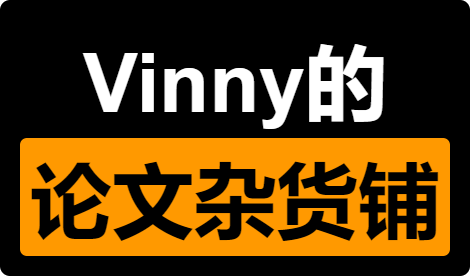
Long-tail Relation Extraction via Knowledge Graph Embeddings and Graph Convolution Networks
https://www.aclweb.org/anthology/N19-1306/www.aclweb.org论文发表在NAACL-2019上
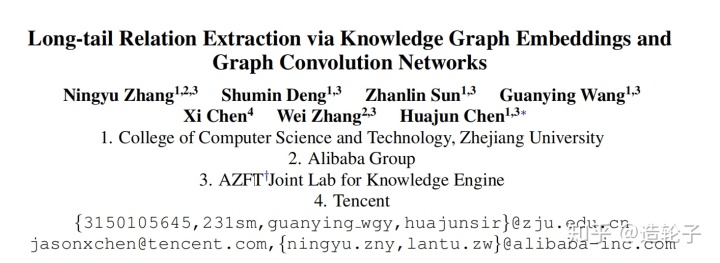
这篇论文的遗憾就是没能要来源码啊~
我觉得现阶段,大部分远程监督工作主要解决的问题就是两个
- 样本噪声问题,因为采用自动对齐知识图谱标注,所以有些样本的label就是错误的。感觉解决噪声的工作相对样本不平衡的要多。
- 样本不平衡或者是long tail问题,比如NYT数据集中,有很多NA标签的样本,还有很多关系样本出现的次数特别少。
这篇论文比较好的地方:
- 在利用关系的依赖这个信息上,能够利用外部知识来“pretrain” relation embedding,等于加入了先验信息。
- 层级attention的构造上面,也进行了改进,在层级representation的拼接上面也加了attention。
- 实验做的很充分。
abstract
We propose a distance supervised relation extraction approach for long-tailed, imbalanced data which is prevalent in real-world settings.
很显然,看名字也知道是解决long tail问题的。
Inspired by the rich semantic correlations between classes at the long tail and those at the head, we take advantage of the knowledge from data-rich classes at the head of the distribution to boost the performance of the data-poor classes at the tail.
也是利用关系之间的依赖来解决问题的,就是想用相关的data-rich classes,来增强long tail classes。
就主要看看这篇工作是怎么引用和对比下面这篇文章的。
造轮子:coarse to fine & 分层REzhuanlan.zhihu.com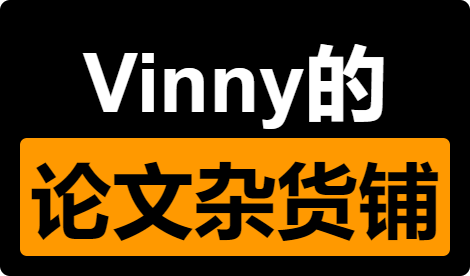
First, we propose to leverage implicit