Github链接https://github.com/open-mmlab官网链接
https://openmmlab.com
目录
算法库详解
目标检测算法库MMDetection
MMDetectionhttp://github.com/open-mmlab/mmdetection
MMDetection 支持了各种不同的检测任务,包括目标检测,实例分割,全景分割,以及半监督目标检测。可以按照自己需求做自己的数据集并使用现有的框架快速训练、推理。
算法框架
模块组件
Backbones | Necks | Loss | Common |
|
目标检测算法库MMYOLO
MMYOLO 中目前实现了目标检测和旋转框目标检测算法,但是相比 MMDeteciton 版本有显著训练加速,训练速度相比原先版本提升 2.6 倍。
MMYOLOhttps://github.com/open-mmlab/mmyolo
文字检测识别算法库MMOCR
MMOCRhttps://github.com/open-mmlab/mmocr
专注于文本检测,文本识别以及相应的下游任务,如关键信息提取。 它是 OpenMMLab 项目的一部分。兼容中文,英文和数字。
3D目标检测算法库MMDetection3D
MMDection3Dhttps://github.com/open-mmlab/mmdetection3d
面向 3D 检测的平台
旋转目标检测算法库MMRotate
图像分割算法库MMSegmentation
图像分类+预训练+多模态算法库MMPretrain
- 图像分类:输入图像,AI给出类别的概率,概率最高的就是最后的类型
- 图像描述(Image Caption):输入图片,AI使用文字描述图片
- 视觉问答(Visual Question Answering):输入图片,问AI一个问题,AI根据图片给出答案
- 视觉定位(Visual Grounding):输入图片,给出提示提,AI根据提示词换出框
- 检索(图搜图,图搜文,文搜图)
姿态估计算法库MMPose
除了人体关键提检测也可以做自己标注数据的关键点检测
三维人体姿态估计算法库MMHuman3D
视频动作识别算法库MMAction2
动作识别,时序动作检测,时空动作检测以及基于人体姿态的动作识别
无法通过单帧画面,需要结合上下文判断
目标追踪MMTracking
支持视频目标检测,多目标跟踪,单目标跟踪和视频实例分割等多种任务和算法。
GitHub - open-mmlab/mmtracking: OpenMMLab Video Perception Toolbox. It supports Video Object Detection (VID), Multiple Object Tracking (MOT), Single Object Tracking (SOT), Video Instance Segmentation (VIS) with a unified framework.OpenMMLab Video Perception Toolbox. It supports Video Object Detection (VID), Multiple Object Tracking (MOT), Single Object Tracking (SOT), Video Instance Segmentation (VIS) with a unified framework. - GitHub - open-mmlab/mmtracking: OpenMMLab Video Perception Toolbox. It supports Video Object Detection (VID), Multiple Object Tracking (MOT), Single Object Tracking (SOT), Video Instance Segmentation (VIS) with a unified framework.
https://github.com/open-mmlab/mmtracking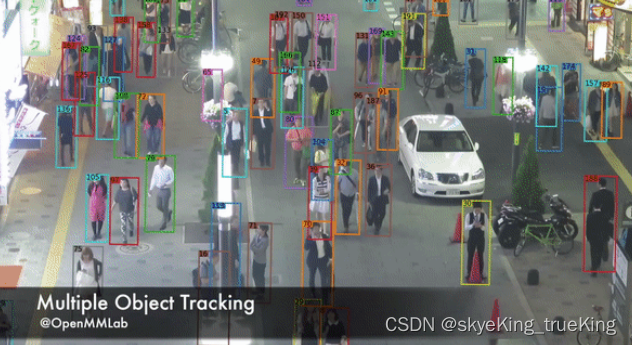
生成模型+底层视觉+AIGC算法库MMagic
支持了流行的图像修复、图文生成、3D生成、图像修补、抠图、超分辨率和生成等任务的应用。 支持 Stable Diffusion 的微调和ControlNet 动画生成。也支持 GANs 的插值,投影,编辑和其他流行的应用。
- 文生图,图生图,图像填充(inpainting)
- 图像去噪,重建,超分辨率,去雾
- 视频插帧,超分辨率
- 黑白老照片上色
- Matting(抠人)
模型部署工具箱MMDeploy
为各算法库提供统一的部署体验
趣味应用游乐场Playground
一个用于收集和展示 OpenMMLab 相关前沿和有趣应用的项目
https://github.com/open-mmlab/playgroundhttps://github.com/open-mmlab/playground