对抗性验证(Adversarial Validation)的作用
生成与待分类数据集同分布的新数据集并当作验证集,这样子训练出来的模型在待分类数据集中的分类效果更好。
AUC简介
最后得到的模型的对新数据的预测结果的AUC值越大,说明这个分类模型的分类能力越好。
项目详解
代码:
import numpy as np # linear algebra
import pandas as pd # data processing, CSV file I/O (e.g. pd.read_csv)
import matplotlib.pyplot as plt
import seaborn as sns
import lightgbm as lgb
from sklearn.model_selection import train_test_split
from sklearn import model_selection, preprocessing, metrics
import warnings
import datetime
warnings.filterwarnings("ignore")
import os
import gc
print(os.listdir("../input"))
print(os.listdir("../input/microsoft-malware-prediction"))
print(os.listdir("../input/malware-feature-engineering-full-train-and-test/"))
输出:
[‘microsoft-malware-prediction’, ‘malware-feature-engineering-full-train-and-test’]
[‘train.csv’, ‘sample_submission.csv’, ‘test.csv’]
[’__output__.json’, ‘custom.css’, ‘new_test.csv’, ‘__results__.html’, ‘new_train.csv’]
columns_to_use = ['ProductName', 'EngineVersion', 'AppVersion', 'AvSigVersion', 'IsBeta',
'RtpStateBitfield', 'IsSxsPassiveMode', 'DefaultBrowsersIdentifier',
'AVProductStatesIdentifier', 'AVProductsInstalled', 'AVProductsEnabled',
'HasTpm', 'CountryIdentifier', 'CityIdentifier',
'OrganizationIdentifier', 'GeoNameIdentifier',
'LocaleEnglishNameIdentifier', 'Platform', 'Processor', 'OsVer',
'OsBuild', 'OsSuite', 'OsPlatformSubRelease', 'OsBuildLab',
'SkuEdition', 'IsProtected', 'AutoSampleOptIn', 'SMode',
'IeVerIdentifier', 'SmartScreen', 'Firewall', 'UacLuaenable',
'Census_MDC2FormFactor', 'Census_DeviceFamily',
'Census_OEMNameIdentifier', 'Census_OEMModelIdentifier',
'Census_ProcessorCoreCount', 'Census_ProcessorManufacturerIdentifier',
'Census_ProcessorModelIdentifier', 'Census_ProcessorClass',
'Census_PrimaryDiskTotalCapacity', 'Census_PrimaryDiskTypeName',
'Census_SystemVolumeTotalCapacity', 'Census_HasOpticalDiskDrive',
'Census_TotalPhysicalRAM', 'Census_ChassisTypeName',
'Census_InternalPrimaryDiagonalDisplaySizeInInches',
'Census_InternalPrimaryDisplayResolutionHorizontal',
'Census_InternalPrimaryDisplayResolutionVertical',
'Census_PowerPlatformRoleName', 'Census_InternalBatteryType',
'Census_InternalBatteryNumberOfCharges', 'Census_OSVersion',
'Census_OSArchitecture', 'Census_OSBranch', 'Census_OSBuildNumber',
'Census_OSBuildRevision', 'Census_OSEdition', 'Census_OSSkuName',
'Census_OSInstallTypeName', 'Census_OSInstallLanguageIdentifier',
'Census_OSUILocaleIdentifier', 'Census_OSWUAutoUpdateOptionsName',
'Census_IsPortableOperatingSystem', 'Census_GenuineStateName',
'Census_ActivationChannel', 'Census_IsFlightingInternal',
'Census_IsFlightsDisabled', 'Census_FlightRing',
'Census_ThresholdOptIn', 'Census_FirmwareManufacturerIdentifier',
'Census_FirmwareVersionIdentifier', 'Census_IsSecureBootEnabled',
'Census_IsWIMBootEnabled', 'Census_IsVirtualDevice',
'Census_IsTouchEnabled', 'Census_IsPenCapable',
'Census_IsAlwaysOnAlwaysConnectedCapable', 'Wdft_IsGamer',
'Wdft_RegionIdentifier']
new_train = pd.read_csv('../input/malware-feature-engineering-full-train-and-test/new_train.csv',
nrows=1000000, usecols = columns_to_use)
print(new_train.shape)
print(new_train.head())
输出:
(1000000, 80)
ProductName | EngineVersion | AppVersion | AvSigVersion | IsBeta | RtpStateBitfield | IsSxsPassiveMode | DefaultBrowsersIdentifier | AVProductStatesIdentifier | AVProductsInstalled | AVProductsEnabled | HasTpm | CountryIdentifier | CityIdentifier | OrganizationIdentifier | GeoNameIdentifier | LocaleEnglishNameIdentifier | Platform | Processor | OsVer | OsBuild | OsSuite | OsPlatformSubRelease | OsBuildLab | SkuEdition | IsProtected | AutoSampleOptIn | SMode | IeVerIdentifier | SmartScreen | Firewall | UacLuaenable | Census_MDC2FormFactor | Census_DeviceFamily | Census_OEMNameIdentifier | Census_OEMModelIdentifier | Census_ProcessorCoreCount | Census_ProcessorManufacturerIdentifier | Census_ProcessorModelIdentifier | Census_ProcessorClass | Census_PrimaryDiskTotalCapacity | Census_PrimaryDiskTypeName | Census_SystemVolumeTotalCapacity | Census_HasOpticalDiskDrive | Census_TotalPhysicalRAM | Census_ChassisTypeName | Census_InternalPrimaryDiagonalDisplaySizeInInches | Census_InternalPrimaryDisplayResolutionHorizontal | Census_InternalPrimaryDisplayResolutionVertical | Census_PowerPlatformRoleName | Census_InternalBatteryType | Census_InternalBatteryNumberOfCharges | Census_OSVersion | Census_OSArchitecture | Census_OSBranch | Census_OSBuildNumber | Census_OSBuildRevision | Census_OSEdition | Census_OSSkuName | Census_OSInstallTypeName | Census_OSInstallLanguageIdentifier | Census_OSUILocaleIdentifier | Census_OSWUAutoUpdateOptionsName | Census_IsPortableOperatingSystem | Census_GenuineStateName | Census_ActivationChannel | Census_IsFlightingInternal | Census_IsFlightsDisabled | Census_FlightRing | Census_ThresholdOptIn | Census_FirmwareManufacturerIdentifier | Census_FirmwareVersionIdentifier | Census_IsSecureBootEnabled | Census_IsWIMBootEnabled | Census_IsVirtualDevice | Census_IsTouchEnabled | Census_IsPenCapable | Census_IsAlwaysOnAlwaysConnectedCapable | Wdft_IsGamer | Wdft_RegionIdentifier | |
0 | 0 | 0 | 0 | 0 | 0 | 0 | 0 | -1 | 0 | 0 | 0 | 1 | 0 | 202.0 | 0 | 0 | 0 | 0 | 0 | 0 | 0 | 0 | 0 | 0 | 0 | 1.0 | 0 | 0.0 | 0 | -1 | 1.0 | 0 | 0 | 0 | 0 | 20832.0 | 4.0 | 0 | 0 | -1 | 476940.0 | 0 | 299451.0 | 0 | 4096.0 | 0 | 18.9 | 1440.0 | 900.0 | 0 | -1 | 4.294967e+09 | 0 | 0 | 0 | 0 | 0 | 0 | 0 | 0 | 0 | 0 | 0 | 0 | 0 | 0 | NaN | 0.0 | 0 | NaN | 0 | 2516.0 | 0 | NaN | 0.0 | 0 | 0 | 0.0 | 0.0 | 0 |
1 | 0 | 1 | 1 | 1 | 0 | 0 | 0 | -1 | 0 | 0 | 0 | 1 | 1 | 164.0 | 0 | 1 | 1 | 0 | 0 | 0 | 0 | 0 | 0 | 0 | 0 | 1.0 | 0 | 0.0 | 0 | -1 | 1.0 | 0 | 1 | 0 | 0 | 98328.0 | 4.0 | 0 | 1 | -1 | 476940.0 | 0 | 102385.0 | 0 | 4096.0 | 1 | 13.9 | 1366.0 | 768.0 | 1 | -1 | 1.000000e+00 | 1 | 0 | 0 | 0 | 1 | 0 | 0 | 1 | 1 | 1 | 0 | 0 | 1 | 0 | NaN | 0.0 | 1 | NaN | 0 | 1767.0 | 0 | NaN | 0.0 | 0 | 0 | 0.0 | 0.0 | 1 |
2 | 0 | 0 | 0 | 2 | 0 | 0 | 0 | -1 | 0 | 0 | 0 | 1 | 2 | 685.0 | 0 | 2 | 2 | 0 | 0 | 0 | 0 | 1 | 0 | 0 | 1 | 1.0 | 0 | 0.0 | 0 | 0 | 1.0 | 0 | 0 | 0 | 1 | 2.0 | 4.0 | 0 | 2 | -1 | 114473.0 | 1 | 113907.0 | 0 | 4096.0 | 0 | 21.5 | 1920.0 | 1080.0 | 0 | -1 | 4.294967e+09 | 0 | 0 | 0 | 0 | 0 | 1 | 1 | 0 | 2 | 2 | 1 | 0 | 0 | 1 | NaN | 0.0 | 0 | NaN | 1 | 190.0 | 0 | NaN | 0.0 | 0 | 0 | 0.0 | 0.0 | 2 |
3 | 0 | 0 | 0 | 3 | 0 | 0 | 0 | -1 | 0 | 0 | 0 | 1 | 3 | 20.0 | -1 | 3 | 3 | 0 | 0 | 0 | 0 | 0 | 0 | 0 | 0 | 1.0 | 0 | 0.0 | 0 | 1 | 1.0 | 0 | 0 | 0 | 2 | 171.0 | 4.0 | 0 | 3 | -1 | 238475.0 | 2 | 227116.0 | 0 | 4096.0 | 2 | 18.5 | 1366.0 | 768.0 | 0 | -1 | 4.294967e+09 | 2 | 0 | 0 | 0 | 2 | 0 | 0 | 0 | 3 | 3 | 1 | 0 | 0 | 1 | NaN | 0.0 | 0 | NaN | 2 | 33.0 | 0 | NaN | 0.0 | 0 | 0 | 0.0 | 0.0 | 2 |
4 | 0 | 0 | 0 | 4 | 0 | 0 | 0 | -1 | 0 | 0 | 0 | 1 | 4 | 15.0 | -1 | 4 | 4 | 0 | 0 | 0 | 0 | 1 | 0 | 0 | 1 | 1.0 | 0 | 0.0 | 0 | 0 | 1.0 | 0 | 1 | 0 | 2 | 2263.0 | 4.0 | 0 | 4 | -1 | 476940.0 | 0 | 101900.0 | 0 | 6144.0 | 3 | 14.0 | 1366.0 | 768.0 | 1 | 0 | 0.000000e+00 | 3 | 0 | 0 | 0 | 3 | 1 | 1 | 2 | 1 | 1 | 1 | 0 | 0 | 0 | 0.0 | 0.0 | 0 | 0.0 | 2 | 124.0 | 0 | 0.0 | 0.0 | 0 | 0 | 0.0 | 0.0 | 3 |
cat_features = ['PuaMode']
new_test = pd.read_csv('../input/malware-feature-engineering-full-train-and-test/new_test.csv',
nrows=1000000, usecols = columns_to_use)
print(new_test.shape)
输出:
(1000000, 80)
new_train['target'] = 0
new_test['target'] = 1
new_train = pd.concat([new_train, new_test], axis =0)
target = new_train['target'].values
del new_train['target']
del new_test
new_train, new_val, target_train, target_val = train_test_split(new_train, target,
test_size=0.2, random_state=42)
param = {'num_leaves': 200,
'min_data_in_leaf': 60,
'objective':'binary',
'max_depth': -1,
'learning_rate': 0.1,
"min_child_samples": 20,
"boosting": "gbdt",
"feature_fraction": 0.8,
"bagging_freq": 1,
"bagging_fraction": 0.8 ,
"bagging_seed": 17,
"metric": 'auc',
"lambda_l1": 0.1,
"verbosity": -1,
"n_jobs":-1}
new_train = lgb.Dataset(new_train.values, label=target_train)
new_val = lgb.Dataset(new_val.values, label=target_val)
num_round = 1000
clf = lgb.train(param, new_train, num_round, valid_sets = [new_train, new_val], verbose_eval=10, early_stopping_rounds = 25)
Training until validation scores don’t improve for 25 rounds.
[10] training’s auc: 0.977506 valid_1’s auc: 0.977521
[20] training’s auc: 0.978298 valid_1’s auc: 0.978195
[30] training’s auc: 0.978955 valid_1’s auc: 0.978624
[40] training’s auc: 0.979589 valid_1’s auc: 0.979024
[50] training’s auc: 0.980195 valid_1’s auc: 0.979331
[60] training’s auc: 0.980738 valid_1’s auc: 0.979562
[70] training’s auc: 0.981254 valid_1’s auc: 0.979729
[80] training’s auc: 0.981701 valid_1’s auc: 0.979824
[90] training’s auc: 0.982138 valid_1’s auc: 0.979934
[100] training’s auc: 0.982507 valid_1’s auc: 0.979991
[110] training’s auc: 0.98287 valid_1’s auc: 0.980026
[120] training’s auc: 0.983184 valid_1’s auc: 0.980058
[130] training’s auc: 0.98349 valid_1’s auc: 0.980061
[140] training’s auc: 0.983802 valid_1’s auc: 0.980066
[150] training’s auc: 0.984118 valid_1’s auc: 0.980061
[160] training’s auc: 0.984421 valid_1’s auc: 0.980064
Early stopping, best iteration is:
[136] training’s auc: 0.983674 valid_1’s auc: 0.980071
通过对抗性验证之后,可以得到为生成与test.csv同分布数据集的原数据集特征的贡献度的排名,并以图形表示出来。
import matplotlib.pyplot as plt
import seaborn as sns
import warnings
warnings.simplefilter(action='ignore', category=FutureWarning)
feature_imp = pd.DataFrame(sorted(zip(clf.feature_importance(),columns_to_use), reverse=True), columns=['Value','Feature'])
plt.figure(figsize=(20, 10))
sns.barplot(x="Value", y="Feature", data=feature_imp.sort_values(by="Value", ascending=False))
plt.title('LightGBM Features (avg over folds)')
plt.tight_layout()
plt.show()
plt.savefig('lgbm_importances-01.png')
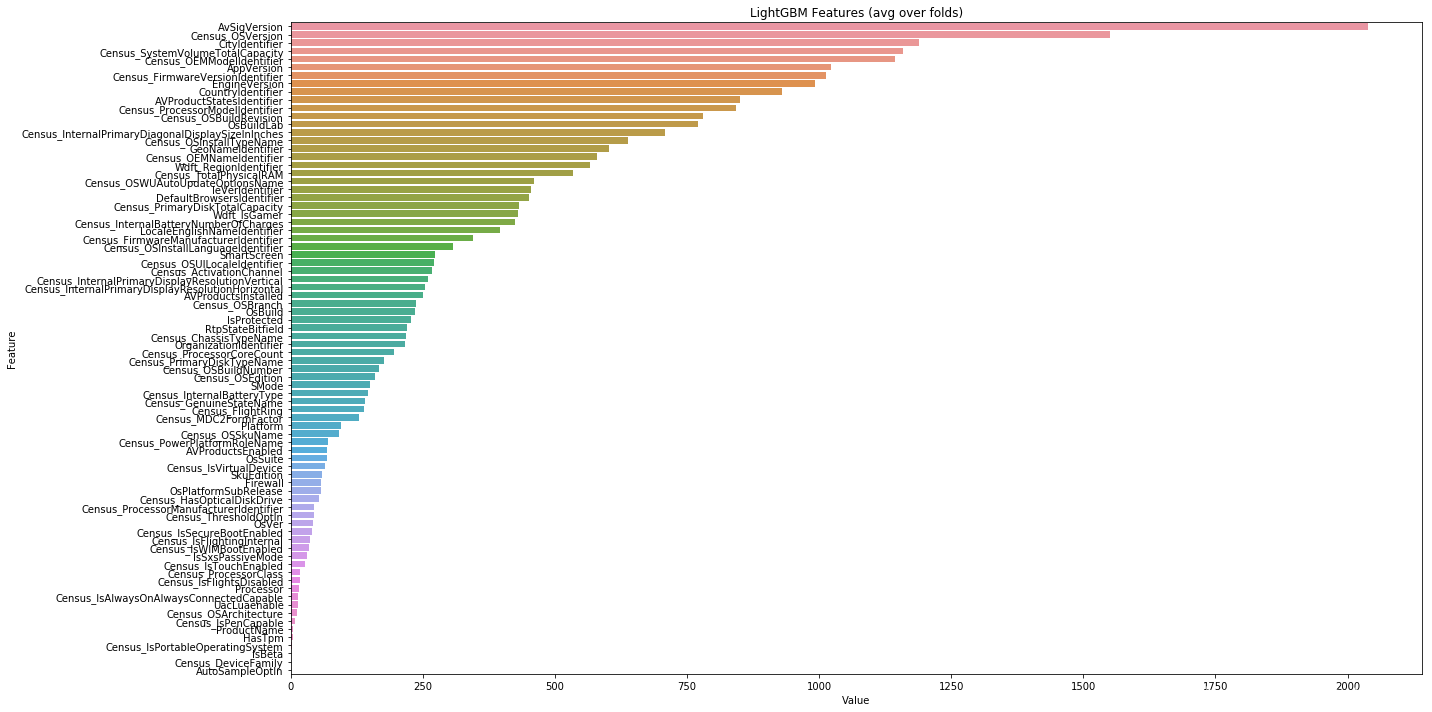
最后,我们可以根据这个 样本重要性 排行榜来选择样本作为验证集
如何利用这个排行榜:
在原始数据中,是存在许多缺失值的,有许多的值的命名也不规范(例如字符串型的特征值),那么,我们要选择哪些样本呢?这时候就可以通过这个排行榜。
举个例子:这个排行榜中的的第一名的特征是’AvSiaVersion’,我们把那些在这个特征上的值是缺失值的样本全部移除,从剩下的样本中挑选出验证集。