Inspired by Google DeepMind’s team, Shakir Mohamed, William Isaac, and Implikit’s founder Marie-Therese Png article, Decolonial AI, my experience with Data Science and readings, I’ll try to propose a production strategy that compensates the lack of scalable ethics in Data Science Systems and make it embedded since the beginning of the development, saving the cost of change later.
受Google DeepMind团队Shakir Mohamed,William Isaac和Implikit创始人Marie-Therese Png文章Decolonial AI的启发,我对数据科学和阅读的经验,我将尝试提出一种生产策略,以弥补数据中缺乏可扩展的道德规范自从开发之初就开始使用Science Systems并将其嵌入,从而节省了以后的更改成本。
问题 (The Problem)
The main problem that I’ll approach might be kind of obvious.
我要解决的主要问题可能很明显。
Data Science does not implement efficient and scalable Ethical guidelines. At least, yet.
数据科学没有实施有效且可扩展的道德准则。 至少呢。
But, in my opinion, it could be reformulated, as:
但是,我认为可以重新定义为:
Today, Data Science is not Customer Centric.
如今,数据科学已不再以客户为中心。
The reason, I'll detail along the article is:
原因,我将在本文中详细介绍:
Implicitly, our work might be motivated by solely on optimizing revenue, costs, human and non-human operational resources under the facade of enriching Customer Experience when we are launching Data-based products.
隐含地,我们的工作可能仅出于在启动基于数据的产品时丰富客户体验的基础上优化收入,成本,人力和非人力运营资源的动机。
This is as complicated to say as is it to tackle.
说起来和解决起来一样复杂。
But I'll explain it and argue along this article that with Architectural principles from Software Engineering, there might be a light at the end of the tunnel.
但是,我将对此进行解释,并在本文中坚持认为,根据软件工程的体系结构原理,隧道尽头可能会有曙光。
Without losing money (or our jobs, for the sake of productivity).
不亏本(或为了提高生产力而失业)。
上下文 (The Context)
We see we live, and it’s pretty clear.
我们看到我们生活了,这很清楚。
Data Science and Software Engineering novelties are bringing lots of innovations, opportunities, but also reinforcing social inequalities and dishonesty.
数据科学和软件工程的新颖性带来了许多创新,机遇,但同时也加剧了社会不平等和不诚实。
For example, thanks to the social network recommendation systems, radical skepticism is becoming almost a needed practice to consume information on the web.
例如,由于有了社交网络推荐系统,激进的怀疑论几乎成为了在网络上消费信息的一种必需做法。
And it shouldn’t be like this, because doing so it’s exhausting, and not everyone is willing to do it.
而且不应该这样,因为这样做很累,而且并不是每个人都愿意这样做。
If some of us do it, we are getting tired, and we are already tired of other consumption cycles, personal problems, and the current international healthcare and political crisis.
如果我们中的某些人这样做,我们会感到疲倦,而我们已经厌倦了其他消费周期,个人问题以及当前的国际医疗保健和政治危机。
Unfortunately, who endorsed this situation, specifically in the information domain?
不幸的是,谁支持这种情况,特别是在信息领域?
In my opinion, we, the Data practitioners.
我认为我们是数据从业者。
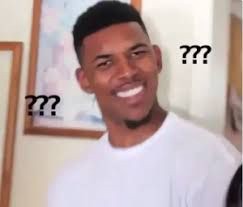
I agree, it’s not like we made it, but let’s be frank, we've let things get out of control.
我同意,这不像我们做到的那样,但是坦率地说,我们让事情失控了。
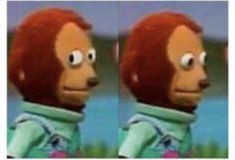
We are acting like Software Engineers once did in enterprises.
我们的行为就像软件工程师曾经在企业中所做的那样。
It really costed them to build stable and good practices, but eventually, it paid off. Usually, they are seen as independent workers, with their own practices and ethics. Practices and ethics that actually, we use almost all of them.
建立稳定和良好的实践确实使他们付出了代价,但最终却获得了回报。 通常,他们被视为具有自己的实践和道德准则的独立工人。 实际上,我们几乎都使用了实践和道德规范。
But, this independence does not follow for Data practitioners yet.
但是,数据从业者还没有遵循这种独立性。
We import many practices from Software Engineering, but our dependencies have very different behaviors since we deal with personal data artifacts.
我们从软件工程部门引进了许多实践,但是由于我们处理个人数据工件,因此我们的依赖项具有截然不同的行为。
And as I saw from other companies, interviews, talking to friends in the field, the production cycle train of thought ends up being "will it pay-off for the company or for my team?".
正如我从其他公司,访谈,与现场朋友交谈中看到的那样,生产周期的思路最终是“为公司还是为我的团队带来回报?”。
The thing about it is that we usually put the customer only in the beginning and at the end of the process.
关于它的事情是,我们通常只将客户放在流程的开始和结尾。
We should be thinking about them along all of the processes.
我们应该在所有过程中考虑它们。
For instance, the recent GPT-3, made by an enormous company like OpenAI, and I didn’t see any pieces of evidence of ethical practices in production. The model is too complex, too big to unbiased effectively.
例如,最近由像OpenAI这样的大公司生产的GPT-3,我没有看到任何有关生产中道德规范的证据。 该模型太复杂,太大而无法有效地进行偏置。
Possibly they’ve used explainability techniques in the model, but is this enough?
他们可能在模型中使用了可解释性技术,但这足够了吗?
Could this avoid GPT-3 producing sophisticated fake news?
这样可以避免GPT-3产生复杂的假新闻吗?
Can underdeveloped countries fight these effectively? Or will they be subjected to the will of an elite that will do according to their ethics? It is of the interest of countries at war “want” to discover if the news of each other is fake, or will they use fake news to fuel rage upon each other?
欠发达国家可以有效地与之抗争吗? 还是会服从按照道德规范行事的精英人士的意志? 发现“彼此”的消息是否是伪造的,还是它们会利用伪造的消息在彼此之间激怒,是否符合“想要”战争国家的利益?
Can an underdeveloped country make its own GPT-3 model compete with OpenAI?
欠发达国家能否使自己的GPT-3模型与OpenAI竞争?
My point is, that maybe we're not thinking enough about the implications of our models.
我的观点是,也许我们没有对模型的含义进行足够的思考。
And that while we continue to be educated by governments and their laws, we are not maturing the area enough.
而且,尽管我们继续受到政府及其法律的教育,但我们对该地区的成熟程度还不够。
Good professional ethics in the field should transcend our local domain problems, and we should start to effectively embed and advocate in our practice ethical concerns.
该领域的良好职业道德应该超越我们当地的问题,我们应该开始有效地将道德 问题纳入和倡导实践。
For starters, just because we use Data from the user or because we talk with them from time to time, it doesn't mean we really care, or that we are thinking about the user, being Customer Centric.
对于初学者来说,仅仅是因为我们使用了来自用户的数据,或者是因为我们不时与他们交谈,这并不意味着我们真的在乎,或者我们正在考虑以用户为中心。
Maybe that's a mixture of Product Centrism and Direct Marketing, but not Customer-Centric.
也许这是产品中心主义和直销的混合,但不是以客户为中心的。
For me, being Customer Centric it's when the Customer comes to our mind in the whole production cycle, embeds care, wishes success for them, and not because we use them for production.
对我而言,以客户为中心是客户在整个生产周期中想到我们,嵌入关怀,为他们祝愿成功的原因,而不是因为我们将其用于生产。
We should really learn the impact, positive or negative of our work, and not make ethics a rhetorical tool for positive, humane marketing.
我们应该真正了解我们工作的正面或负面影响,而不是将道德规范作为积极,人道营销的修辞工具。
我们正在成为技术专家吗? (Are we becoming technocrats?)
And this rhetoric might be endorsed by the predominant technocrat perspective in the field.
这种言论可能被该领域主要的技术专家观点所认可。
While we excessively value the technical side of Data Science, pure Data as our guide, modeling complex Machine Learning models, we are not being serious about our social responsibilities.
尽管我们过分重视数据科学的技术方面,以纯数据为指导,为复杂的机器学习模型建模,但我们并不认真对待我们的社会责任。
Many of us suppose our work is neutral, but as I see it:
我们许多人认为我们的工作是中立的,但据我所知:
Data = People.
数据=人员。
And not thinking like this it seems to me to be a backward, especially when we're establishing ethical directives. Ethics starts to look like it's a detail of our system, a marketing tool.
而且我不这样想,这似乎是一种落后,特别是在我们建立道德规范时。 道德开始看起来像是我们系统的一种细节,一种营销工具。
Do you know that Christmas ornament? That one we put in the ending? Looks like what we're making of ethics.
你知道那个圣诞节装饰品吗? 我们把那个放在结尾吗? 看起来像我们在讲道德。
But, shouldn't it be?
但是,不是吗?
I don't think so.
我不这么认为。
And to showcase the lack of neutrality of our work, how power is under every relationship, I'll try to summarize my point with two subjects commonly seen as neutral in society, that relates very closely in Data Science, both Science and Language.
为了说明我们的工作缺乏中立性,以及在每种关系下力量如何发挥作用,我将尝试总结两个在社会中通常被视为中立的主题,这两个主题在数据科学中与科学和语言密切相关。
首先,科学。 (First, Science.)
Like any other institution, is moved by the engine of interests and desires. There are those who make decisions of what is relevant or not, of what gets published or not based on variable criteria.
像任何其他机构一样,它是由利益和欲望的动力所驱动的。 有些人根据可变的标准来决定是否相关,是否发布哪些内容。
Kevin C. Elliott and Daniel J. McKaughan described well at the Philosophy of Science paper “Non-epistemic Values and the Multiple Goals of Science”.
凯文·埃利奥特(Kevin C. Elliott)和丹尼尔·麦考恩(Daniel J. McKaughan)在《科学哲学》论文“非流行性价值观和科学的多重目标”中作了很好的描述。
In summary, they argue that non-epistemic values (those not related to knowledge itself) also direct science growth, not only pure and quality knowledge since they depend on someone to approve the definition of what is “ purity” and “ quality”.
总之,他们认为非流行性价值观(那些与知识本身无关的价值观)也指导科学的发展,不仅是纯粹的知识和高质量的知识,因为它们依赖于某人来认可“纯度”和“质量”的定义。
第二,语言。 (Second, language.)
Another example is the mathematical language. If we consider mathematics a language, modeling of phenomena, there is a filter of information as in any other model and group of people who speak it.
另一个例子是数学语言。 如果我们认为数学是一种语言,一种现象的建模,那么就像任何其他模型和说话的人一样,信息过滤器也是如此。
We could ask:
我们可以问:
Who usually practice mathematics?
谁通常练习数学?
What’s the ratio of black woman and white males in math academia? LGBTQI+?
在学术界,黑人女性和白人男性的比例是多少? LGBTQI +?
Segmenting by country, how is it in the USA? In Brazil? In Venezuela? In Argentina? In China?
按国家细分,在美国如何? 在巴西? 在委内瑞拉? 在阿根廷? 在中国?
Another dimension of language is regional:
语言的另一个方面是区域性的:
What are the proportion of mathematical Portuguese articles in science that have more than 1.000 citations in relation to English?
相对于英语,被引用超过1.000的科学数学葡萄牙语文章占什么比例?
Is English that “universal”? Or is “dominant”?
英语是“通用”吗? 还是“显性”?
If we assume that English is “universal”, what does that mean for approximately 95% of the Brazillians that doesn't speak it?
如果我们假设英语是“通用”的,那么对于大约95%不会说英语的巴西人意味着什么 ?
Are they inferior? Or they lack opportunity and infrastructure?
他们是自卑的吗? 还是他们缺乏机会和基础设施?
As we question, and question, power structures start to unveil, even for language itself.
当我们质疑时,甚至对于语言本身,权力结构也开始显现。
In the context of Data Science - sure - the poor, uninformed, minorities in power can produce Data Science.
在数据科学的背景下-当然-权力低下,知情的少数群体可以产生数据科学。
But what are the odds that they will make their own best practices for their context? Do they have sustainable infrastructure to practice?
但是,他们会根据自己的情况制定最佳实践的可能性有多大? 他们有可实践的可持续基础设施吗?
Or will they follow international guidelines, that probably doesn’t think about them? Implement solutions in their context that might be more damaging than positive in the long run?
还是他们会遵循可能不考虑它们的国际准则? 在他们的环境中实施解决方案,从长远来看可能比积极的解决方案更具破坏性?
For me, it's pretty clear that:
对我来说,很明显:
Data Science is far from neutral.
数据科学远非中立。
And this should be something we need to act upon if we are serious about it.
如果我们认真对待,这应该是我们需要采取的行动。
If we continue to act and think only based on technical valuation and conception, on pure data, we will inevitably end up excluding others, excluding minorities of our workflow, and producing biased products and experience.
如果我们继续仅基于技术评估和构想采取行动并思考,仅基于纯数据,我们将不可避免地最终将其他人排除在外,排除工作流程中的少数人,并产生有偏见的产品和经验。
That's why Ethics and Customer-Centric philosophy are heavily important for a sustainable Data Science practice.
这就是为什么道德和以客户为中心的哲学对于可持续的数据科学实践至关重要的原因。
For me, this is maintained today because of two factors:
对我而言,由于两个因素,今天仍保持这种状态:
- The way the Data practices were built around Operational Research in the context of companies, and how it didn't address the Customer-Centric model; 在公司背景下围绕运营研究构建数据实践的方式,以及它如何不解决以客户为中心的模型;
- Because of how we are implementing our system in Agile practice today. 由于我们今天如何在敏捷实践中实施我们的系统。
1º原因:“数据科学”有何目的? (1º Reason: “Data Science” for what purpose?)
There is a possibility that we are heirs of problems not solved by another data-based field, Operational Research (OR).
我们有可能成为其他基于数据的领域运筹学(OR)无法解决的问题的继承人。
For those who don’t know, since the II World War, Operational Research was the main Data-based technique being used, focused on optimizing resource allocations to win the war.
对于那些不知道的人,自第二次世界大战以来,运筹学是使用的主要基于数据的技术,专注于优化资源分配以赢得战争。
Maximizing and minimizing resources for some specific goal, or as we might call, the objective function.
为某些特定目标或目标功能最大化或最小化资源。
The philosophy of optimal production, performance independent of the circumstances, was really attractive then and later in the '60s.
最佳生产的哲学,与环境无关的性能,在那时和60年代后期确实很有吸引力。
Not a surprise that it became a success. Since then, it became a powerful tool for optimizing cost-benefit relationships in enterprise production until today.
它成功了就不足为奇了。 从那时起,直到今天,它已成为在企业生产中优化成本-收益关系的有力工具。
Usually, an Operational Research model structures itself in three attributes:
通常,运筹学模型将自身构造为三个属性:
- The decision variables, or the resource variables that we will use for obtaining our objectives; 决策变量或我们将用于实现目标的资源变量;
- Objective functions, usually a function of the decision variables that we want to minimize or maximize; 目标函数,通常是我们要最小化或最大化的决策变量的函数;
- Restrictions, that will make the contour of the solution space of our problem. 限制,将使我们的问题的解决空间成为轮廓。
There are companies solutions like ILOG CPLEX from IBM, Gurobi Solvers, these apply specific methods for each kind of problem using Dual-Simplex, Interior Points Methods, and others to obtain the optimal solution in optimal time.
有一些公司的解决方案,例如IBM的ILOG CPLEX,Gurobi Solvers,这些解决方案使用Dual-Simplex,Interior Points方法等针对每种问题应用特定方法,以在最佳时间内获得最佳解决方案。
The OR workflow, extremely simplified, goes like this:
极简化的OR工作流程如下:
(please OR practitioners, don't kill me)
(请或从业者,别杀了我)
First, you model the problem. Like we want the optimal share for certain users in a Revenue Sharing model.
首先,您对问题进行建模。 就像我们希望在“收入共享”模型中为某些用户获得最佳份额一样。
Second, specify the decision variables for attaining optimality.
其次,指定决策变量以获得最优性。
Third, define the restrictions of the model based on enterprise resources.
第三,根据企业资源定义模型的限制。
Write the model in a solver, and press enter :)
将模型写入求解器,然后按Enter :)
In practice, is this easy as it looks?
实际上,这看起来容易吗?
No, far from easy. It takes time to make an efficient enterprise solution based on MILP ( Mixed-Integer Linear Programming ), but it also depends on the problem. I just needed to summarize so I don’t end up writing a full Epic.
不,绝非易事。 制定基于MILP(混合整数线性规划)的高效企业解决方案需要花费时间,但是这也取决于问题。 我只需要总结一下,这样我就不会写完整的Epic。
And well, I don’t know what you think, but this process certainly is not Customer-Centric for me.
而且,我不知道您的想法,但是对于我来说,此过程当然不是以客户为中心的。
100% Product, Capital, Enterprise Centric.
100%产品,资本,以企业为中心。
And this was the core of Operational Research.
这是运筹学的核心 。
But how could we make the modeling process more Customer-Centric?
但是我们如何才能使建模过程更加以客户为中心呢?
One solution could be enforcing restrictions that consider human health, age, time spent producing, mental conditions. All of these can be modeled mathematically, we just need to make it part of the development of the solution.
一种解决方案可能是实施限制措施,考虑人类健康,年龄,生产时间,精神状况。 所有这些都可以用数学方式建模,我们只需要使其成为解决方案开发的一部分即可。
When we apply OR to factories, enterprise productivity optimization, we could consider the humans and their necessities for well-being in the restrictions.
当我们将OR应用于工厂,企业生产力优化时,我们可以在限制条件中考虑人员及其对幸福感的必要性。
But usually, it's not.
但通常不是。
In practice, usually Operational Researchers deal with data as pure resources.
实际上,运营研究人员通常将数据视为纯资源。
And Data Scientists that deal with customers should see data as living behavior.
与客户打交道的数据科学家应该将数据视为生活行为。
But not coincidentally, they both end up with the same scope today.
但并非巧合的是,它们今天最终都具有相同的范围。
Optimizing, scaling processes, getting the state-of-the-art. But where is the Customer at this process?
优化,扩展流程,获取最新技术。 但是在此过程中客户在哪里?
It seems to me that the way we deal with data today is that if it were only resources, and not behaviors. In the end we're kind of reproducing the Operational Research way of thinking data.
在我看来,我们今天处理数据的方式是,如果它只是资源而不是行为。 最后,我们将重现运营研究的思维数据方式。
We're thinking about speed, optimizing metrics.
我们正在考虑速度,优化指标。
We're being agile, but in the wrong sense.
我们正在敏捷,但是在错误的意义上。
Usually, there are two common misinterpretations of the Agile production model:
通常,对敏捷生产模型有两种常见的误解:
1.速度: (1. Speed:)
Agile translates to continuous iteration, evolutionary design, and it does not mean necessarily to produce things fast.
敏捷转化为连续迭代,进化设计,并不意味着一定要快速生产。
When we think of Data Science as something purely technical, achieving full speed and metrics optimization should be the pinnacle of our art. But as we’ve discussed, it’s not.
当我们将数据科学视为纯粹的技术时,实现全速和指标优化应该是我们艺术的顶峰。 但是,正如我们所讨论的,事实并非如此。
When we join this Agile misinterpretation with seeing Data Science as something purely technical, good professional ethics with customers are usually a "nice to have", when it should be upfront.
当我们将敏捷性误解与将数据科学视为纯粹的技术结合起来时,与客户保持良好的职业道德通常是“必不可少的”,应该先行一步。
2.缺乏长期规划: (2. The absence of long-term planning:)
Iterations, but how small should them be?
迭代,但是应该多小呢?
What's the scope so that we don't over-engineer or we stop losing track of what does really delivers value with professional ethics?
在什么范围内我们可以不过度设计,或者我们不再失去对职业道德真正带来价值的追踪?
That might be something that is lacking in today's Engineering practices in Data Science, an Architectural perspective. And might be the secret to reduce the cost of implementing ethical principles in our Data Platform.
从架构的角度来看,这可能是当今数据科学的工程实践中缺少的东西。 并且可能是降低在我们的数据平台中实施道德原则的成本的秘密。
2º原因:我们的敏捷性不那么敏捷 (2º Reason: Our Agile is not that agile)
Since the beginning, Agile and eXtreme Programming philosophies advocated for incremental and continuous development. Solving a problem when it emerges, YAGNI and KISS.
从一开始,敏捷和极限编程理念就倡导渐进和持续的开发。 YAGNI和KISS解决出现的问题。
It's productive, but they might be a problem when a system has no Architectural long-term guidelines.
它很有生产力,但是当系统没有体系结构长期指南时,它们可能会成为问题。
And in Data Science in particular, we have very few good architecture references.
尤其是在数据科学领域,我们很少有优秀的体系结构参考。
Don’t we have lot's of Data Pipelines and Machine Learning Processes?
我们是否没有大量的数据管道和机器学习流程?
The way I see it, these are operational pipelines, not Architectural projects.
我的看法是,这些是可操作的管道,而不是建筑项目。
Agile architectures should be built incrementally, with small and complete iterations, to maximize value delivery and maintain close contact with customers.
敏捷体系结构应逐步构建,并进行小而完整的迭代,以最大程度地提高价值交付并保持与客户的密切联系。
They call it an evolutionary design, and I think it makes total sense.
他们称之为进化设计,我认为这是完全合理的。
A good Software Architect (or Data Architect) should have a horizon of the system in mind as soon as possible because the Architecture will guide him through the constraints of the system.
优秀的软件架构师(或数据架构师)应尽快考虑系统的前景,因为架构将引导他克服系统的约束。
If we don’t have this, we will postpone invisible problems, that are not usually measurable at the beginning, that will end up showing up with harsh costs.
如果没有这些,我们将推迟通常在一开始就无法衡量的无形的问题,这些问题最终将导致高昂的代价。
In our case, we've seen with the latest events how Data-based Platforms influenced social behavior in the Coronavirus Crisis, the latest elections won based on Fake News and automated bots and other consequences.
在我们的案例中,我们通过最新事件了解了基于数据的平台如何影响冠状病毒危机中的社会行为,基于虚假新闻和自动漫游器赢得的最新选举以及其他后果。
These invisible problems, are getting big. But even so, until now enterprises don't want or can't approach effectively those problems, because of cost-of-change constraints.
这些无形的问题正在变得越来越大。 但是即使如此,由于变更成本的限制,直到现在,企业还是不希望或无法有效解决这些问题。
So
所以
解决方案:了解客户,然后围绕他构建系统 (A Solution: Understand the customer, then build the System around him)
A possible solution might be based at the center of a Software Architecture:
可能的解决方案可能基于软件体系结构的中心:
The Domain Layer.
域层。
For those who already studied the Clean Architecture model of software systems, Hexagonal, Ports and Adapters, etc, know that the most stable part of the system is the domain part of it.
对于那些已经研究过软件体系结构,六角形,端口和适配器等清洁结构模型的人来说,知道系统的最稳定部分是系统领域的一部分。
The business rules, that we programmers, Data Scientists follow, are ruled by the customer experience, problems, and desires that define use cases.
我们的程序员,数据科学家遵循的业务规则由定义用例的客户体验,问题和需求所决定。
The rest of the system is developed around it.
系统的其余部分围绕它开发。
If the Customer is at the center of the system domain, it means that ethics should be in the Domain region of our Architecture also.
如果客户是系统领域的中心,则意味着道德也应该在我们架构的领域范围内。
That might be the road for a good, sustainable solution in today’s production model.
在当今的生产模型中,这可能是一个好的,可持续的解决方案的道路。
Because when we delegate Customer Centrism, Ethics as a detail — as Robert Martin says in Clean Architecture — we are making the system less and less dependent on it, and that means we are implicitly saying:
因为当我们委托客户中心主义时,道德作为一个细节,正如罗伯特·马丁(Robert Martin)在“清洁建筑”中所说的那样,我们正在使系统对它的依赖越来越少,这意味着我们暗中说:
This does not matter now, we can delegate.
现在没关系,我们可以委托。
And this is actually wrong from a professional perspective.
从专业的角度来看,这实际上是错误的。
By telling customers we want to make their experience the best with their data, but are not we thinking about them in the process, the social implications of our work, using their information and behavior solely for our own production and profit optimization, we are lying.
通过告诉客户我们希望利用他们的数据使他们的体验达到最佳,但是我们不是在过程中,工作的社会含义,仅将他们的信息和行为用于我们自己的生产和利润优化时就在考虑他们,这是在说谎。
If we don’t make Ethics part of the core of our development cycle, we won’t experience really concrete our proposal to the customer. We are just using them for profit, for their data assets, and they benefit somehow while they use our product.
如果我们不将道德规范纳入开发周期的核心,我们将不会真正向客户提出具体的建议。 我们只是将它们用于牟利,获取数据资产,并且在使用我们的产品时会从某种程度上受益。
Somewhere in a close future, it's quite possible that probably someone will brag about the systems build like this and say:
在不久的将来的某个地方,很可能有人会吹嘘这样的系统构建并说:
How did they not think about this? I’ll have to fix this mess somehow…
他们怎么没想到呢? 我将不得不以某种方式解决此问题……
New Data usage legislations, regulations will come, and we could be already prepared for it.
新的数据使用立法,法规将出台,我们可能已经为此做好了准备。
This is somehow familiar with what already happened with Software Engineering, in production environment.
这对生产环境中软件工程已经发生的事情有些熟悉。
When programmers were postponing detecting bugs, implementing tests, building monolithic systems that had a bizarre cost of change if they needed to refactor, fix bugs, concise and scalable implementation of business rules in production.
当程序员推迟检测错误,实施测试,构建整体式系统时,如果他们需要重构,修复错误,在业务中实现业务规则的简洁和可扩展实施,那么变更成本将非常高。
Until certain point in history, they used to delegate the responsibility, and someone else, who was going to fix it that mess, would feel like this:
直到历史上的某个特定时刻,他们通常将职责委派给他人,而要修复这一混乱状况的其他人会感觉像这样:
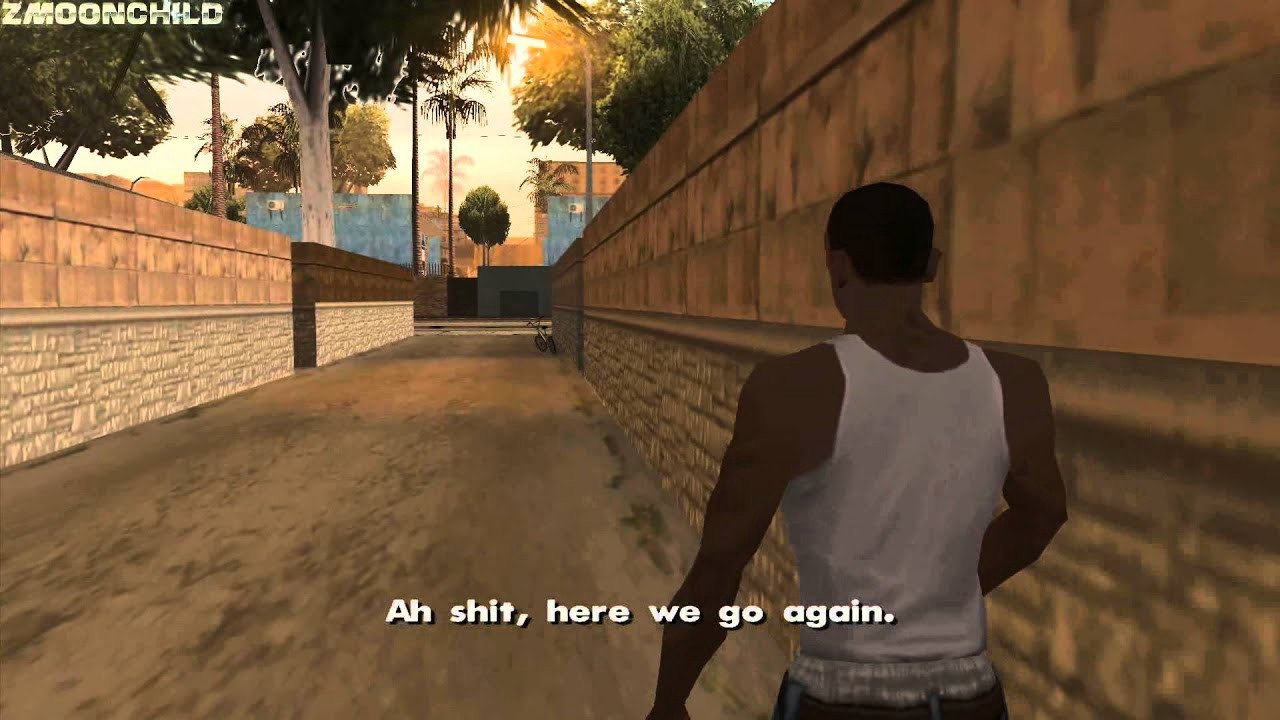
And because of these problems, specially in production environment, Test-Driven Development was formalized and is evangelized until today.
由于存在这些问题,特别是在生产环境中,“测试驱动开发”被正式化并推广到今天。
They embedded in their practice professional ethics and anticipation, that if well implemented didn't augmented the software production time.
他们将实践中的职业道德和期望嵌入到实践中,即如果实施得当,不会增加软件生产时间。
You don't ship production code without testing.
未经测试,您不会交付生产代码。
Those who shameful about their past could say:
那些对自己的过去感到羞耻的人可以说:
We didn’t knew that. It was not a good practice then…
我们不知道。 那不是一个好习惯……
But I think probably you knew somehow, as much as we Data Scientists know.
但是我认为您可能知道了什么,正如我们数据科学家所知道的那样。
It's like we fear the speed of the production engine. And I get it, it’s quite scary and big.
就像我们担心生产引擎的速度一样。 而且我知道,它非常可怕而且很大。
It can take your job, salary if you don’t go along with it, if you're not as fast as they think it should be, so you postpone invisible but important things like tests and ethical data usage, feature engineering.
它会占用您的工作,薪水(如果您不配合的话),薪水不如他们认为的那么快,因此您会推迟看不见但重要的事情,例如测试和道德数据使用,功能工程。
Eventually, this will not hold. It's not sustainable, the same thing is happening again in Data Science, and the consequences are showing up very quickly.
最终,这将不成立。 这是不可持续的,数据科学领域又发生了同样的事情,其后果正在Swift显现。
But, if we embed the ethical logic in the core of the system, the Domain Layer, in our core practices, we enforce ethical values not only in our Data Platform, but in our daily practice.
但是 ,如果在我们的核心实践中将道德逻辑嵌入到系统的核心(领域层)中,我们不仅在我们的数据平台中,而且在我们的日常实践中都践行道德价值观。
And that could be our strategy.
那可能是我们的策略。
为什么以及如何运作? (Why and how should this work?)
The architectural reason is that, ideally, the core of the system is as abstract as stable.
架构上的原因是,理想情况下,系统的核心既要抽象又要稳定。
That means that most of the modules depend on business rules, in our case, all data products depends on the Customer Problem, Use Cases, and Business Rules.
这意味着大多数模块都取决于业务规则,在我们的情况下,所有数据产品都取决于客户问题,用例和业务规则。
If we include Customer Ethics in this domain, we protect it and make it almost obligatory.
如果我们将客户道德规范纳入此领域,则我们将对其加以保护并使其几乎成为强制性。
So in summary, all you need to do is to include a Customer Ethics in the Domain Layer. Then, we'll have an Ethical Data Science Platform.
因此,总而言之,您需要做的就是在域层中加入客户道德规范。 然后,我们将有一个道德数据科学平台。
Once you make the Ethics part of the Domain Layer, it’s not a detail anymore, there is no escape, because it will be part of the most stable part of the system.
一旦将Ethics设置为Domain Layer的一部分,就不再是一个细节,也就不会逃脱,因为它将成为系统最稳定的部分。
But how will I unify them?
但是,我将如何统一它们?
Create an interface called Ethics, CustomerEthics implements it, and compose with Customer? What else?
创建一个名为Ethics的接口,CustomerEthics实施该接口,并与Customer组成? 还有什么?
You could do that, but I don’t see the need for this yet, maybe it’s a solution I didn’t think about and might be good in the future.
您可以做到这一点,但我认为还没有必要,也许这是我没有考虑过的解决方案,并且将来可能会很好。
For now, I thought that you need to construct the system thinking about building a culture around the user.
就目前而言,我认为您需要构建考虑围绕用户的文化的系统。
Gather knowledge from the customer, directly and indirectly, understand their pain, and understand how power inequalities might affect them.
直接和间接地从客户那里收集知识,了解他们的痛苦,并了解电力不平等如何影响他们。
Understand the socioeconomic profile of your users, the proportions, and design along with product priorities, making ethics part of the Business Rules.
了解用户的社会经济概况,比例,设计以及产品优先级,从而将道德规范纳入业务规则。
This should enforce production train of thought to include best ethical practices in the Data system.
这应该加强生产思路,以在数据系统中包括最佳道德规范。
Displacing the Data Platform development from 100% technical, functional pure data to 50% technical and 50% user profiling, experience (or something like that), the designing starts to change, and the way the team thinks will start to change also.
将数据平台的开发从100%的技术,功能纯数据转移到50%的技术和50%的用户配置文件,经验(或类似的东西),设计开始发生变化,并且团队认为的方式也将开始发生变化。
Make it in the Domain, and the natural evolution of the system should take care of it, with good Data Architecting and Agile made it right.
在Domain中实现它,并且系统的自然演进应该照顾好它,良好的Data Architect和Agile正确地做到了。
That’s how a Data Science System could start to evolve naturally with Ethics, without having to implement big changes later, you already made them in the first place.
这样一来,数据科学系统便可以随着Ethics自然地发展,而不必稍后进行重大更改,而您已经在第一时间进行了更改。
For references and different use cases, there is a good catalog that might help you ideate and define specific strategies based on this architectural approach on the Decolonial AI article.
对于参考和不同的用例,有一个不错的目录,可以帮助您根据Decolonial AI文章中的这种体系结构方法来构思和定义特定策略。
As enterprises domain vary a lot, understanding the possible biases made upon users could be interesting to change the Data culture in your culture also, making Data-Driven philosophy more mature.
由于企业领域千差万别,因此了解用户上可能存在的偏见也可能会改变您所在文化中的数据文化,从而使数据驱动的哲学更加成熟。
Probably uniting with a UX team might be very effective for this, since they are specialists in User Stories, new strategies might come along in your company, since Ethical guidelines will differ from Use Cases to Use Cases.
也许与UX团队团结起来可能会非常有效,因为他们是用户故事的专家,因此,公司的道德准则会因用例而异,因此新的策略可能会出现在您的公司中。
结论 (Conclusions)
And that’s it, after a long reading, I hope I made some contributions to the discussion, with my point of view of how can we implement an efficient Data Science System without suffering later with Ethical concerns.
就这样,经过长时间的阅读,我希望我能为讨论做出一些贡献,并提出自己的观点,即如何实施高效的数据科学系统而又不会再遭受道德问题的困扰。
The objective is to put Ethics in the Domain Layer of an Architectural perspective of the system and build it around it with responsible Agile development.
目的是将道德放在系统的体系结构透视图的领域层中,并通过负责任的敏捷开发围绕它进行构建。
Gather with UX researchers, your PM’s and Data fellows to understand the profile of the user, because your tools interact directly with them.
与您的UX研究人员,您的PM和数据研究员一起,了解用户的概况,因为您的工具直接与他们互动。
That's why we should
这就是为什么我们应该
Make Data products Customer-Centric.
使数据产品以客户为中心。
Data is unstable because it has a bijective relationship with the Customer, that's why we should invest deeply understanding them.
数据不稳定,因为它与客户之间存在双向关系,这就是为什么我们应该投入更多精力来理解它们。
The more we realize this, I think the more we will mature our practices and how we are seen professionally.
我们越了解这一点,我认为我们越会成熟我们的实践以及如何在专业上被看待。
If you agree, disagree, think there are any historical, logical misconceptions on the text, want to contribute somehow, I’ll be glad to discuss, and you can e-mail me in victor.souza@passeidireto.com, or talk with me in my LinkedIn page.
如果您同意,不同意,认为文本有任何历史上的,逻辑上的误解,想以某种方式做出贡献,我将很高兴进行讨论,您可以通过victor.souza@passeidireto.com给我发送电子邮件,或与我在我的LinkedIn页面上 。
[1] Mohamed, S., Png, M. & Isaac, W. Decolonial AI: Decolonial Theory as Sociotechnical Foresight in Artificial Intelligence (2020), Philos. Technol.
[1] Mohamed,S.,Png,M.和Isaac,W. Decolonial AI:作为人工智能的社会技术预见的Decolonial理论 (2020年), Philos。 技术。
[2] Kevin C. Elliott and Daniel J. McKaughan, Nonepistemic Values and the Multiple Goals of Science (2014), Philosophy of Science 81:1, 1–21
[2]凯文·埃利奥特(Kevin C. Elliott)和丹尼尔·麦考恩(Daniel J. McKaughan),《非精神价值论和科学的多重目标》 (2014年),《科学哲学》 81:1,1–21
[3] Robert C. Martin, Clean Architecture: A Craftsman’s Guide to Software Structure and Design (2017), Prentice Hall
[3] Robert C. Martin,《 清洁建筑:软件结构和设计的工匠指南》 (2017年),Prentice Hall