model = tf.keras.Sequential()
model.add(ly.Flatten(input_shape=(28, 28)))
model.add(ly.Dense(64,activation='relu',kernel_initializer=tf.keras.initializers.glorot_normal))
model.add(ly.Dense(32,activation='relu',kernel_regularizer=tf.keras
解决tensorflow2.0中出现的TypeError: get_config() missing 1 required positional argument: 'self',save过程中出错
最新推荐文章于 2024-06-22 14:53:57 发布
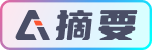