import geopandas as gpd
dist_buffer = 500
jy_12_poly_gdf = gpd.read_file(jy_12_split_file)
for i in range(100):
nei_poly = jy_12_poly_gdf[jy_12_poly_gdf.within(jy_12_poly_gdf[
【Python实现Select by Location】使用geopandas对多边形进行buffer筛选
最新推荐文章于 2024-07-28 08:00:00 发布
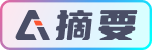