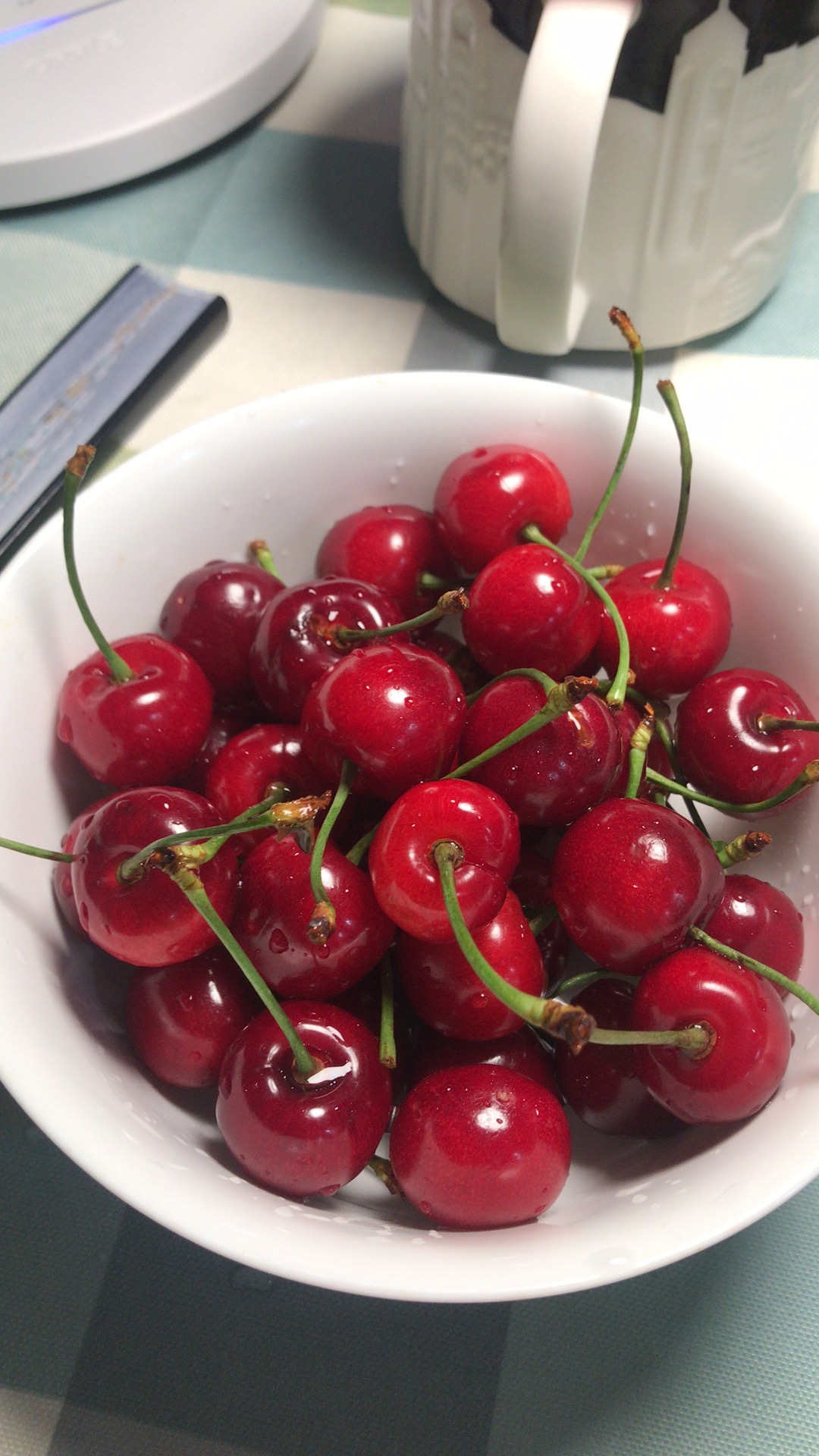
参考: https:// blog.csdn.net/ykf173/ar ticle/details/86747592 http://www. cips-cl.org/static/anth ology/CCL-2015/CCL-15-019.pdf
最近需要根据句子对齐,给中英句对进行打分,因此看了一下相关的开源项目。
Gale和Church的句对齐算法
参考: https:// zhuanlan.zhihu.com/p/59 071889 https:// github.com/NLPpupil/gal e_and_church_align https://www. aclweb.org/anthology/J9 3-1004.pdf
Gale和Church在1993年提出了一个基于长度进行句对齐的算法,并在附录里公开了C源代码。这篇论文相当经典,以至于之后的关于句对齐的论文大多数要引用它。论文的题目是 A Program for Aligning Sentences in Bilingual Corpora。这个方法适合欧美语系,思想就是根据句子的长度来比较的。比较出名的hunalign工具是基于galechurch想法写的,并做了改进。Hunalign可以用于十几种语句的对齐,但是很遗憾,中文不太使用,但是也不是完全不适用,只是效果不太好。LF就是根据它做了一些小的改进,对其效果还可以。
对齐分两步。第一步是段落对其,第二步是在段落内部进行句对齐。段落对齐重要,不过简单,问题在于段落内部的句对齐。所以本文只解析已知段落对齐,怎样在段落内进行句对齐。首先定义几个概念,所有论文中出现的符号都对应定义里的符号。
- 句子 一个短的字符串。
- 段落 语文里的自然段。分为源语言L1的段落和目标语言L2的段落,或称原文段落和译文段落。段落由一个序列的连续句子组成。
- 片段 一个序列的连续的句子,是段落的子集。对应论文中的portion of text。
- 片段对 原文片段和译文片段组成的对。

分别对应片段对中原文部分和译文部分的字符总数。

假设源语言中的一个字符在目标语言中对应的字符数是一个随机变量,且该随机变量服从正态分布

。(如何估计可参考:https://www.zhihu.com/question/39080163)

论文中定义为
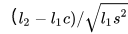
。每一个片段对都有自己的一个

。
- 对齐模式 或称匹配模式,描述一个句块对由几个原文句子和几个译文句子组成。比如1-2表示一个原文句子翻译成两个译文句子的对齐模式。
- match 对齐模式的概率分布。
- 距离(distance measure) 衡量片段对两个片段之间的距离。距离度量是对

的估计。当一个片段对确定后,我们就知道它的mathc和

。距离越大,此片段对对齐的概率越小。
- 片段对序列 一个序列的片段对,这些片段对的原文部分的集合是原文段落的一个划分,译文部分的集合是译文段落的一个划分。
- 距离和 距离和是片段对序列中所有片段对的
- 对齐序列 距离和最小的片段对序列。
对齐算法的输入是某一对相互对齐的段落,输出是对齐序列。接下来就变成了动态规划问题,类似最小编辑距离。片段对序列那么多,哪个是对齐序列呢?如果用穷举法,计算量太大,显然不现实。换个角度想,假设我们已经知道了对齐序列,用

表示该对齐序列的距离和,其中

是原文段落最后一个句子的index,

是译文段落最后一个句子的index。对齐序列的距离和可以表示成最后一个片段对的距离加上去掉最后一个片段对的剩下的片段对序列的距离和(可以认为对齐序列的子序列也是对齐序列)。最后一个片段对有六种对齐模式,所以要对每种模式分情况讨论,选择结果最小的那个。动态规划的递归式就是这么来的。
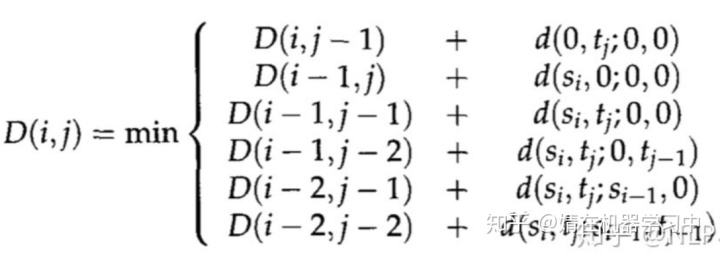
递归式的基础情况D(0,0)=0,通过递归式,我们可以求出对齐序列的距离和,在求距离和的过程中,我们顺便记录了对齐轨迹,也就是顺便求出了对齐序列。这就是算法的主干思想。下面就是它的主要代码:
import math
import scipy.stats
# 先使用统计的方法计算出对齐模式的概率分布
match = {(1, 2): 0.023114355231143552,
(1, 3): 0.0012165450121654502,
(2, 2): 0.006082725060827251,
(3, 1): 0.0006082725060827251,
(1, 1): 0.9422141119221411,
(2, 1): 0.0267639902676399}
# 源语言的一个字符对应于目标语言的字符数(正太分布)的均值
c = 1.467
# 源语言的一个字符对应于目标语言的字符数(正太分布)的方差
s2 = 6.315
def prob_delta(delta):
return scipy.stats.norm(0,1).cdf(delta)
def length(sentence):
punt_list = ',.!?:;~,。!?:;~”“《》'
sentence = sentence
return sum(1 for char in sentence if char not in punt_list)
def distance(partition1,partition2,match_prob):
l1 = sum(map(length,partition1))
l2 = sum(map(length,partition2))
try:
delta = (l2-l1*c)/math.sqrt(l1*s2)
except ZeroDivisionError:
return float('inf')
prob_delta_given_match = 2*(1 - prob_delta(abs(delta)))
try:
return - math.log(prob_delta_given_match) - math.log(match_prob)
except ValueError:
return float('inf')
def align(para1,para2):
"""
输入两个句子序列,生成句对
句对是倒序的,从段落结尾开始向开头对齐
"""
align_trace = {}
for i in range(len(para1) + 1):
for j in range(len(para2) + 1):
if i == j == 0:
align_trace[0, 0] = (0, 0, 0)
else:
align_trace[i,j] = (float('inf'),0,0)
for (di, dj), match_prob in match.items():
if i-di>=0 and j-dj>=0:
align_trace[i,j] = min(align_trace[i,j],(align_trace[i-di, j-dj][0] + distance(para1[i-di:i],para2[j-dj:j],match_prob), di, dj))
i, j = len(para1), len(para2)
while True:
(c, di, dj) = align_trace[i, j]
if di == dj == 0:
break
yield ''.join(para1[i-di:i]), ''.join(para2[j-dj:j])
i -= di
j -= d
Champollion
参考: https://www. aclweb.org/anthology/L0 6-1465/ https://www. aclweb.org/anthology/C1 0-2081.pdf https:// github.com/LowResourceL anguages/champollion
Champollion是基于长度和词典的对齐算法,是中国人写的,对于中-英对齐比较好。相比于Gale&Church这种对长度比较敏感(适合于英-法)的算法,Champollion更多关注到了内容。Champollion相比于其他算法的优点如下:
- Champollion假设输入有很大噪声(以往假设源语言与目标语言的match模式主要为1:1,但在中英语料中,句子对齐噪声非常大),使得删除和插入的次数变得很重要
- 与其他以词典为基础的算法不同,每个词根据对齐的重要性不同赋予了不同的权重。文中举例如下:
a. Marketplace bombing kills 23 in Iraq
b. 伊拉克 集市 爆炸 造成 23 人 死亡
在这个例子中,(23, 23)这个pair相比于(Iraq, 伊拉克)更重要。因为(Iraq, 伊拉克)相比于(23, 23)更经常出现。
Champollion因此赋予更少出现的translation pair更大的权重。
- Champollion对于每个segment pair(每个segment有1~多句话)进行打分,对于非1~1对齐进行了惩罚
句子相似度计算
论文将计算相似度问题转化为检索系统中计算query跟document相似度的问题。计算stf=segment term frequency(某个term在一个segment中出现的次数),定义

,其中T是document中的总term数。stf衡量term在segment中重要性,idtf衡量term在document中重要性。 Stf-idtf衡量了一个translate-pair对两个segment对齐的重要性。Champollion将两个segment看成对顺序不敏感的词袋:
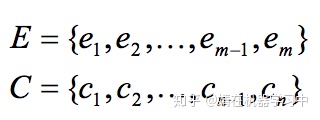
。定义k个在两个segment中出现的translate-pair:

,则E和C的相似度定义为:
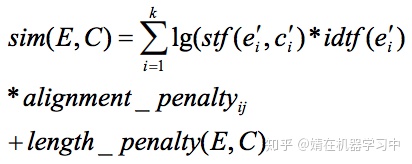
。其中alignment_penalty是一个[0, 1]的值,对于1-1的对齐其值为1。length_penalty是一个函数,对于长度不匹配的翻译要进行一下惩罚。
动态规划算法
Champollion允许1-0, 0-1, 1-1, 2-1, 1-2, 1-3, 3-1, 1-4 和 4-1对齐,其动态规划转移方程为:
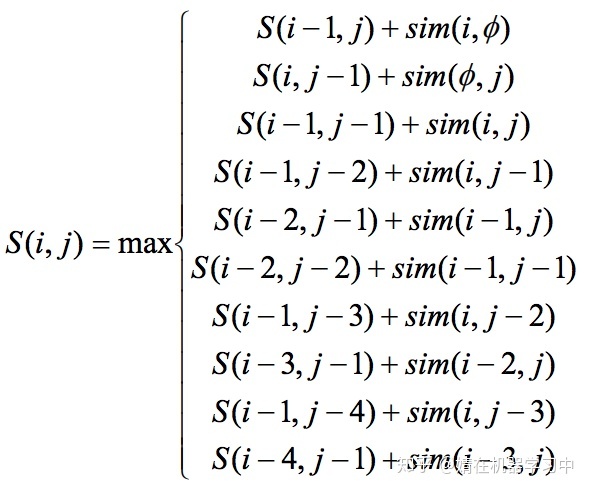
YALIGN
参考: https:// github.com/machinalis/y align https:// mailman.uib.no/public/c orpora/2013-September/018912.html
Yalign工具使用了一下,但效果不太好,它主要提供了两个功能:
- 一个句子相似度度量:给定两个句子,它就会对这两个句子相互翻译的可能性产生一个粗略的估计(0到1之间的一个数字)。
- 一个序列对齐工具:这样给定两个文档(一个句子列表),它产生一个对齐,最大化单个(每个句子对)相似度的总和。所以Yalign的主要算法实际上是一个标准序列对齐算法的包装。
在序列对齐上,Yalign使用Needleman-Wunch算法的一个变体来查找两个给定文档中的句子之间的最佳对齐。它带来的优点是,使该算法具有多项式时间最坏情况的复杂性,并产生最优对齐。反之其缺点是不能处理相互交叉的对齐或从两个句子到一个句子的对齐。关于Needleman-Wunch算法可参考https://zhuanlan.zhihu.com/p/26212767。
对齐之后,只有翻译概率高的句子才会被包含在最终的对齐中。也就是说,有些结果会被过滤,以提供高质量的校准。使用一个阈值以便在句子相似度度量足够差时排除该对。
对于句子相似度度量,Yalign使用统计分类器的似然输出,并将其调整为0-1范围。分类器被训练来确定一对句子是否互相翻译。Yalign使用支持向量机作为分类器,对齐的质量不仅取决于输入,还取决于经过训练的分类器的质量。
下面是它的一些重要函数或类的说明:
- Sentence: 训练数据的基本类型,继承list
- input_conversation.py:将文本/tmx/html格式的训练数据转化为Sentence
- YalignModel: 主类,配合basic_model函数进行模型训练、导入、预测
- SentencePairScore:定义句对的特征并进行打分
- SequenceAligner:序列对齐类
- SVMClassifier:训练一个支持向量机
- WordPairScore:词及词翻译的概率,可以使用fast-align获取s
Bleualign
参考: https://www. aclweb.org/anthology/W1 1-4624.pdf https:// github.com/rsennrich/Bl eualign
Bleualign借助机器翻译的结果进行对齐。使用机器翻译的目的是用目标语表示原文的大概意思,然后和译文进行比较,其算法的主要流程如下:
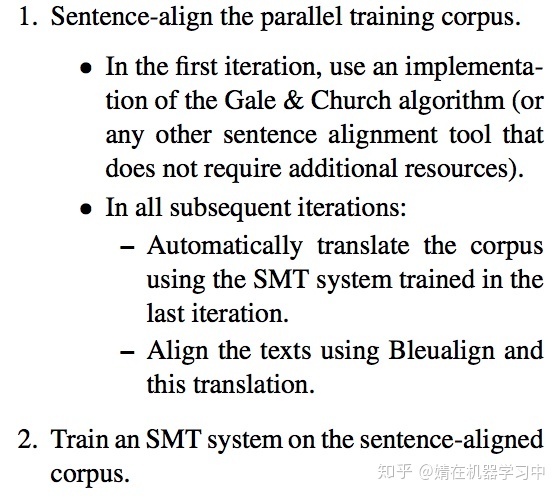
Bleualign没使用过,不知道实际效果怎么样。
Vecalign
参考: https:// github.com/thompsonb/ve calign
Vecalign: Improved Sentence Alignment in Linear Time and Space( https://www. aclweb.org/anthology/D1 9-1136.pdf )
vecalign计算句子相似度的方法跟之前不同,它使用了facebook开源的laser embedding进行句子相似度计算,计算公式如下:
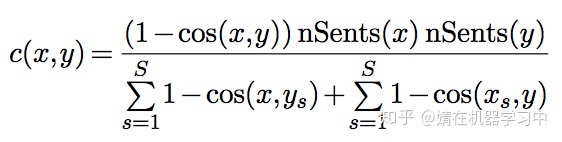
,且对于非1-1对齐采取了一定的惩罚。下面是它的核心代码:
def vecalign(vecs0,
vecs1,
final_alignment_types,
del_percentile_frac,
width_over2,
max_size_full_dp,
costs_sample_size,
num_samps_for_norm,
norms0=None,
norms1=None):
if width_over2 < 3:
logger.warning(
'width_over2 was set to %d, which does not make sense. '
'increasing to 3.', width_over2)
width_over2 = 3
# make sure input embeddings are norm==1
make_norm1(vecs0)
make_norm1(vecs1)
# save off runtime stats for summary
runtimes = OrderedDict()
# Determine stack depth
s0, s1 = vecs0.shape[1], vecs1.shape[1]
max_depth = 0
while s0 * s1 > max_size_full_dp ** 2:
max_depth += 1
s0 = s0 // 2
s1 = s1 // 2
# init recursion stack
# depth is 0-based (full size is 0, 1 is half, 2 is quarter, etc)
stack = {0: {'v0': vecs0, 'v1': vecs1}}
# downsample sentence vectors
t0 = time()
for depth in range(1, max_depth + 1):
stack[depth] = {'v0': downsample_vectors(stack[depth - 1]['v0']),
'v1': downsample_vectors(stack[depth - 1]['v1'])}
runtimes['Downsample embeddings'] = time() - t0
# compute norms for all depths, add sizes, add alignment types
t0 = time()
for depth in stack:
stack[depth]['size0'] = stack[depth]['v0'].shape[1]
stack[depth]['size1'] = stack[depth]['v1'].shape[1]
if depth == 0:
stack[depth]['alignment_types'] = final_alignment_types
else:
stack[depth]['alignment_types'] = [(1, 1)]
if depth == 0 and norms0 is not None:
if norms0.shape != vecs0.shape[:2]:
print('norms0.shape:', norms0.shape)
print('vecs0.shape[:2]:', vecs0.shape[:2])
raise Exception('norms0 wrong shape')
stack[depth]['n0'] = norms0
else:
stack[depth]['n0'] = compute_norms(
stack[depth]['v0'], stack[depth]['v1'], num_samps_for_norm)
if depth == 0 and norms1 is not None:
if norms1.shape != vecs1.shape[:2]:
print('norms1.shape:', norms1.shape)
print('vecs1.shape[:2]:', vecs1.shape[:2])
raise Exception('norms1 wrong shape')
stack[depth]['n1'] = norms1
else:
stack[depth]['n1'] = compute_norms(
stack[depth]['v1'], stack[depth]['v0'], num_samps_for_norm)
runtimes['Normalize embeddings'] = time() - t0
# Compute deletion penalty for all depths
t0 = time()
for depth in stack:
stack[depth]['del_knob'] = make_del_knob(
e_laser=stack[depth]['v0'][0, :, :],
f_laser=stack[depth]['v1'][0, :, :],
e_laser_norms=stack[depth]['n0'][0, :],
f_laser_norms=stack[depth]['n1'][0, :],
sample_size=costs_sample_size)
stack[depth]['del_penalty'] =
stack[depth]['del_knob'].percentile_frac_to_del_penalty(
del_percentile_frac)
logger.debug('del_penalty at depth %d: %f',
depth, stack[depth]['del_penalty'])
runtimes['Compute deletion penalties'] = time() - t0
tt = time() - t0
logger.debug(
'%d x %d full DP make features: %.6fs (%.3e per dot product)',
stack[max_depth]['size0'], stack[max_depth]['size1'], tt,
tt / (stack[max_depth]['size0'] + 1e-6) /
(stack[max_depth]['size1'] + 1e-6))
# full DP at maximum recursion depth
t0 = time()
stack[max_depth]['costs_1to1'] = make_dense_costs(stack[max_depth]['v0'],
stack[max_depth]['v1'],
stack[max_depth]['n0'],
stack[max_depth]['n1'])
runtimes['Full DP make features'] = time() - t0
t0 = time()
_, stack[max_depth]['x_y_tb'] = dense_dp(
stack[max_depth]['costs_1to1'], stack[max_depth]['del_penalty'])
stack[max_depth]['alignments'] = dense_traceback(
stack[max_depth]['x_y_tb'])
runtimes['Full DP'] = time() - t0
# upsample the path up to the top resolution
compute_costs_times = []
dp_times = []
upsample_depths = [0, ] if max_depth == 0 else list(
reversed(range(0, max_depth)))
for depth in upsample_depths:
if max_depth > 0: # upsample previoius alignment to current resolution
course_alignments = upsample_alignment(
stack[depth + 1]['alignments'])
# features may have been truncated when downsampleing,
# so alignment may need extended
extend_alignments(
course_alignments, stack[depth]['size0'],
stack[depth]['size1']) # in-place
else:
# We did a full size 1-1 search,
# so search same size with more alignment types
course_alignments = stack[0]['alignments']
# convert couse alignments to a searchpath
stack[depth]['searchpath'] = alignment_to_search_path(
course_alignments)
# compute ccosts for sparse DP
t0 = time()
stack[depth]['a_b_costs'], stack[depth]['b_offset'] =
make_sparse_costs(stack[depth]['v0'], stack[depth]['v1'],
stack[depth]['n0'], stack[depth]['n1'],
stack[depth]['searchpath'],
stack[depth]['alignment_types'],
width_over2)
tt = time() - t0
num_dot_products = len(stack[depth]['b_offset']) *
len(stack[depth]['alignment_types']) * width_over2 * 2
logger.debug('%d x %d sparse DP (%d alignment types, %d window) '
'make features: %.6fs (%.3e per dot product)',
stack[max_depth]['size0'], stack[max_depth]['size1'],
len(stack[depth]['alignment_types']), width_over2 * 2,
tt, tt / (num_dot_products + 1e6))
compute_costs_times.append(time() - t0)
t0 = time()
# perform sparse DP
stack[depth]['a_b_csum'], stack[depth]['a_b_xp'],
stack[depth]['a_b_yp'], stack[depth]['new_b_offset'] =
sparse_dp(
stack[depth]['a_b_costs'], stack[depth]['b_offset'],
stack[depth]['alignment_types'], stack[depth]['del_penalty'],
stack[depth]['size0'], stack[depth]['size1'])
# performace traceback to get alignments and alignment scores
# for debugging, avoid overwriting stack[depth]['alignments']
akey = 'final_alignments' if depth == 0 else 'alignments'
stack[depth][akey], stack[depth]['alignment_scores'] =
sparse_traceback(stack[depth]['a_b_csum'],
stack[depth]['a_b_xp'],
stack[depth]['a_b_yp'],
stack[depth]['new_b_offset'],
stack[depth]['size0'],
stack[depth]['size1'])
dp_times.append(time() - t0)
runtimes['Upsample DP compute costs'] = sum(compute_costs_times[:-1])
runtimes['Upsample DP'] = sum(dp_times[:-1])
runtimes['Final DP compute costs'] = compute_costs_times[-1]
runtimes['Final DP'] = dp_times[-1]
# log time stats
max_key_str_len = max([len(key) for key in runtimes])
for key in runtimes:
if runtimes[key] > 5e-5:
logger.info(
key + ' took ' + '.' * (
max_key_str_len + 5 - len(key)) + (
'%.4fs' % runtimes[key]).rjust(7))
其他相关资源
- https://github.com/cocoxu/Shakespeare/tree/master/bilingual-sentence-aligner
- https://github.com/loomchild/maligna