本部分内容来自Spark Deploying 和 Spark大数据处理相关内容
Spark的运行模式多种多样,灵活多变.
该系统当前支持几个集群管理器:
- 单机版 – Spark附带的简单群集管理器,可轻松设置群集。
- Apache Mesos –通用集群管理器,也可以运行Hadoop MapReduce和服务应用程序。
- Hadoop YARN – Hadoop 2中的资源管理器。
- Kubernetes –一个开源系统,用于自动化、容器化应用程序的部署,扩展和管理。
一、Spark运行模式概述
【1.1】运行模式概述
Spark的运行模式取决于传递给SparkContext
的MASTER
环境变量的值,传递给Spark的特定字符串或者URL可以采用以下格式之一
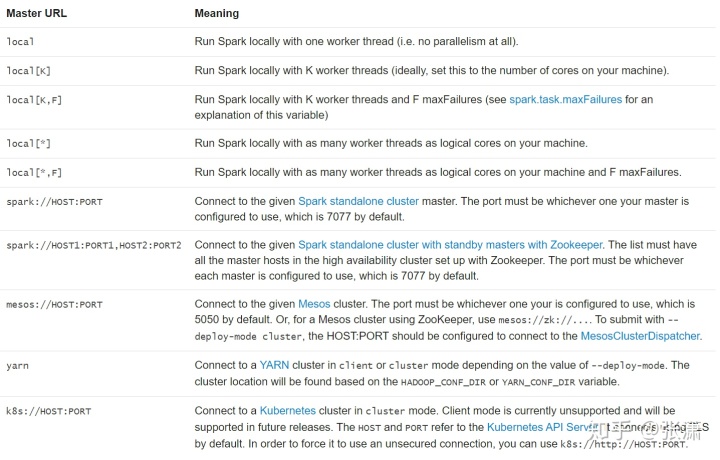
【1.2】Spark的基本工作流程
Spark的工作流程可以分为2大部分,分别是任务调度和任务执行.
从下图可以看到Spark引用程序离不开SparkContext
和Executor
两部分。Executor
负责执行任务,运行Executor
的机器称为Worker
节点,SparkContext
由用户程序启动,通过资源调度模块和Executor
通信。
SparkContext
和Executor
这两部分的核心代码实现在各种运行模式中都是公用的,在它们之上,根据运行部署模式的不同,包装了不同调度模块以及相关的适配代码。
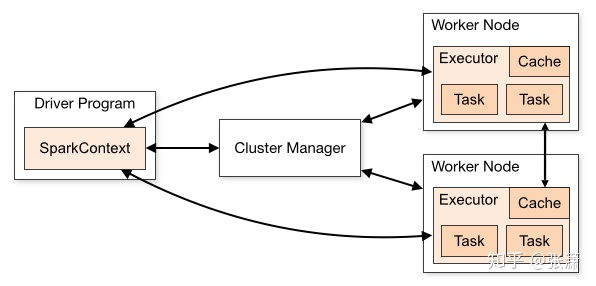
具体来说,以SparkContext
为程序运行的总入口,在SparkContext
的初始化过程中,Spark会分别创建DAGScheduler
作业调度和TaskScheduler
任务调度两级调度模块。
其中作业调度模块是基于任务阶段的高层调度模块,它为每个Spark作业计算具有依赖关系的多个调度阶段stage
(通常根据shuffle
来划分),然后为每个stage
构建出一组具体的任务(通常会考虑数据的本地性等),然后以TaskSets
(任务组)的形式提交给任务调度模块来具体执行。而任务调度模块则负责具体启动任务、监控和汇报任务运行情况。
作业调度模块和具体的部署运行模式无关,在各种运行模式下逻辑相同,因此在本章不做过多介绍,我们会在后面介绍调度管理的章节中再详细分析。
不同运行模式的区别主要体现在任务调度模块。不同的部署和运行模式,根据底层资源调度方式的不同,各自实现了自己特定的任务调度模块,用来将任务实际调度给对应的计算资源。
【1.3】相关基本类
TaskScheduler/SchedulerBackend
为了抽象出一个公共的接口供DAGScheduler
作业调度模块使用,所有的这些运行模式实现的任务调度模块都是基于这两个接口(Trait)的TaskScheduler
及SchedulerBackend
。
private[spark] trait TaskScheduler {
private val appId = "spark-application-" + System.currentTimeMillis
def rootPool: Pool
def schedulingMode: SchedulingMode
def start(): Unit
// Invoked after system has successfully initialized (typically in spark context).
// Yarn uses this to bootstrap allocation of resources based on preferred locations,
// wait for slave registrations, etc.
def postStartHook() { }
// Disconnect from the cluster.
def stop(): Unit
// Submit a sequence of tasks to run.
def submitTasks(taskSet: TaskSet): Unit
// Cancel a stage.
def cancelTasks(stageId: Int, interruptThread: Boolean)
// Set the DAG scheduler for upcalls. This is guaranteed to be set before submitTasks is called.
def setDAGScheduler(dagScheduler: DAGScheduler): Unit
// Get the default level of parallelism to use in the cluster, as a hint for sizing jobs.
def defaultParallelism(): Int
/**
* Update metrics for in-progress tasks and let the master know that the BlockManager is still
* alive. Return true if the driver knows about the given block manager. Otherwise, return false,
* indicating that the block manager should re-register.
*/
def executorHeartbeatReceived(execId: String, taskMetrics: Array[(Long, TaskMetrics)],
blockManagerId: BlockManagerId): Boolean
/**
* Get an application ID associated with the job.
*
* @return An application ID
*/
def applicationId(): String = appId
/**
* Process a lost executor
*/
def executorLost(executorId: String, reason: ExecutorLossReason): Unit
/**
* Get an application's attempt ID associated with the job.
*
* @return An application's Attempt ID
*/
def applicationAttemptId(): Option[String]
}
TaskScheduler
的实现主要用于与DAGScheduler
交互,负责任务的具体调度和运行,其核心方法是submitTasks
和cancelTasks
。
private[spark] trait SchedulerBackend {
private val appId = "spark-application-" + System.currentTimeMillis
def start(): Unit
def stop(): Unit
def reviveOffers(): Unit
def defaultParallelism(): Int
def killTask(taskId: Long, executorId: String, interruptThread: Boolean): Unit =
throw new UnsupportedOperationException
def isReady(): Boolean = true
/**
* Get an application ID associated with the job.
*
* @return An application ID
*/
def applicationId(): String = appId
/**
* Get the attempt ID for this run, if the cluster manager supports multiple
* attempts. Applications run in client mode will not have attempt IDs.
*
* @return The application attempt id, if available.
*/
def applicationAttemptId(): Option[String] = None
/**
* Get the URLs for the driver logs. These URLs are used to display the links in the UI
* Executors tab for the driver.
* @return Map containing the log names and their respective URLs
*/
def getDriverLogUrls: Option[Map[String, String]] = None
}
SchedulerBackend
的实现是与底层资源调度系统交互(如Mesos/YARN
),配合TaskScheduler
实现具体任务执行所需的资源分配,核心接口是receiveOffers
。
这两者之间的实际交互过程取决于具体调度模式,理论上这两者的实现是成对匹配工作的,之所以拆分成两部分,是有利于相似的调度模式共享代码功能模块。
Executor
实际任务的运行,最终都由Executor
类来执行,Executor
对每一个任务创建一个TaskRunner
类对象,交给线程池运行
// Start worker thread pool
private val threadPool = ThreadUtils.newDaemonCachedThreadPool("Executor task launch worker")
// Maintains the list of running tasks.
private val runningTasks = new ConcurrentHashMap[Long, TaskRunner]
def launchTask(
context: ExecutorBackend,
taskId: Long,
attemptNumber: Int,
taskName: String,
serializedTask: ByteBuffer): Unit = {
val tr = new TaskRunner(context, taskId = taskId, attemptNumber = attemptNumber, taskName,
serializedTask)
runningTasks.put(taskId, tr)
threadPool.execute(tr)
}
待续未完....