我的小程序:
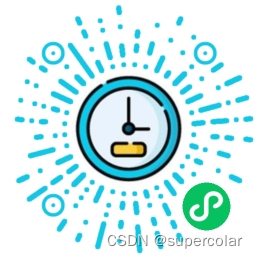
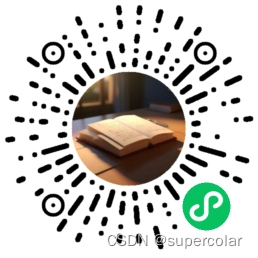
首先,nn.ModuleList 和 nn.Sequential都是用来封装多个层。
区别在于:
nn.Sequential有forward()方法,如:
nn.Sequential allows you to build a neural net by specifying sequentially the building blocks (nn.Module's) of that net.
simple_cnn = nn.Sequential(
nn.Conv2d(3, 32, kernel_size=7, stride=2),
nn.ReLU(inplace=True),
Flatten(),
nn.Linear(5408, 10),
)
y = simple_cnn(x)#可直接调用nn.Sequential的faward()方法
实例化一个nn.Sequential的对象simple_cnn,可直接调用simple_cnn(x),调用的就是nn.Sequential的forward()方法,这样会把nn.Sequential里的所有层都forward(),一步到位。
而nn.ModuleList仅仅类似于pytho中的list类型,只是将一系列层装入列表,并没有forward()方法,如定义自己的一个网络:
nn.ModuleList is just like a Python list. It was designed to store any desired number of nn.Module's. It may be useful, for instance, if you want to design a neural network whose number of layers is passed as input:
class LinearNet(nn.Module):
def __init__(self, input_size, num_layers, layers_size, output_size):
super(LinearNet, self).__init__()
self.linears = nn.ModuleList([nn.Linear(input_size, layers_size)])
self.linears.extend([nn.Linear(layers_size, layers_size) for i in range(1, self.num_layers-1)])
self.linears.append(nn.Linear(layers_size, output_size)
可以看到nn.ModuleList有python list中的extend,append等方法。因为nn.ModuleList没有forward()方法,所以装入nn.ModuleList的层需要自己循环调用forward(),当然,这样有了更多的灵活性,可以在每一层的输入输出中做一些自己的处理。如:
#self._blocks是一个nn.ModuleList
for idx, block in enumerate(self._blocks):
drop_connect_rate = self._global_params.drop_connect_rate
if drop_connect_rate:
drop_connect_rate *= float(idx) / len(self._blocks)
x = block(x, drop_connect_rate=drop_connect_rate)
nn.Sequential的优点是可以一步到位froward,方便高效,但缺少灵活性;nn.ModuleList的优点是灵活性高,但需要手动forward。各有自己适用的场景。
虽然nn.ModuleList就是一个list的功能,但不能用python中的list代替nn.ModuleList,因为pytorch不能识别放在python list中的模型变量。
In nn.Sequential, the nn.Module's stored inside are connected in a cascaded way. For instance, in the example that I gave, I define a neural network that receives as input an image with 3 channels and outputs 10 neurons. That network is composed by the following blocks, in the following order: Conv2D -> ReLU -> Linear layer. Moreover, an object of type nn.Sequential has a forward() method, so if I have an input image x I can directly call y = simple_cnn(x) to obtain the scores for x. When you define an nn.Sequential you must be careful to make sure that the output size of a block matches the input size of the following block. Basically, it behaves just like a nn.Module
On the other hand, nn.ModuleList does not have a forward() method, because it does not define any neural network, that is, there is no connection between each of the nn.Module's that it stores. You may use it to store nn.Module's, just like you use Python lists to store other types of objects (integers, strings, etc). The advantage of using nn.ModuleList's instead of using conventional Python lists to store nn.Module's is that Pytorch is “aware” of the existence of the nn.Module's inside an nn.ModuleList, which is not the case for Python lists. If you want to understand exactly what I mean, just try to redefine my class LinearNet using a Python list instead of a nn.ModuleList and train it. When defining the optimizer() for that net, you'll get an error saying that your model has no parameters, because PyTorch does not see the parameters of the layers stored in a Python list. If you use a nn.ModuleList instead, you'll get no error.
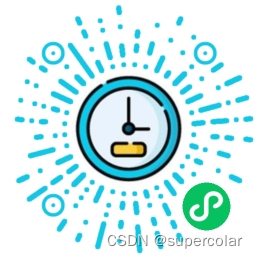
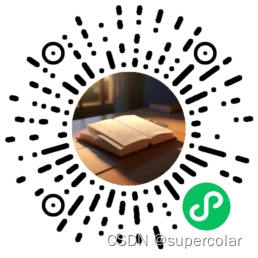