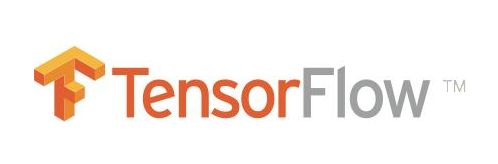
自定义损失函数/层
1. 自定义损失函数:
只需要将model.compile()中的loss参数更改为自定义的损失函数即可。
自定义损失函数可以直接调用tf下数学相关的api进行数学运算。
# 自定义损失函数
def customized_mse(y_true, y_pred):
return tf.reduce_mean(tf.square(y_pred - y_true))
# 模型构建
model = keras.Sequential([
keras.layers.Dense(30, activation="relu", input_shape=x_train_all.shape[1:]),
keras.layers.Dense(1),
])
# 查看模型结构
model.summary()
# 模型配置编译
model.compile(loss = customized_mse ,optimizer = "adam", metrics=["accuracy", "mean_squared_error"])
# 定义所需的callbacks
callbacks = [keras.callbacks.EarlyStopping(patience=5, min_delta=1e-3)]
# 模型训练(fit为拟合之意)
history = model.fit(x_train_scaled, y_train,
validation_data = (x_valid_scaled, y_valid),
epochs = 100,
callbacks = callbacks)
2. 自定义layer:
使用函数式方法调用layer
layer = tf.keras.layers.Dense(100, activation = "relu", input_shape =(None, 5))
layer(tf.zeros([10,5]))
<tf.Tensor: shape=(10, 100), dtype=float32, numpy= array([[0., 0., 0., 0., 0., 0., 0., 0., 0., 0., 0., 0., 0., 0., 0., 0., 0., 0., 0., 0., 0., 0., 0., 0., 0., 0., 0., 0., 0., 0., 0., 0., 0., 0., 0., 0., 0., 0., 0., 0., 0., 0., 0., 0., 0., 0., 0., 0., 0., 0., 0., 0., 0., 0., 0., 0., 0., 0., 0., 0., 0., 0., 0., 0., 0., 0., 0., 0., 0., 0., 0., 0., 0., 0., 0., 0., 0., 0., 0., 0., 0., 0., 0., 0., 0., 0., 0., 0., 0., 0., 0., 0., 0., 0., 0., 0., 0., 0., 0., 0.],......
layer常用方法:
layer.variables
# 1. keral:权重项
layer.variables[0]
# 2. bias:偏置项
layer.variables[1]
[<tf.Variable 'dense_6/kernel:0' shape=(5, 100) dtype=float32, numpy=
array([[]],dtype=float32)>,
<tf.Variable 'dense_6/bias:0' shape=(100,) dtype=float32, numpy=
array([[]]dtype=float32)>]
# 获取可训练的参数
layer.trainable_variables
help(layer)
使用子类自定义layer层
# customized dense layer.
class CustomizedDenseLayer(keras.layers.Layer):
def __init__(self, units, activation=None, **kwargs):
self.units = units
self.activation = keras.layers.Activation(activation)
super(CustomizedDenseLayer, self).__init__(**kwargs)
# initialize data
def build(self, input_shape):
"""构建所需要的参数"""
# x * w + b. input_shape:[None, a] w:[a, b] output_shape:[None, b]
self.kernel = self.add_weight(name= 'kernel',
shape = (input_shape[1], self.units),
initializer = 'uniform',
trainable = True)
self.bias = self.add_weight(name = 'bias',
shape = (self.units),
initializer = 'zeros',
trainable = True)
super(CustomizedDenseLayer, self).build(input_shape)
def call(self, x):
"""完整的正向计算"""
return self.activation(x @ self.kernel + self.bias)
model = keras.Sequential([
CustomizedDenseLayer(30, activation="relu", input_shape=x_train_all.shape[1:]),
CustomizedDenseLayer(1),
customized_softplus,
# keras.layers.Dense(1, activation="softplus"),
# keras.layers.Dense(1), keras.layers.Activation('softplus'),
])
model.summary()
model.compile(loss = "mse" ,optimizer = "adam", metrics=["accuracy", "mean_squared_error"])
callbacks = [keras.callbacks.EarlyStopping(patience=5, min_delta=1e-3)]
super()
继承父类的方法而无需知道父类的确切名称,此方法还常用于多重继承。
lambda函数自定义layer
# tf.nn.softplus : log(1+e^x)
customized_softplus = keras.layers.Lambda(lambda x:tf.nn.softplus(x))
print(customized_softplus([-10.,-5.,0.,5.,10.]))