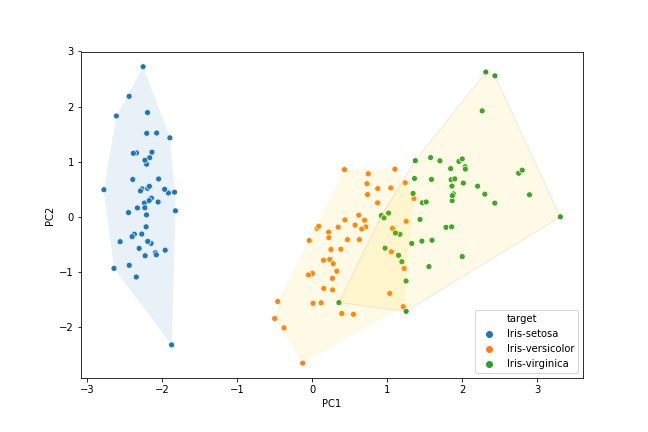
原文地址
Principal Component Analysis (PCA) with Python
导入需要的模块
import matplotlib.pyplot as plt
import pandas as pd
import seaborn as sns
import numpy as np
from sklearn.preprocessing import StandardScaler
from sklearn.decomposition import PCA
读入数据
数据获取连接 https://archive.ics.uci.edu/ml/machine-learning-databases/iris/iris.data
df = pd.read_csv("Desktop/Python_GUI/Tkinter/iris.csv")
df.head()
Out[8]:
sepal length sepal width petal lenght petal width target
0 5.1 3.5 1.4 0.2 Iris-setosa
1 4.9 3.0 1.4 0.2 Iris-setosa
2 4.7 3.2 1.3 0.2 Iris-setosa
3 4.6 3.1 1.5 0.2 Iris-setosa
4 5.0 3.6 1.4 0.2 Iris-setosa
主成分分析
df1 = df.loc[:,df.columns[0:4]]
scaler = StandardScaler()
scaler.fit(df1)
scaled_df = scaler.transform(df1)
pca = PCA(n_components = 4)
pca.fit(scaled_df)
x_pca = pca.transform(scaled_df)
pca.explained_variance_ratio_
plt.bar(x = range(1,5),height=percent_variance,tick_label=["PC" + str(i) for i in range(1,5)])
plt.ylabel('Percentate of Variance Explained')
plt.xlabel('Principal Component')
plt.title('PCA Scree Plot')
plt.savefig("1.jpg")
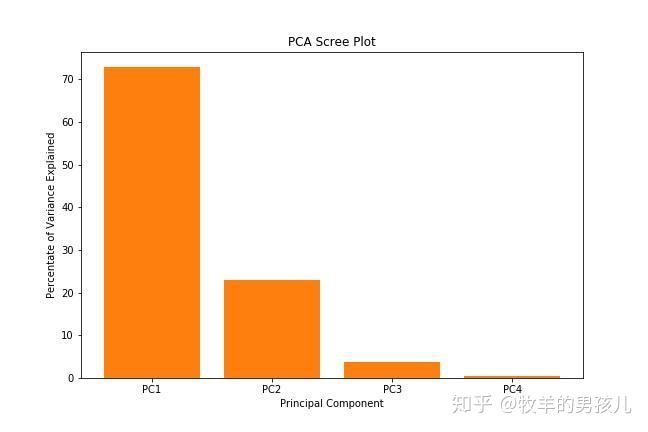
df2 = pd.DataFrame(data=x_pca,columns=["PC"+str(i) for i in range(1,5)])
df3 = pd.concat([df2,df['target']],axis=1)
plt.figure(figsize=(9,6))
sns.scatterplot(x="PC1",y="PC2",hue='target',data=df3)
plt.xlabel("PC1")
plt.ylabel("PC2")
plt.title("PC1 VS PC2")
plt.savefig("2.jpg")
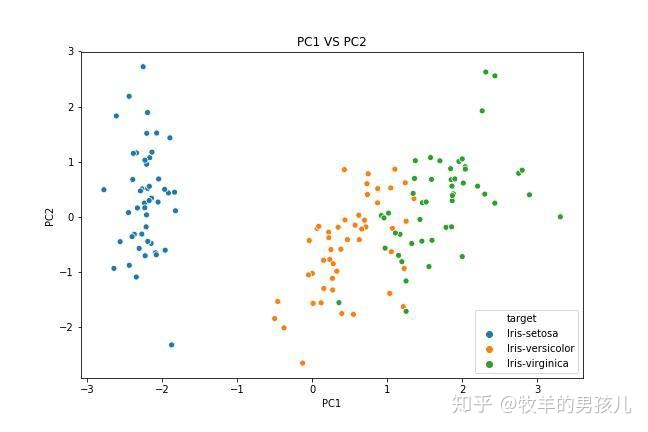
为不同的品种绘制边界
代码来自知乎文章 数据分析最有用的25个 Matplotlib图(一) 用到的代码自己还看不懂
from matplotlib import patches
from scipy.spatial import ConvexHull
#这个函数的代码自己还看不懂
def encircle(x,y, ax=None, **kw):
if not ax: ax=plt.gca()
p = np.c_[x,y]
hull = ConvexHull(p)
poly = plt.Polygon(p[hull.vertices,:], **kw)
ax.add_patch(poly)
df3_1 = df3.loc[df3.target == df3.target.unique()[0],:]
df3_2 = df3.loc[df3.target == df3.target.unique()[1],:]
df3_3 = df3.loc[df3.target == df3.target.unique()[2],:]
plt.figure(figsize=(9,6))
sns.scatterplot(x="PC1",y="PC2",hue='target',data=df3)
encircle(df3_1.PC1,df3_1.PC2,alpha=0.1)
encircle(df3_2.PC1,df3_2.PC2,fc = "gold", alpha=0.1)
encircle(df3_3.PC1,df3_3.PC2,ec = "firebrick",fc = "gold", alpha=0.1)
plt.savefig("3.jpg")
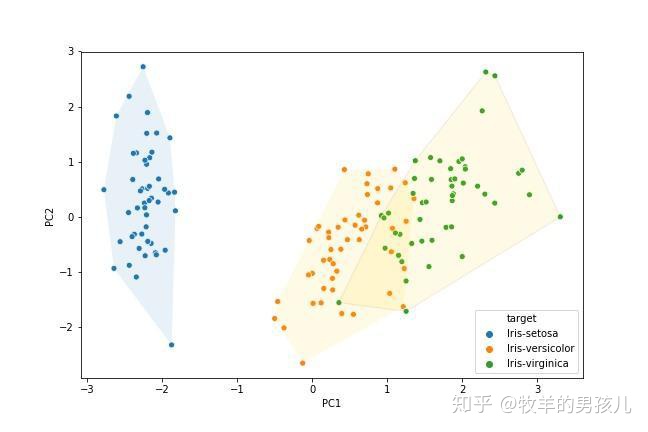
参考文章
- https://datascienceplus.com/principal-component-analysis-pca-with-python/
- https://scikit-learn.org/stable/auto_examples/decomposition/plot_pca_iris.html
- https://www.jianshu.com/p/4528aaa6dc48
- https://scikit-learn.org/stable/auto_examples/decomposition/plot_pca_iris.html
- https://www.kindsonthegenius.com/2019/01/12/principal-components-analysispca-in-python-step-by-step/
- https://jakevdp.github.io/PythonDataScienceHandbook/05.09-principal-component-analysis.html