神经网络激活函数对数函数
Activation function, as the name suggests, decides whether a neuron should be activated or not based on the addition of a bias with the weighted sum of inputs. Hence, it is a very significant component of Deep Learning, as they in a way determine the output of models. The activation function has to be efficient so the model can scale along the increase in the number of neurons.
顾名思义,激活函数根据输入的加权和加上偏倚来决定是否激活神经元。 因此,它是深度学习的重要组成部分,因为它们以某种方式确定了模型的输出。 激活功能必须高效,因此模型可以随着神经元数量的增加而缩放。
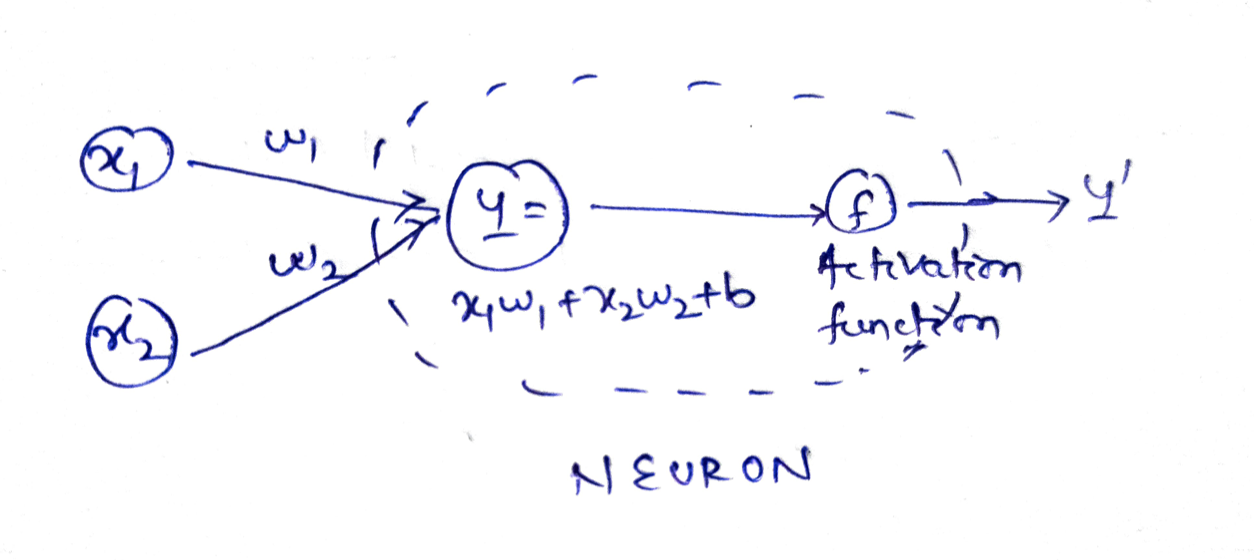
To be precise, the activation function decides how much information of the input relevant for the next stage.
确切地说,激活功能决定了与下一阶段相关的输入信息量。
For example, suppose x1 and x2 are two inputs with w1 and w2 their respective weights to the neuron. The output Y = activation_function(y).
例如,假设x1和x2是两个输入,其中w1和w2分别占神经元的权重。 输出Y = activation_function(y)。
Here, y = x1.w1 + x2.w2 + b i.e. weighted sum of inputs and bias.
y = x1.w1 + x2.w2 + b,即输入和偏置的加权和。
Activation functions are mainly of 3 types. We will analyze the curves, pros and cons of each here. The input we work on will be an arithmetic progression in [-10, 10] with a constant difference of 0.1
激活功能主要有3种。 我们将在这里分析每个曲线的优缺点。 我们正在处理的输入将是[-10,10]的算术级数,常数差为0.1
x = tf.Variable(tf.range(-10, 10, 0.1), dtype=tf.float32)
二元步 (Binary step)
A binary step function is a threshold-based activation function. If the input value is above or below a certain threshold, the neuron is activated and sends exactly the same signal to the next layer.
二进制步进函数是基于阈值的激活函数。 如果输入值高于或低于某个阈值,则神经元被激活并将完全相同的信号发送到下一层。
#Binary Step Activation
def binary_step(x):
return np.array([1 if each > 0 else 0 for each in list(x.numpy())])
do_plot(x.numpy(), binary_step(x), 'Binary Step')
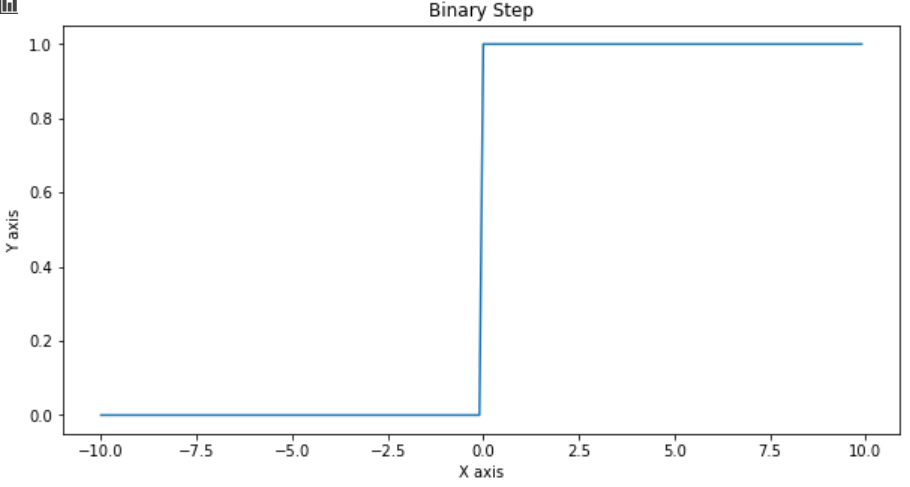
The binary step is not used mostly because of two reasons. Firstly it allows only 2 outputs that don’t work for multi-class problems. Also, it doesn’t have a derivative.
不使用二进制步骤主要是因为两个原因。 首先,它仅允许2个对多类问题不起作用的输出。 另外,它没有派生词。
线性的 (Linear)
As the name suggests, the output is a linear function of the input i.e. y = cx
顾名思义,输出是输入的线性函数,即y = cx
#Linear Activation
def linear_activation(x):
c = 0.1
return c*x.numpy()
do_plot(x.numpy(), linear_activation(x), 'Linear Activation')
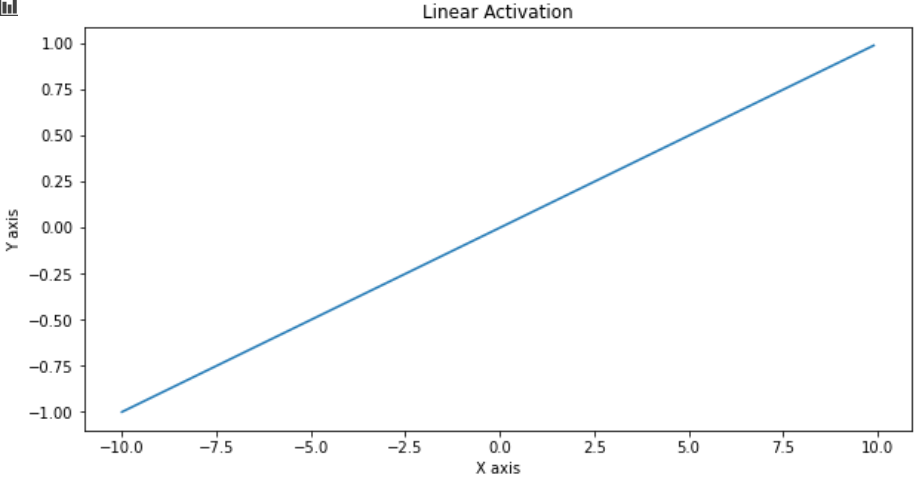
The linear activation function is also not used is neural networks because of two main reasons.
由于两个主要原因,线性神经网络也不使用线性激活函数。
Firstly, with this activation at multiple layers, the last output just becomes a linear function of the input. That defeats the purpose of multiple neurons and layers.
首先,通过多层激活,最后一个输出刚好成为输入的线性函数。 这破坏了多个神经元和层的目的。
Secondly, as y = cx, the derivative becomes dy/dx = c, which is a constant. So it can’t be used in backpropagation to train neural networks.
其次,当y = cx时,导数变为dy / dx = c,这是一个常数。 因此,它不能用于反向传播训练神经网络。
非线性的 (Non-linear)
Non-linear activation functions are used everywhere in neural networks as they capture the complex pattern in data due to the non-linearity nature and support back-propagation as they have a derivative function.
非线性激活函数在神经网络中随处可见,因为它们具有非线性特性,因此可以捕获数据中的复杂模式,并且由于具有微分函数而支持反向传播。
Here we discuss 5 popularly used activation functions.
在这里,我们讨论5种常用的激活函数。
Sigmoid
乙状结肠
Sigmoid function squashes the output between 0 to 1 and the function looks like sigmoid(x) = 1 / (1 + e^(−x))
Sigmoid函数将输出压缩在0到1之间,并且该函数看起来像sigmoid(x)= 1 /(1 + e ^(-x))
y = tf.nn.sigmoid(x)
do_plot(x.numpy(), y.numpy(), 'Sigmoid Activation')
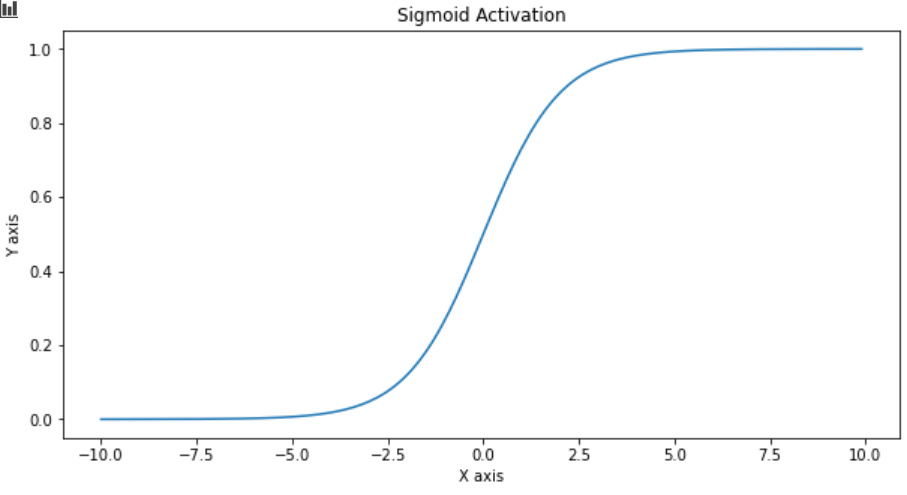
The major advantage of this function is that its gradient is smooth and output always lies between 0 to 1.
此功能的主要优点是其渐变平滑,输出始终在0到1之间。
It has a few cons like, the output always lies between 0 to 1 which is not suitable for multi-class problems. With multiple layers and neurons, the training gets slower as it is computationally expensive.
它有一些缺点,例如输出始终位于0到1之间,这不适合多类问题。 由于具有多层和神经元,因此训练速度较慢,因为计算量大。
with tf.GradientTape() as t:
y = tf.nn.sigmoid(x)
do_plot(x.numpy(), t.gradient(y, x).numpy(), 'Grad of Sigmoid')
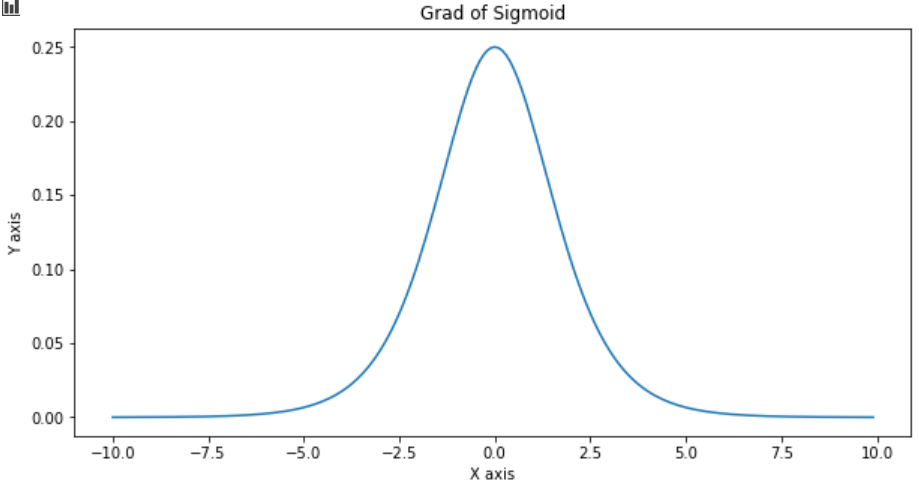
Also as seen in the gradient graph, it suffers from vanishing gradient problem. The input changes from -10 to -5 and 5 to 10, but the gradient output doesn’t change.
同样从梯度图中可以看出,它遭受了梯度消失的困扰。 输入从-10更改为-5,从5更改为10,但梯度输出不变。
2. Tanh
2. 谭
Tanh activation function is more like sigmoid but it squashes output between -1 to 1. The function looks like tanh(x) =(1-e^(-2x))/(1+e^(2x))
Tanh激活函数更像是Sigmoid,但是在-1到1之间压缩输出。该函数看起来像tanh(x)=(1-e ^(-2x))/(1 + e ^(2x))
y = tf.nn.tanh(x)
do_plot(x.numpy(), y.numpy(), 'Tanh Activation')
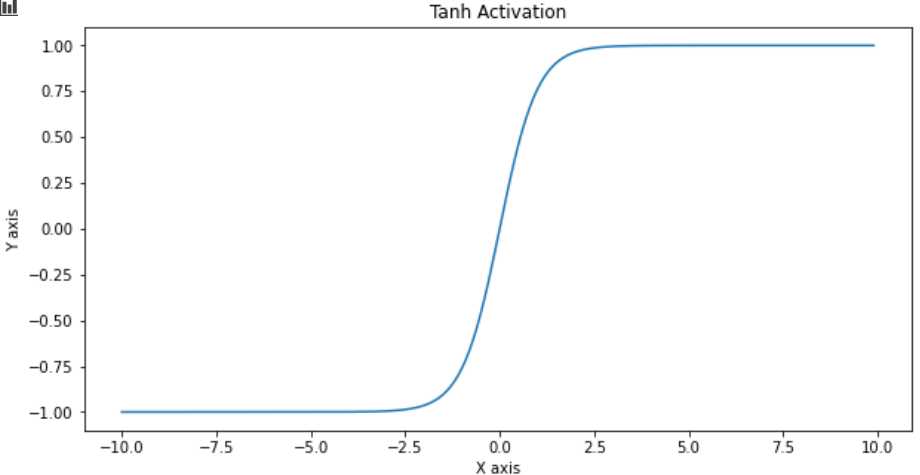
In addition to the advantages of the sigmoid function, its output is 0 centered.
除了S形函数的优点外,其输出以0为中心。
With multiple layers and neurons, the training gets slower as it is also computationally expensive.
由于具有多层和神经元,因此训练变得较慢,因为它在计算上也很昂贵。
with tf.GradientTape() as t:
y = tf.nn.tanh(x)
do_plot(x.numpy(), t.gradient(y, x).numpy(), 'Grad of Tanh')
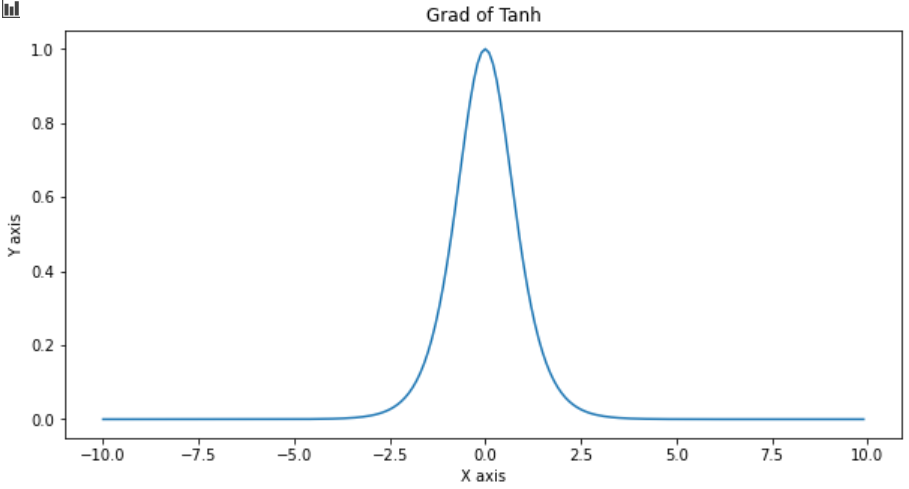
As seen in the gradient graph, it also suffers from a vanishing gradient problem. Here the input gets out of [-2.5, 2.5], but the gradient output doesn’t change no matter how much the input changes.
从梯度图中可以看出,它也面临着消失的梯度问题。 此处输入脱离[-2.5,2.5],但无论输入变化多少,梯度输出都不会改变。
3. ReLU
3. ReLU
ReLU or Rectified Linear Unit either passes the information further or completely blocks it. The function looks like relu(x) = max(0, x)
ReLU或整流线性单元会进一步传递信息或将其完全阻止。 该函数看起来像relu(x)= max(0,x)
y = tf.nn.relu(x)
do_plot(x.numpy(), y.numpy(), 'ReLU Activation')
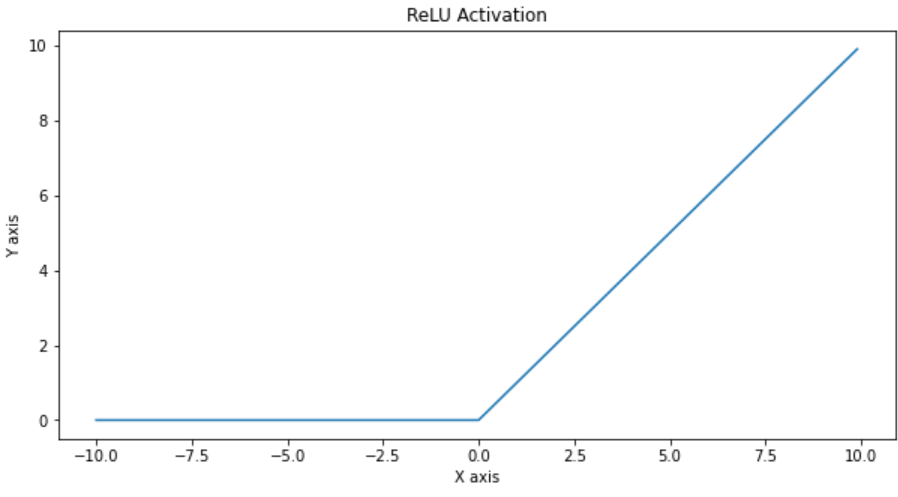
It is most popular due to its simplicity and non-linearity. Its derivatives are particularly well behaved: either they vanish or they just let the argument through.
由于其简单性和非线性,它是最受欢迎的。 它的派生词表现得特别好:要么消失,要么只是让争论通过。
with tf.GradientTape() as t:
y = tf.nn.relu(x)
do_plot(x.numpy(), t.gradient(y, x).numpy(), 'Grad of ReLU')
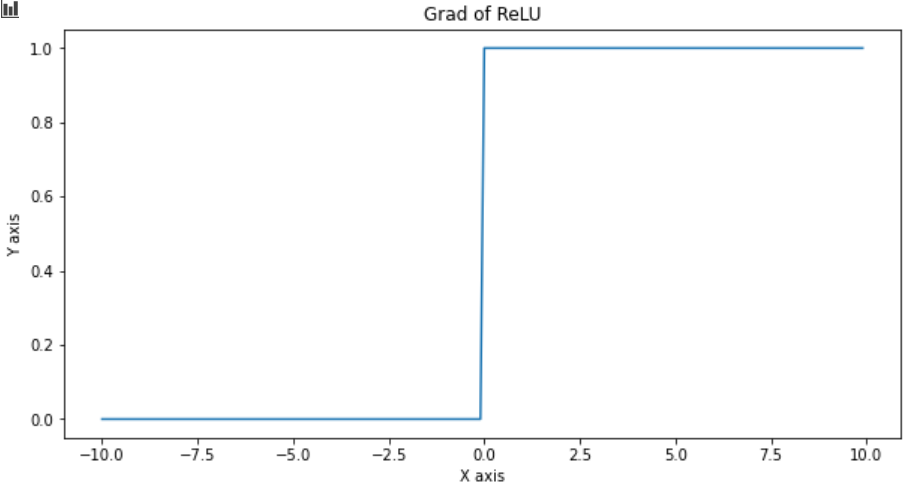
One disadvantage it has is that it doesn’t retain negative inputs thus if inputs are negative, it doesn’t learn anything. That is called the dying ReLU problem.
它的一个缺点是它不会保留负输入,因此,如果输入为负,它不会学到任何东西。 那就是垂死的ReLU问题。
4. Softmax
4. Softmax
Softmax activation function gives output in terms of probability and the number of outputs is equal to the number of inputs. The function looks like,
Softmax激活函数根据概率给出输出,并且输出数量等于输入数量。 该函数看起来像
softmax(xi) = xi / sum(xj)
softmax(xi)= xi /和(xj)
x1 = tf.Variable(tf.range(-1, 1, .5), dtype=tf.float32)
y = tf.nn.softmax(x1)
The major advantage of this activation function is it gives multiple outputs that make it popularly used in the output layer of a neural network. It makes it easier to classify multiple categories.
该激活函数的主要优点是它提供了多个输出,使其广泛用于神经网络的输出层。 这样可以更轻松地对多个类别进行分类。
The main limitation of this algorithm is that it won’t work if data is not linearly separable. Another limitation is that it does not support null rejection, so you need to train the algorithm with a specific null class if you need one.
该算法的主要局限性在于,如果数据不可线性分离,则该算法将不起作用。 另一个限制是它不支持空值拒绝,因此如果需要一个特定的空值类,则需要对算法进行训练。
5. Swish
5. 挥舞
Google Brain Team has proposed this activation function, named Swish. The function looks like swish(x) = x.sigmoid(x)
Google Brain团队提出了名为Swish的激活功能。 该函数看起来像swish(x)= x.sigmoid(x)
According to their paper, it performs better than ReLU with a similar level of computational efficiency.
根据他们的论文 ,在类似的计算效率水平上 ,它的性能优于ReLU。
y = tf.nn.swish(x)
do_plot(x.numpy(), y.numpy(), 'Swish Activation')
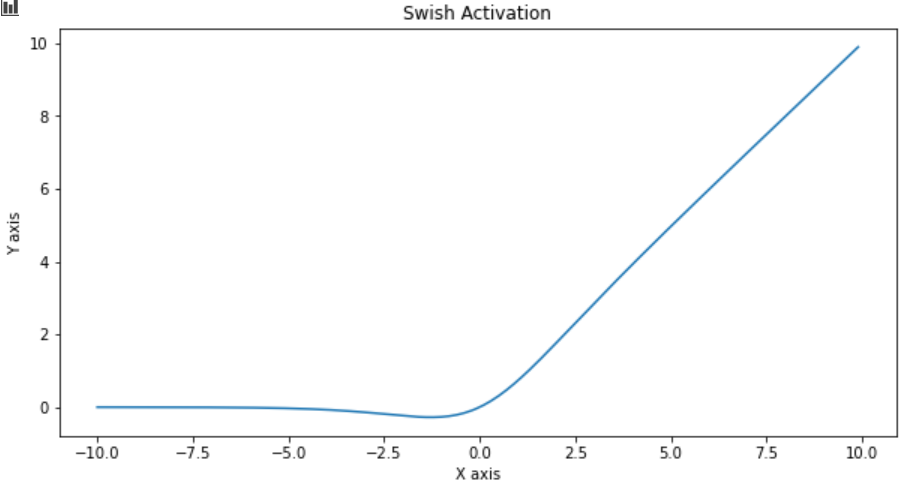
One reason swish might be performing better than ReLU is it addresses the dying ReLU issue as shown in the below graph.
下图显示swish可能比ReLU更好的原因之一是它解决了垂死的ReLU问题,如下图所示。
with tf.GradientTape() as t:
y = tf.nn.swish(x)
do_plot(x.numpy(), t.gradient(y, x).numpy(), 'Grad of Swish')
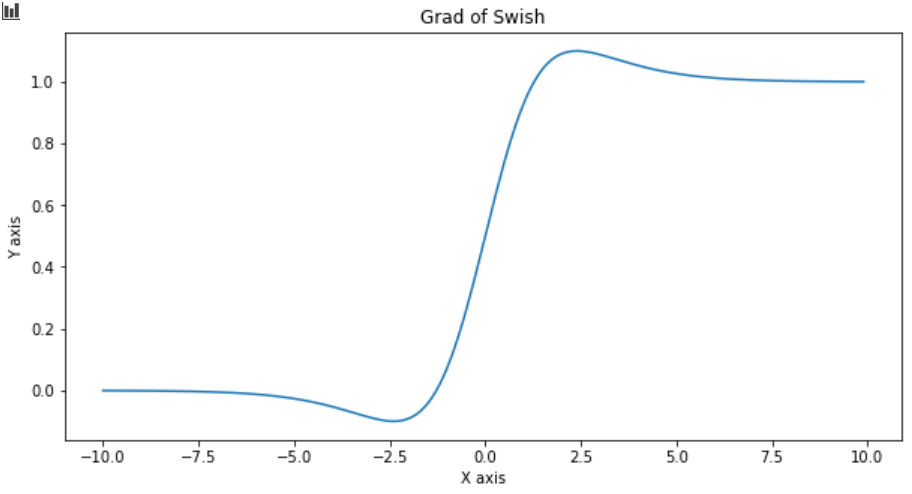
On an additional note, ReLU has got other variances of its type also which are quite popular like leaky ReLU, parametric ReLU, etc.
另外需要注意的是,ReLU还具有其他类型的差异,如泄漏ReLU,参数化ReLU等,它们也很受欢迎。
翻译自: https://medium.com/swlh/activation-functions-in-neural-network-eb0ab4bb493
神经网络激活函数对数函数