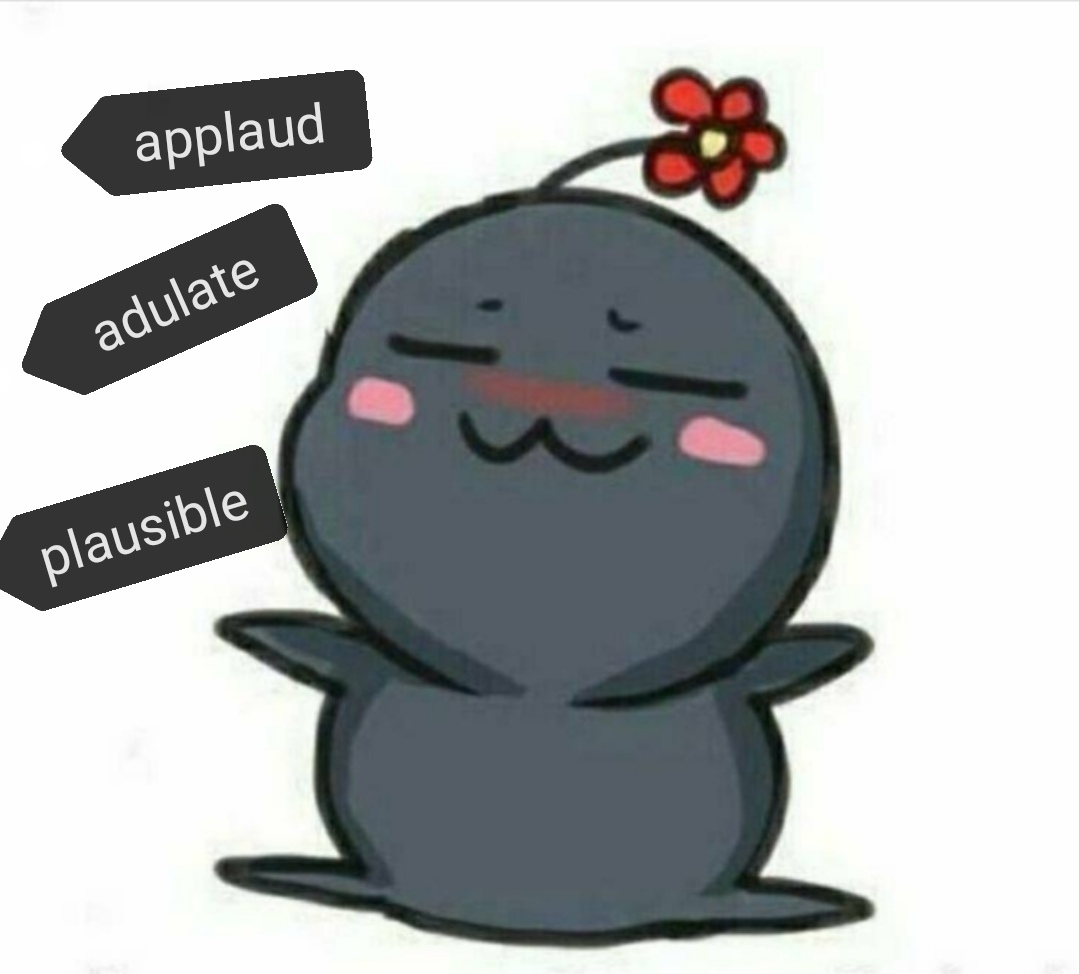
我们现在的时间序列课程简直了,PPT上好多typo,记号也很乱,网课卡成一个字一个字的。。。人生艰难
这篇会分享:1.一些关于资产收益的性质;2.模拟GARCH模型的代码;3.关于条件异方差的一些相关代码。
我对着老师时常有错误的PPT和R code瞪了半天终于感觉自己似乎理清了思路,然后模模糊糊地完成了作业。大家如果有发现错误或者需要改进的地方,欢迎提出~
这里,我们假设GARCH(p,q)模型为【可能和你们一般的p,q恰好反过来,我们老师PPT上全部是这样顺序的记号】:
这里,我们称
一些关于资产收益的性质 (stylized facts of asset returns)
来自论文Empirical properties of asset returns: stylized facts and statistical issues
在金融资产里,如果我们收集到了一个时间序列,比如某金融资产的日价格
1.缺乏自相关性(absence of autocorrelation)
资产收益
2. 重尾(heavy tails)
资产收益
3.收益/损失不对称(gain/loss asymmetry)
股票价格与股票指数有很大的衰减但是极大的上升趋势却是不相等的【这里我也很迷惑,我不了解股票。。。】
4.聚集高斯性(aggregational gaussianity)
当我们增大了收益的计算时间间隔
5.间歇性(intermittency)
收益在任意的时间间隔下都表现出极大的可变性(variability),通常,我们会画出波动率估计值(volatility estimators)的时间序列图,而在这个时间序列图上会出现不规则的波动(irregular bursts)。
6.波动聚集性(volatility clustering)
波动率会在几天内呈现的正的自相关系数。
7.条件重尾(conditional heavy tails)
我们在利用了GARCH模型进行拟合之后,相当于对波动聚集性做了校正,但是模型的残差序列仍然表现出重尾。但是,这里的重尾会比收益非条件的分布平缓一些。
8.收益绝对值自相关系数缓慢衰减(slow decay of autocorrelation in absolute returns)
收益的绝对值的自相关系数随着时间呈现缓慢的衰减。
9.杠杆效应(leverage effect)
很多情况下,波动率与收益呈现负相关
10.体积/波动 相关性(volumn/volatility correlation)
交易量(tradeing volume)与所有的波动率相关。
11.时间不对称性(asymmetry in time scales)
粗粒度的波动率比细粒度的波动率更容易预测。(这个我完全不理解)
一个模拟GARCH模型的代码
library(TSA)
c=0.1
b=0.08 #beta
a=0.82 #alpha
#.Random.seed = .seed20140224
x= 1:2000 #data structure
v=x #data structure
v[1]=c/(1-a-b) #unconditional variance
x[1]=rnorm(1)*sqrt(v[1]) #unconditional marginal
for(i in 2:2000)
{
v[i] = c+a*x[i-1]*x[i-1]+b*v[i-1]
x[i] = sqrt(v[i])*rnorm(1)
}
x = x[1001:2000]
plot(x)
sigma=sqrt(v[1001:2000])
#library("TSA")
sd(x); kurtosis(x)
#x=garch.sim(alpha=c(c, a), beta=b, n=1000)
#tt="package:TSA"
#detach(tt, character.only = TRUE) # detach TSA from the current session
plot(x, type="l", xlab='t', ylab='', main='(a) GARCH(1,1) time series', col=4)
#points(x,pch=20,col=2)
hist(x, xlab='', nclass=25, probability=T, col=3, ylab='', main='(b) Histogram')
mu=mean(x); v=sqrt(var(x))
h=6*v/200
vv=1:200
vv[1]=mu-3*v
for(i in 2:200) vv[i]=vv[i-1]+h
y=dnorm(vv,mu,v)
lines(vv,y, col=2,lwd=2)
plot(sigma, type="l", xlab='t', ylab='', main='(c) Conditional STD', col=4)
qqnorm(x, xlab='Normal quantile', ylab='GARCH quantile', main='(d) QQ-plot', col=4)
qqline(x, col=2)
acf(x, lag=30, main='(e) ACF', ylab='', col="green")
acf(x*x, lag=30, main='(f) ACF of squared series', ylab='', col="green")
#plot(r, main='(e) ACF', ylab='', col=5)
#plot(rr, ylab='', main='(f) ACF of squared series',col="brown")
dev.off()
关于条件异方差的一些相关代码
后边有时间再来更~