径,从而更好地理解权重空间(和解决方案)。 要了解为什么这可以更好地理解,请考虑以下插图。
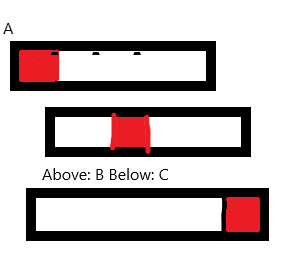