Python神经影像社区日趋完善,Linux shell搭配Python的神经影像工具开发方式越来越受追捧,催生出fMRIPrep、fMRIDenoise、Nipype、Nilearn、PyMVPA等优秀的toolbox,今天给大家介绍一个前期准备数据,自动整理BIDS格式的软件
基于Linux及MacOS的Heudiconv是一款很方便的dicom转nifti格式的工具,不同于dcm2nii等传统软件仅提供格式转换,Heudiconv的亮点在于自动组织图像路径,生成结构化的影像数据存储模式——BIDS格式
软件包的细节不赘述了,感兴趣的可以去Heudiconv的官网去研究:
https://neuroimaging-core-docs.readthedocs.io/en/latest/pages/heudiconv.htmlneuroimaging-core-docs.readthedocs.io这里主要分享自己使用Heudiconv的具体操作步骤:
Step 1:整理自己的Dicom数据格式
首先我们需要将自己的Dicom格式文件整理好,建议存放的路径为项目文件夹 -> Dicom数据文件夹 -> 被试文件夹 -> session文件夹 (optional) -> 各模态文件夹 -> 具体的DCM/IMA文件
/Project
/SourceData
/001
/pretreatment (optional)
T1
Func
...
/sub002
/sub003
...
session一般指研究里大部分被试参与了的两次及以上相同扫描序列的扫描,纵向研究中涉及较多,如治疗前后,或者纵向多时间点随访等,如果你的数据涉及可以加这一级文件夹,如果不涉及则可以忽略这一级。
Step 2:读取原始Dicom数据头文件信息
现在开始任意读取一个Dicom数据的头文件信息
sudo docker run --rm -it -v /home/dell/project:/base nipy/heudiconv:latest -d /base/SourceData/{subject}/{session}/*/*.dcm -o /base/RawData/ -f convertall -s 001 -ss pretreatment -c none --overwrite
这里借助Docker运行(推荐),具体安装参照官网及我在fMRIPrep里给出的docker安装方法
-v 后面时定义你的project所在路径为base路径
nipy/heudiconv:latest 则是调用最新版的heudiconv
-d 后面是所有dcm文件存放的位置,利用了通配符实现,{subject}和{session}必须这样表示,用来获取相应字段名命名后续的Nifti
-o Nifti文件放置的位置,这里我们命名为RawData
-s 是你选择的被试文件夹名
-ss 是你选择的session文件夹名 (optional)
其他参数完全一致不用变
运行过后,我们会在RawData下找到一个.heudiconv的隐藏文件夹,显示隐藏文件夹如下勾选Show hidden files (Ubuntu)
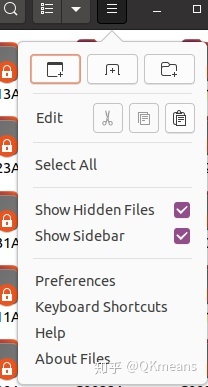
这样在.heudiconv/001/info 下会有如下几个文件,我们重点关注dicominfo.tsv和heuristic.py两个文件
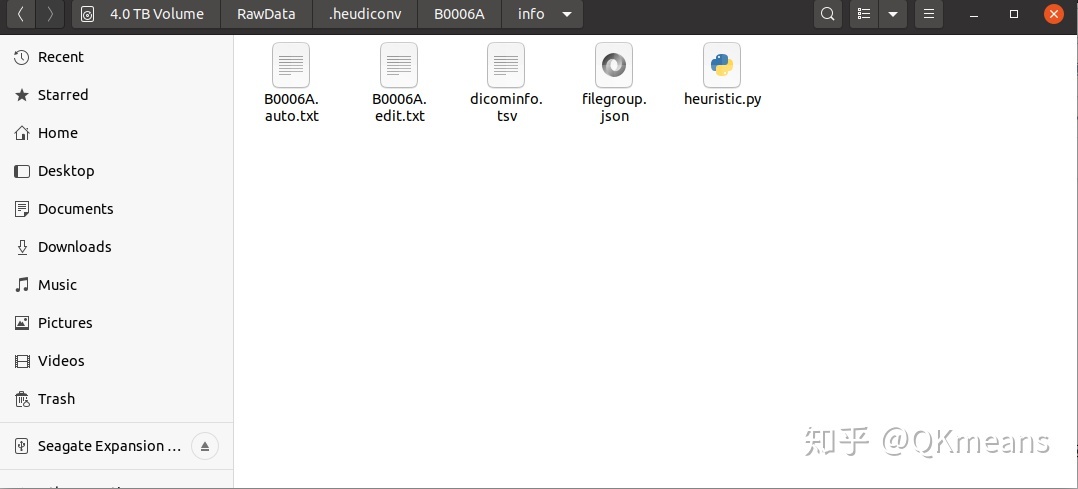
打开这个tsv文件,观察我们所有序列的头文件信息(用一个公开数据做演示)

观察你序列的信息用来创建关键的heuristic.py文件
我们将这里的heuristic.py文件拷贝出来,推荐RawData目录下创建一个code文件夹,符合BIDS社区规范,并且修改下权限(如果非root用户可能这个文件是只读)
# 拷贝文件
sudo cp /home/dell/project/RawData/.heudiconv/001/info/heuristic.py /home/dell/project/Rawdata/code/
# 修改整个code文件夹的权限
sudo chmod -R 777 /home/dell/project/RawData/code
打开heuristic.py文件并修改,不推荐使用普通的文本编辑器打开,这样会导致一些缩进错误的问题,熟悉Python的话,最好用Pycharm打开修改,熟悉Linux的话可以尝试用vim打开修改,未经修改的文件如下:
import os
def create_key(template, outtype=('nii.gz',), annotation_classes=None):
if template is None or not template:
raise ValueError('Template must be a valid format string')
return template, outtype, annotation_classes
def infotodict(seqinfo):
"""Heuristic evaluator for determining which runs belong where
allowed template fields - follow python string module:
item: index within category
subject: participant id
seqitem: run number during scanning
subindex: sub index within group
"""
data = create_key('run{item:03d}')
info = {data: []}
last_run = len(seqinfo)
for s in seqinfo:
"""
The namedtuple `s` contains the following fields:
* total_files_till_now
* example_dcm_file
* series_id
* dcm_dir_name
* unspecified2
* unspecified3
* dim1
* dim2
* dim3
* dim4
* TR
* TE
* protocol_name
* is_motion_corrected
* is_derived
* patient_id
* study_description
* referring_physician_name
* series_description
* image_type
"""
info[data].append(s.series_id)
return info
文件里定义了两个函数,第一个我们不用管,重点是第二个,需要参考dicominfo.tsv文件来对我们想转换的序列做筛选
比如根据上面的dicominfo.tsv我想转T1和rest两个模态,则heuristic.py文件修改如下:
import os
def create_key(template, outtype=('nii.gz',), annotation_classes=None):
if template is None or not template:
raise ValueError('Template must be a valid format string')
return template, outtype, annotation_classes
def infotodict(seqinfo):
"""Heuristic evaluator for determining which runs belong where
allowed template fields - follow python string module:
item: index within category
subject: participant id
seqitem: run number during scanning
subindex: sub index within group
"""
t1w = create_key('sub-{subject}/ses-{session}/anat/sub-{subject}_ses-{session}_T1w')
rest = create_key('sub-{subject}/ses-{session}/func/sub-{subject}_ses-{session}_task-rest_bold')
info = {t1w: [], rest: []}
last_run = len(seqinfo)
for s in seqinfo:
"""
The namedtuple `s` contains the following fields:
* total_files_till_now
* example_dcm_file
* series_id
* dcm_dir_name
* unspecified2
* unspecified3
* dim1
* dim2
* dim3
* dim4
* TR
* TE
* protocol_name
* is_motion_corrected
* is_derived
* patient_id
* study_description
* referring_physician_name
* series_description
* image_type
"""
if (s.dim3 == 176) and ('mpr' in s.protocol_name):
info[t1w].append(s.series_id)
if (s.dim4 == 175) and ('rest' in s.protocol_name):
info[rest].append(s.series_id)
return info
这里改动了的地方(需要一些轻度Python基础):
1. data = create_key('run{item:03d}')
改为(声明Nifti文件命名规则):
t1w = create_key('sub-{subject}/ses-{session}/anat/sub-{subject}_ses-{session}_T1w')
rest = create_key('sub-{subject}/ses-{session}/func/sub-{subject}_ses-{session}_task-rest_bold')
2. for循环里添加筛选条件,需要一些能区分出该序列的特征,s所涉及的所有字段都可以拿来作筛选,比如dim3(图像层数),protocol名称等
if (s.dim3 == 176) and ('mpr' in s.protocol_name):
info[t1w].append(s.series_id)
if (s.dim4 == 175) and ('rest' in s.protocol_name):
info[rest].append(s.series_id)
更改完成后保存退出即可
Step 3:调用dcm2niix重新运行Heudiconv,通过shell的for循环实现所有被试的批处理转换
cd /home/dell/project/SourceData/
for sub in `ls -d *`
do
sudo docker run --rm -it -v /home/dell/project:/base nipy/heudiconv:latest -d /base/SourceData/{subject}/{session}/*/*.dcm -o /base/RawData/ -f /base/RawData/code/heuristic.py -s $sub -ss pretreatment -c dcm2niix -b --overwrite
done
这样整个处理过程即完成,参考下我的SourceData和最后生成的RawData
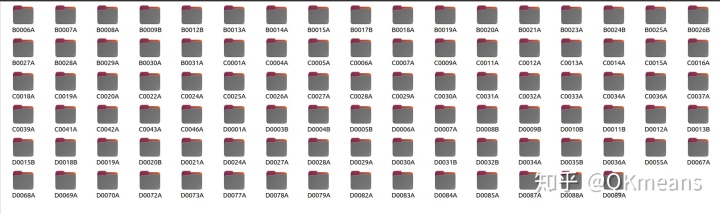
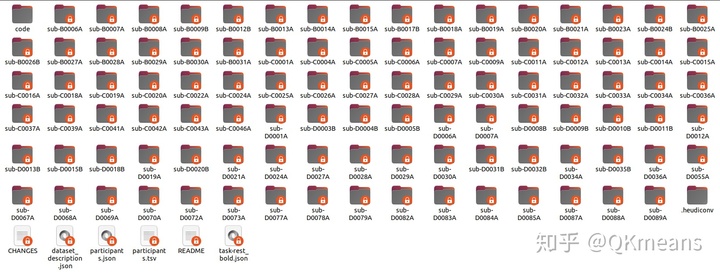

最后再把RawData放入BIDS validator验证这是不是一个有效的BIDS dataset(BIDS的介绍、命名规范以及BIDS validator,可参考之前fMRIPrep的介绍)
QKmeans:fMRIPrep安装与使用笔记---BIDS formatzhuanlan.zhihu.com这样的RawData就可以直接放入fMRIPrep、MRIQC等支持BIDS格式的toolbox中进行预处理
关于Heudiconv更多详细信息可参考:
DICOMS to BIDSnipy.orgBIDS Tutorial Series: HeuDiConv Walkthrough DICOMS to BIDSnipy.org BIDS Tutorial Series: HeuDiConv Walkthroughreproducibility.stanford.edu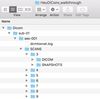