目录
1. 日期转换
1.1 数据集
2. 用注意力模型进行机器翻译
2.1 注意力机制
3. 可视化注意力
1. 数据合成:创建语音数据集
1.1 听一下数据
1.2 音频转频谱
1.3 生成一个训练样本
1.4 全部训练集
1.5 开发集
2. 模型
2.1 建模
2.2 训练
2.3 测试模型
3. 预测
3.3 在开发集上测试
4. 用自己的样本测试
测试题:参考博文
笔记:W3.序列模型和注意力机制
作业1:机器翻译
建立一个神经元机器翻译(NMT)模型来将人类可读日期(25th of June, 2009
)翻译成机器可读日期(“2009—06—25”
)
将使用「注意力模型」来实现这一点,这是最复杂的 序列到序列 模型之一
注意安装包
pip install Faker==2.0.0
pip install babel
- 导入包
from keras.layers import Bidirectional, Concatenate, Permute, Dot, Input, LSTM, Multiply
from keras.layers import RepeatVector, Dense, Activation, Lambda
from keras.optimizers import Adam
from keras.utils import to_categorical
from keras.models import load_model, Model
import keras.backend as K
import numpy as np
from faker import Faker
import random
from tqdm import tqdm
from babel.dates import format_date
from nmt_utils import *
import matplotlib.pyplot as plt
%matplotlib inline
1. 日期转换
模型将输入以各种可能格式书写的日期(例如"the 29th of August 1958", "03/30/1968", "24 JUNE 1987"
),并将其转换为标准化、机器可读的日期(如 "1958-08-29", "1968-03-30", "1987-06-24"
)。我们将让模型学习以通用机器可读格式YYYY-MM-DD
输出日期
1.1 数据集
- 1万条数据
m = 10000
dataset, human_vocab, machine_vocab, inv_machine_vocab = load_dataset(m)
- 打印看看
dataset[:10]
输出:
[('9 may 1998', '1998-05-09'),
('10.11.19', '2019-11-10'),
('9/10/70', '1970-09-10'),
('saturday april 28 1990', '1990-04-28'),
('thursday january 26 1995', '1995-01-26'),
('monday march 7 1983', '1983-03-07'),
('sunday may 22 1988', '1988-05-22'),
('08 jul 2008', '2008-07-08'),
('8 sep 1999', '1999-09-08'),
('thursday january 1 1981', '1981-01-01')]
上面加载了:
dataset
human_vocab
: 字典, human readable dates :an integer-valued indexmachine_vocab
: 字典, machine readable dates :an integer-valued indexinv_machine_vocab
: 字典,machine_vocab的反向映射,indices :characters
Tx = 30 # 最大输入长度,如果大了,就截断
Ty = 10 # 输出日期长度 YYYY-MM-DD
X, Y, Xoh, Yoh = preprocess_data(dataset, human_vocab, machine_vocab, Tx, Ty)
print("X.shape:", X.shape)
print("Y.shape:", Y.shape)
print("Xoh.shape:", Xoh.shape)
print("Yoh.shape:", Yoh.shape)
输出:
X.shape: (10000, 30)
Y.shape: (10000, 10)
Xoh.shape: (10000, 30, 37) # 37 是 len(human_vocab)
Yoh.shape: (10000, 10, 11) # 11 是 日期中的字符种类 0-9 和 ‘-’
- 看看数据(数据不够长度的,会补充 pad,所有 x 都是 30 长度)
index = 52
print("Source date:", dataset[index][0])
print("Target date:", dataset[index][1])
print()
print("Source after preprocessing (indices):", X[index])
print("Target after preprocessing (indices):", Y[index])
print()
print("Source after preprocessing (one-hot):", Xoh[index])
print("Target after preprocessing (one-hot):", Yoh[index])
输出:
Source date: saturday october 9 1976
Target date: 1976-10-09
Source after preprocessing (indices): [29 13 30 31 28 16 13 34 0 26 15 30 26 14 17 28 0 12 0 4 12 10 9 36
36 36 36 36 36 36]
Target after preprocessing (indices): [ 2 10 8 7 0 2 1 0 1 10]
Source after preprocessing (one-hot): [[0. 0. 0. ... 0. 0. 0.]
[0. 0. 0. ... 0. 0. 0.]
[0. 0. 0. ... 0. 0. 0.]
...
[0. 0. 0. ... 0. 0. 1.]
[0. 0. 0. ... 0. 0. 1.]
[0. 0. 0. ... 0. 0. 1.]]
Target after preprocessing (one-hot): [[0. 0. 1. 0. 0. 0. 0. 0. 0. 0. 0.]
[0. 0. 0. 0. 0. 0. 0. 0. 0. 0. 1.]
[0. 0. 0. 0. 0. 0. 0. 0. 1. 0. 0.]
[0. 0. 0. 0. 0. 0. 0. 1. 0. 0. 0.]
[1. 0. 0. 0. 0. 0. 0. 0. 0. 0. 0.]
[0. 0. 1. 0. 0. 0. 0. 0. 0. 0. 0.]
[0. 1. 0. 0. 0. 0. 0. 0. 0. 0. 0.]
[1. 0. 0. 0. 0. 0. 0. 0. 0. 0. 0.]
[0. 1. 0. 0. 0. 0. 0. 0. 0. 0. 0.]
[0. 0. 0. 0. 0. 0. 0. 0. 0. 0. 1.]]
2. 用注意力模型进行机器翻译
2.1 注意力机制
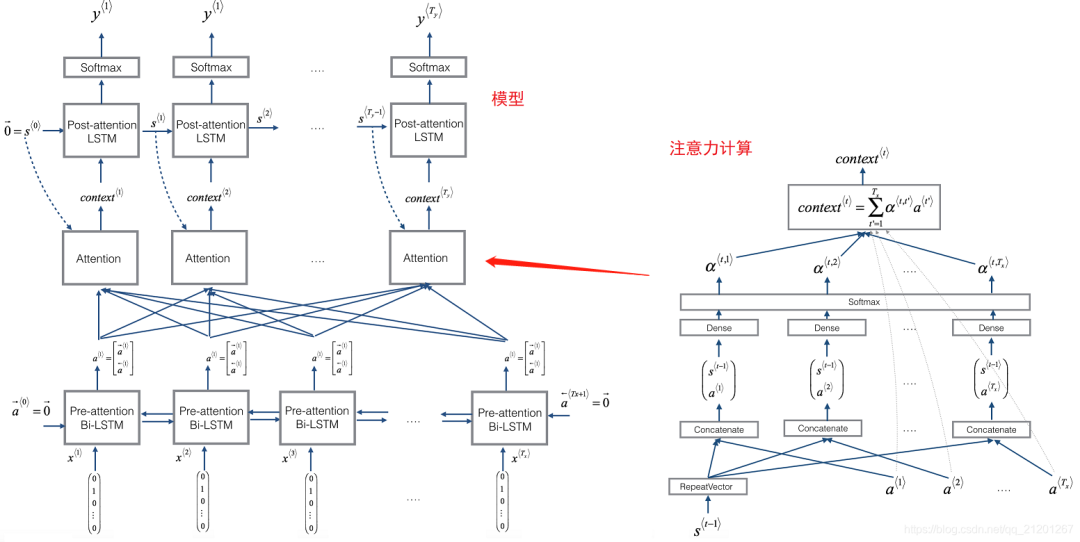
将输入重复几次:https://keras.io/zh/layers/core/#repeatvector输入张量通过 axis 轴串联起来 https://keras.io/zh/layers/merge/#concatenate_1https://keras.io/zh/layers/wrappers/#bidirectional
# Defined shared layers as global variables
repeator = RepeatVector(Tx)
concatenator = Concatenate(axis=-1)
densor1 = Dense(10, activation = "tanh")
densor2 = Dense(1, activation = "relu")
activator = Activation(softmax, name='attention_weights')
# We are using a custom softmax(axis = 1) loaded in this notebook
dotor = Dot(axes = 1)
- 注意力计算
# GRADED FUNCTION: one_step_attention
def one_step_attention(a, s_prev):
"""
Performs one step of attention: Outputs a context vector computed as a dot product of the attention weights
"alphas" and the hidden states "a" of the Bi-LSTM.
Arguments:
a -- hidden state output of the Bi-LSTM, numpy-array of shape (m, Tx, 2*n_a)
s_prev -- previous hidden state of the (post-attention) LSTM, numpy-array of shape (m, n_s)
Returns:
context -- context vector, input of the next (post-attetion) LSTM cell
"""
### START CODE HERE ###
# Use repeator to repeat s_prev to be of shape (m, Tx, n_s) so that you can concatenate it with all hidden states "a" (≈ 1 line)
s_prev = repeator(s_p