站长之家权重查询 API 接口在网上已经很多且大都封装成了 API 供别人调用。支持前台跨域请求,以GET/POST方式提交即可。站长之家权重查询 API 接口可以查询百度权重、360权重、神马权重等,你可以选择调用本站的接口,也可以利用本站的 API 接口封装成自己的接口。
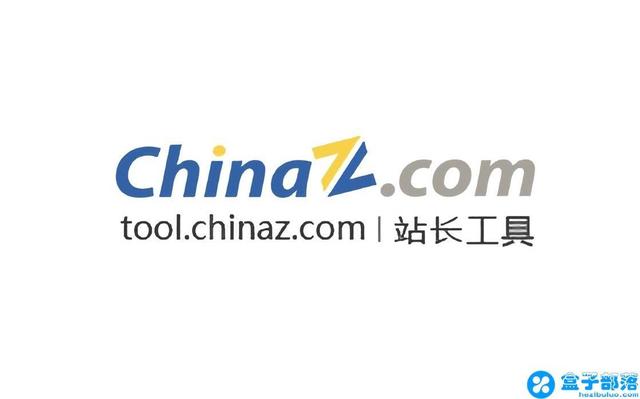
#站长之家权重查询API请求方式 #
Method: GET/POST
# 请求地址 #
https://api.ooopn.com/rank/chinaz/api.php
# 请求参数 #
url //要查询的域名,不带http/https(必填)
img //bdpc-bdm-360pc-360m-sm,返回权重图片(选填)
# 返回参数 #
code //状态码,200-查询成功,201-没填写域名
domain //要查询的域名
bdpc //百度权重
bdm //百度移动权重
360pc //360权重
360m //360移动权重
sm //神马权重
msg //错误状态
# 调用示例 #
https://api.ooopn.com/rank/chinaz/api.php?url=www.qq.com
https://api.ooopn.com/rank/chinaz/api.php?url=www.qq.com&img=bdpc
# 返回数据 #
{
"code": "200