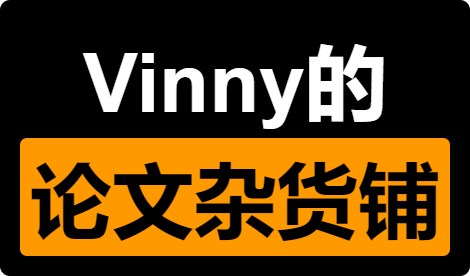
Attention-Based Capsule Networks with Dynamic Routing for Relation Extraction
Attention-Based Capsule Networks with Dynamic Routing for Relation Extractionwww.aclweb.org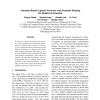
本文发表在EMNLP-2018上
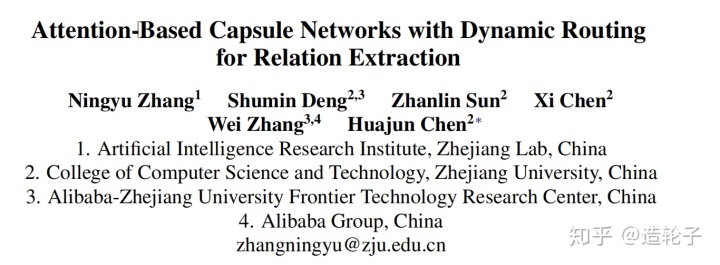
还有一篇类似的论文是AAAI-2019的,没看过的可以参考下:
造轮子:利用注意力胶囊网络进行多标签关系抽取zhuanlan.zhihu.com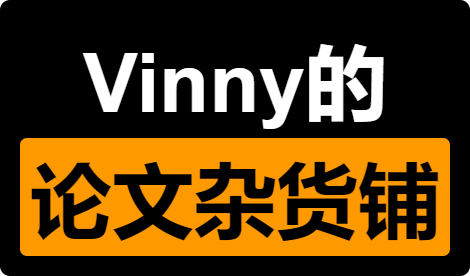
abstract
In this paper, we explore the capsule networks used for relation extraction in a multi-instance multi-label learning framework and propose a novel neural approach based on capsule networks with attention mechanisms
从摘要来看这篇文章也是用来解决multi-label关系分类,运用了capsule network。并且也有一个attention mechanism。这里就要看看和之前写的那篇有什么不同之处。他的attention也是在dynamic routing那里做的改进嘛?
不过有一点需要搞清楚的是,既然做的是multi-label并不是distant supervision,那么为什么要用到multi-instance呢?
motivation
之前的工作存在以下不足:
- First, the existing models focus on, and heavily rely on, the quality of instance representation.Using a vector to represent a sentence is limited because languages are delicate and complex.
- Second, CNN subsampling fails to retain the precise spatial relationships between higher-level parts.The structural relationships such as the positions in sentences are valuable.
- Besides, existing aggregation operations summarizing the sentence meaning into a fixed size vector such as max or average pooling are lack of guidance by task information.
其实总的来说,motivation跟19年的还是比较相似的。
methods
We regard the aggregation as a routing problem of how to deliver the messages from source nodes to target nodes
这一点与19年的那篇处理overlapped、discrete relation feature那里真是“异曲同工之妙啊”
Furthermore, the capsule networks convert the multi-label classification problem into a multiple binary classification problem.
这里其实是capsule network所固有的,最后一层的胶囊数量就是relation的数量,胶囊的模长就是一个label的置信度。所以对多个label 分类,就像对多个胶囊做二分类。设定阈值,当大于阈值的时候,就认定这个relation label是存在的。
网络的结构图如下所示:
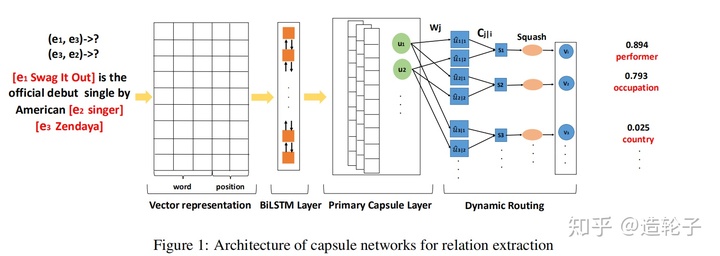
基本与19年AAAI的结构完全类似。
这篇文章没有对capsule network的margin loss进行改进

动态路由部分也是采用原始的
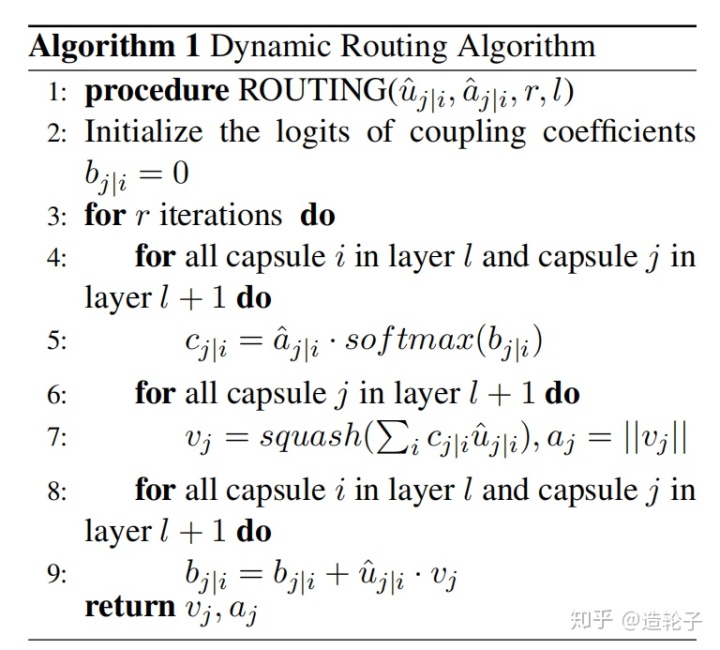
他这里的多实体关系抽取,指的是在一个sentence里面有多个实体对,但是每个实体对只有一个relation

所以在预测阶段,就有两种情况:
- 对于单实体对,直接计算最长的胶囊即可。
- 对于多实体对, For multiple entity pairs relation extraction, we choose relations with top two probability meanwhile bigger than the threshold
experiment
实验针对两个数据集,一个是NYT10用来做单实体对RE;一个是Wikidata dataset 用来做多实体对RE。
不过他这里的多关系,并不是指的一个实体对的多关系。所以这个部分也是这个工作和19年AAAI最主要的区别。
下面是在NYT10上面的PR曲线,这里实验的设置应该是直接参考了15年zeng的那篇PCNN论文。
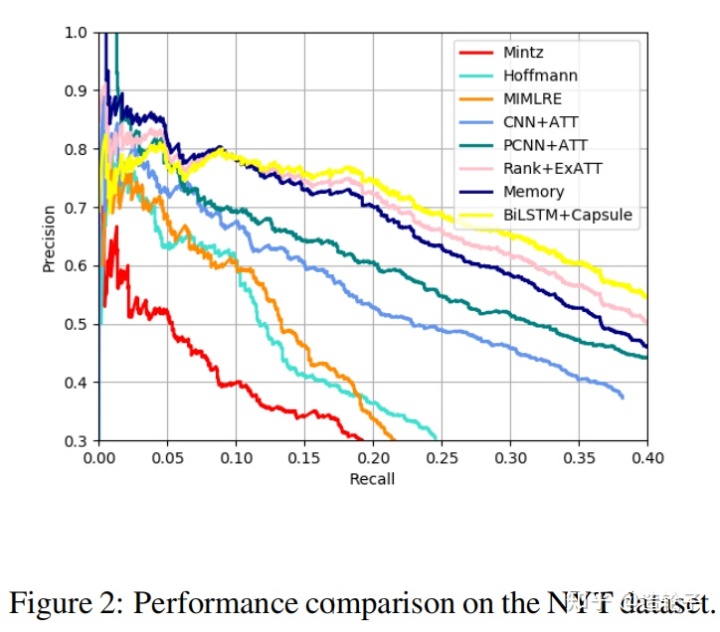
后面的实验就是在Wikidata dataset 上面测了下,又做了一个简单的消融实验。
这篇论文最大的亮点,差不多就是套用了capsule network来做RE。