决策树的实现相对我这种新手比较难,参考了一篇文章 数据挖掘领域十大经典算法之—C4.5算法(超详细附代码)
首先贴上书上的相关内容,包括P94,一个预测拖欠贷款的数据集,以及根据数据生成的树。后面是P101,树生成的算法框架。
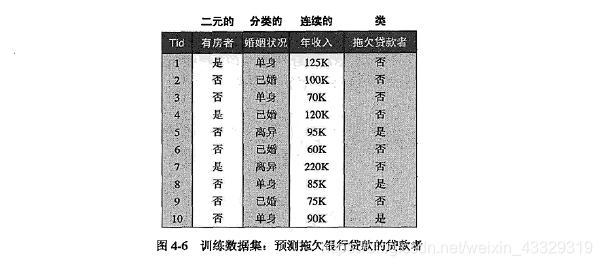
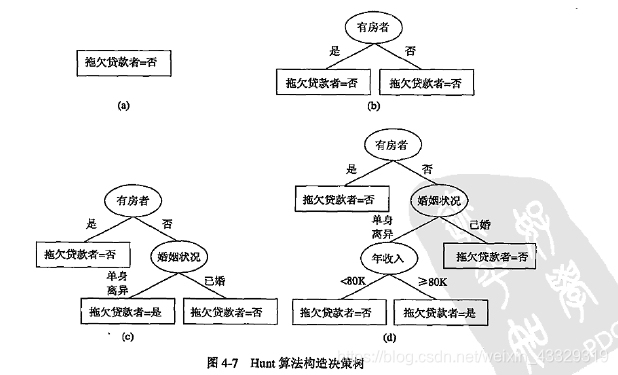
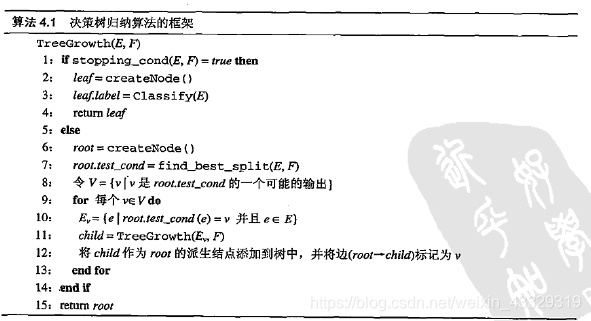
1、树类
1.1、参考
但是,他里面写的内容比较散乱,明显没有书本P101的框架明白,因此仅参考了他的‘树’类的写法,下面是他的原写法:
class Tree(object):
def __init__(self,node_type,Class = None, feature = None):
self.node_type = node_type # 节点类型(internal或leaf)
self.dict = {} # dict的键表示特征Ag的可能值ai,值表示根据ai得到的子树
self.Class = Class # 叶节点表示的类,若是内部节点则为none
self.feature = feature
# 表示当前的树即将由第feature个特征划分z(即第feature特征是使得当前树中信息增益最大的特征)
def add_tree(self,key,tree):
self.dict[key] = tree
def predict(self,features):
print(self.dict)
if self.node_type == 'leaf' or (features[self.feature] not in self.dict):
return self.Class
tree = self.dict.get(features[self.feature])
# print(tree.dict)
return tree.predict(features)
用一个例子(P94)测试一下效果:
a=Tree('internal',None,0)
one1=Tree('leaf','No',1)
one2=Tree('internal',None,1)
a.add_tree('Yes',one1)
a.add_tree('No',one2)
two1=Tree('leaf','No',2)
two2=Tree('internal',None,2)
one2.add_tree('married',two1)
one2.add_tree('single',two2)
three1=Tree('leaf','No',3)
three2=Tree('leaf','Yes',3)
two2.add_tree('<80K',three1)
two2.add_tree('>80K',three2)
a.predict(['No','single','>80K'])
:结果如下:
{'Yes': <__main__.Tree object at 0x0000000005131390>, 'No': <__main__.Tree object at 0x0000000005131400>}
{'married': <__main__.Tree object at 0x0000000005131278>, 'single': <__main__.Tree object at 0x0000000005113CF8>}
{'<80K': <__main__.Tree object at 0x0000000005113D30>, '>80K': <__main__.Tree object at 0x00000000051026A0>}
{}
'Yes'
1.2、改写
原写法花了不少时间才弄明白feature
是指针,参考书本P101的框架,发现并不需要Class
参数。并且,predict
中终止条件属性错误应该是Error
。
按照P101的框架需要改写一下(更加清晰):
class Node():
def __init__(self,label=None,test_cond = None):
self.label = label # 叶节点表示的类,内部节点为None
self.test_cond = test_cond # 当前的测试特征,叶节点为None
self.dict = {} # dict的键表示当前测试特征的可能值v,值是对应的子树
def add_child(self,key,child):
self.dict[key] = child
def predict(self,F): #递归预测
# print(self.dict) #测试用
if self.label: #递归的终止条件(到达叶节点)
return self.label
# 输入特征值错误处理
if F[self.test_cond] not in self.dict:
return 'feature Error: %s'% F[self.test_cond]
#由当前的特征值test_cond,进入下一级
child = self.dict.get(F[self.test_cond])
return child.predict(F)#递归
2、main
2.1、测试
P101这个框架是递归的方式,由于对递归不太了解,首先对这个框架进行了简单的代码测试:
def Test(L):
if len(L)==1:
return Node('hello')
root=Node()
V=L[0]
root.test_cond=V
L=L[1:]
child=Test(L)
root.add_child(V,child)
return root
L=list(range(10))
root=Test(L)
root.predict(L)
结果不错:
{0: <__main__.Node object at 0x0000000005137C50>}
{1: <__main__.Node object at 0x00000000051379B0>}
{2: <__main__.Node object at 0x0000000005137D68>}
{3: <__main__.Node object at 0x0000000005137DD8>}
{4: <__main__.Node object at 0x0000000005137E48>}
{5: <__main__.Node object at 0x0000000005137DA0>}
{6: <__main__.Node object at 0x0000000005137E10>}
{7: <__main__.Node object at 0x0000000005137E80>}
{8: <__main__.Node object at 0x0000000005137EF0>}
{}
'hello'
2.1、代码
按照书上框架写下去真是非常清爽,就是Gini计算时候,数组比较麻烦,还用上了reduce
,还是DataFrame好。然后本想对连续属性进行处理和计算一下增益,最后权衡一下,还是抓紧赶进度吧。
import numpy as np
from functools import reduce
#E是训练记录集,F是属性集,在程序内部使用数字索引的,F仅为了便于理解
#树分裂的停止条件
def stopping_cond(E,F):
stop=False
if len(F)==0 or len(set(E[:,-1]))==1:
stop=True
return stop
#确定叶节点类标号
def Classify(E):
labels=E[:,-1]
class_count=[(i,len(list(filter(lambda x:x==i,labels)))) for i in set(labels)]
(max_class,max_len)=max(class_count,key=lambda x:x[1])
return max_class
#不纯度量
def Gini(T):
#提取属性测试列
V=list(set(T[:,0]))
#提取全部标签
labels=list(set(T[:,1]))
count_all=len(T)
gini=0
#按照当前属性将T进行分割
split=[(v,list(filter(lambda x:x[0]==v,T))) for v in V ]
for s in range(len(split)):
#按照标签进行数量统计
class_count=[len(list(filter(lambda x:x[1]==i,split[s][1]))) for i in labels]
c=len(split[s][1])
#计算Gini
gini_single=1-reduce(lambda x,y:(x/c)**2+(y/c)**2,class_count)
gini+=gini_single*c/count_all
return gini
#选择最优属性,作为本次划分条件
def find_best_split(E,F):
E=E[:-1]#弃掉label列
gini=[(i,Gini(E[:,[i,-1]])) for i in range(len(F))]
(best_split,min_gini)=min(gini,key=lambda x:x[1])
return best_split
def TreeGrowth(E,F):
if stopping_cond(E,F)==True:
leaf=Node()
leaf.label=Classify(E)
return leaf
else:
root=Node()
root.test_cond=find_best_split(E,F)
# print(root.test_cond,'--') #测试
V=list(set(E[:,root.test_cond]))
for v in V:
Ev=E[E[:,root.test_cond]==v]
child=TreeGrowth(Ev,F)
root.add_child(v,child)
return root
测试数据,还是P94页例子的数据。从测试代码print(root.test_cond,'--')
,得到的结果是[1,0,0,2],因为对于婚姻一项没有合并,与书本的书略有不同。
E=np.array([['Yes','single','>=80K','No'],
['No','married','>=80K','No'],
['No','single','<80K','No'],
['Yes','married','>=80K','No'],
['No','Divorced','>=80K','Yes'],
['No','married','<80K','No'],
['Yes','Divorced','>=80K','No'],
['No','single','>=80K','Yes'],
['No','married','<80K','No'],
['No','single','>=80K','Yes']])
F=['house','marital','income']
print(E[:,0])
Tree=TreeGrowth(E,F)
Tree.predict(['No','single','>=80K'])
使用负类进行测试,能得到全部的树,可以看到第一次分裂,婚姻属性是多路划分:
{'married': <__main__.Node object at 0x0000000009C1C518>, 'Divorced': <__main__.Node object at 0x0000000009C1CFD0>, 'single': <__main__.Node object at 0x0000000009C1C470>}
{'Yes': <__main__.Node object at 0x0000000009C1CDD8>, 'No': <__main__.Node object at 0x0000000009C1CEB8>}
{'>=80K': <__main__.Node object at 0x0000000009C1CC88>, '<80K': <__main__.Node object at 0x0000000009C1C3C8>}
{}
'Yes'