》》欢迎 点赞,留言,收藏加关注《《
4. 配置模型
接下来是选择模型、配置模型参数,建议先阅读深度学习经典模型的文章(见本专栏补充文章:卷积神经网路),便于快速掌握深度学习模型的相关知识。
(1)选择模型
本案例将采用LeNet模型来训练MNIST手写数字模型,LeNet是一个经典卷积神经网络模型,结构简单,针对MNIST这种简单的数据集可达到比较好的效果,LeNet模型的原理介绍请见文章(见本专栏补充文章:CNN经典模型—LeNet),网络结构图如下:
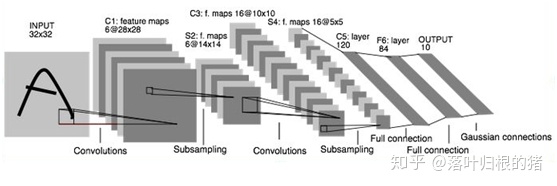
(2)设置参数
在训练模型时,一般要设置的参数有:
step_cnt=10000 # 训练模型的迭代步数
batch_size = 100 # 每次迭代批量取样本数据的量
learning_rate = 0.001 # 学习率
除此之外还有卷积层权重和偏置、池化层权重、全联接层权重和偏置、优化函数等等,根据模型需要进行设置。
6. 训练模型
接下来便是根据选择好的模型,构建网络,然后开始训练。
(1)构建模型
本案例按照LeNet的网络模型结构,构建网络模型,网络结果如下
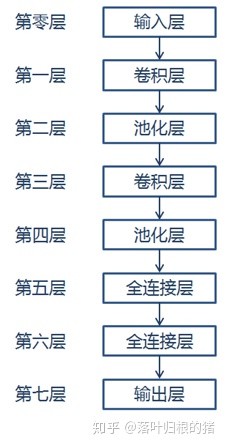
代码如下:
# 训练数据,占位符
x = tf.placeholder("float", shape=[None, 784])
# 训练的标签数据,占位符
y_ = tf.placeholder("float", shape=[None, 10])
# 将样本数据转为28x28
x_image = tf.reshape(x, [-1, 28, 28, 1])
# 保留概率,用于 dropout 层
keep_prob = tf.placeholder(tf.float32)
# 第一层:卷积层
# 卷积核尺寸为5x5,通道数为1,深度为32,移动步长为1,采用ReLU激励函数
conv1_weights = tf.get_variable("conv1_weights", [5, 5, 1, 32], initializer=tf.truncated_normal_initializer(stddev=0.1))
conv1_biases = tf.get_variable("conv1_biases", [32], initializer=tf.constant_initializer(0.0))
conv1 = tf.nn.conv2d(x_image, conv1_weights, strides=[1, 1, 1, 1], padding='SAME')
relu1 = tf.nn.relu(tf.nn.bias_add(conv1, conv1_biases))
# 第二层:最大池化层
# 池化核的尺寸为2x2,移动步长为2,使用全0填充
pool1 = tf.nn.max_pool(relu1, ksize=[1, 2, 2, 1], strides=[1, 2, 2, 1], padding='SAME')
# 第三层:卷积层
# 卷积核尺寸为5x5,通道数为32,深度为64,移动步长为1,采用ReLU激励函数
conv2_weights = tf.get_variable("conv2_weights", [5, 5, 32, 64], initializer=tf.truncated_normal_initializer(stddev=0.1))
conv2_biases = tf.get_variable("conv2_biases", [64], initializer=tf.constant_initializer(0.0))
conv2 = tf.nn.conv2d(pool1, conv2_weights, strides=[1, 1, 1, 1], padding='SAME')
relu2 = tf.nn.relu(tf.nn.bias_add(conv2, conv2_biases))
# 第四层:最大池化层
# 池化核尺寸为2x2, 移动步长为2,使用全0填充
pool2 = tf.nn.max_pool(relu2, ksize=[1, 2, 2, 1], strides=[1, 2, 2, 1], padding='SAME')
# 第五层:全连接层
fc1_weights = tf.get_variable("fc1_weights", [7 * 7 * 64, 1024],
initializer=tf.truncated_normal_initializer(stddev=0.1))
fc1_baises = tf.get_variable("fc1_baises", [1024], initializer=tf.constant_initializer(0.1))
pool2_vector = tf.reshape(pool2, [-1, 7 * 7 * 64])
fc1 = tf.nn.relu(tf.matmul(pool2_vector, fc1_weights) + fc1_baises)
# Dropout层(即按keep_prob的概率保留数据,其它丢弃),以防止过拟合
fc1_dropout = tf.nn.dropout(fc1, keep_prob)
# 第六层:全连接层
fc2_weights = tf.get_variable("fc2_weights", [1024, 10],
initializer=tf.truncated_normal_initializer(stddev=0.1)) # 神经元节点数1024, 分类节点10
fc2_biases = tf.get_variable("fc2_biases", [10], initializer=tf.constant_initializer(0.1))
fc2 = tf.matmul(fc1_dropout, fc2_weights) + fc2_biases
# 第七层:输出层
y_conv = tf.nn.softmax(fc2)
(2)训练模型
在训练模型时,需要选择优化器,也就是说要告诉模型以什么策略来提升模型的准确率,一般是选择交叉熵损失函数,然后使用优化器在反向传播时最小化损失函数,从而使模型的质量在不断迭代中逐步提升。
代码如下:
# 定义交叉熵损失函数
# y_ 为真实标签
cross_entropy = tf.reduce_mean(-tf.reduce_sum(y_ * tf.log(y_conv), reduction_indices=[1]))
# 选择优化器,使优化器最小化损失函数
train_step = tf.train.AdamOptimizer(learning_rate).minimize(cross_entropy)
# 返回模型预测的最大概率的结果,并与真实值作比较
correct_prediction = tf.equal(tf.argmax(y_conv, 1), tf.argmax(y_, 1))
# 用平均值来统计测试准确率
accuracy = tf.reduce_mean(tf.cast(correct_prediction, tf.float32))
# 训练模型
saver=tf.train.Saver()
with tf.Session() as sess:
tf.global_variables_initializer().run()
for step in range(step_cnt):
batch = mnist.train.next_batch(batch_size)
if step % 100 == 0:
# 每迭代100步进行一次评估,输出结果,保存模型,便于及时了解模型训练进展
train_accuracy = accuracy.eval(feed_dict={x: batch[0], y_: batch[1], keep_prob: 1.0})
print("step %d, training accuracy %g" % (step, train_accuracy))
saver.save(sess,model_dir+'/my_mnist_model.ctpk',global_step=step)
train_step.run(feed_dict={x: batch[0], y_: batch[1], keep_prob: 0.8})
# 使用测试数据测试准确率
print("test accuracy %g" % accuracy.eval(feed_dict={x: mnist.test.images, y_: mnist.test.labels, keep_prob: 1.0}))