保存网络:
torch.save(网络, 网络名)
torch.save(网络.state_dict(), 网络名)
保存整个网络
,不需要再搭建结构;只保存网络参数
需要在搭建之前一样的网络结构,再将参数放进去。就好比前者是去饭店买来一碗色香俱全的酸菜鱼,后者是老板加什么调料,多少调料,煮多久都告诉你,你回家自己做,做完和直接买来的一样了。- 据说
只保存网络参数
会快一点哦。
提取网络:
torch.load(网络名)
网络.load_state_dict(torch.load(网络名))
以之前线性回归代码为例,用保存的网络,比较用两种方法提取的网络
import torch
import matplotlib.pyplot as plt
def save_all_net(net, net_name):
"""保存整个网络"""
torch.save(net, net_name)
def save_net_parameters(net, net_name):
"""只保存网络中的参数"""
torch.save(net.state_dict(), net_name)
def restore_net(net_name):
"""提取整个模型"""
net = torch.load(net_name)
return net
def restore_parameters(network, net_name):
"""提取网络中的参数"""
network.load_state_dict(torch.load(net_name))
x = torch.unsqueeze(torch.linspace(-1, 1, 100), dim=-1)
y = x.pow(2)
def orignal_net():
"""原始网络"""
net = torch.nn.Sequential(
torch.nn.Linear(1, 10),
torch.nn.ReLU(),
torch.nn.Linear(10, 1)
)
optimizer = torch.optim.SGD(net.parameters(), lr=0.05)
loss_funcation = torch.nn.MSELoss()
for epoch in range(100):
pridect = net(x)
loss = loss_funcation(pridect, y)
optimizer.zero_grad()
loss.backward()
optimizer.step()
plt.figure(1, figsize=(10, 3))
plt.subplot(131)
plt.title('Net1')
plt.scatter(x.data.numpy(), y.data.numpy())
plt.plot(x.data.numpy(), pridect.data.numpy(), 'r-', lw=5)
save_all_net(net, "net.pkl")
save_net_parameters(net, "net_params.pkl")
def read_all_net():
"""恢复提取整个的网络"""
net2 = restore_net("net.pkl")
pridect = net2(x)
plt.subplot(132)
plt.title('Net2')
plt.scatter(x.data.numpy(), y.data.numpy())
plt.plot(x.data.numpy(), pridect.data.numpy(), 'r-', lw=5)
def read_parameters():
"""恢复只提取参数的网络"""
net3 = torch.nn.Sequential(
torch.nn.Linear(1, 10),
torch.nn.ReLU(),
torch.nn.Linear(10, 1),
)
restore_parameters(net3, "net_params.pkl")
pridect = net3(x)
plt.subplot(133)
plt.title('Net3')
plt.scatter(x.data.numpy(), y.data.numpy())
plt.plot(x.data.numpy(), pridect.data.numpy(), 'r-', lw=5)
plt.show()
orignal_net()
read_all_net()
read_parameters()
可视化图:证明一样
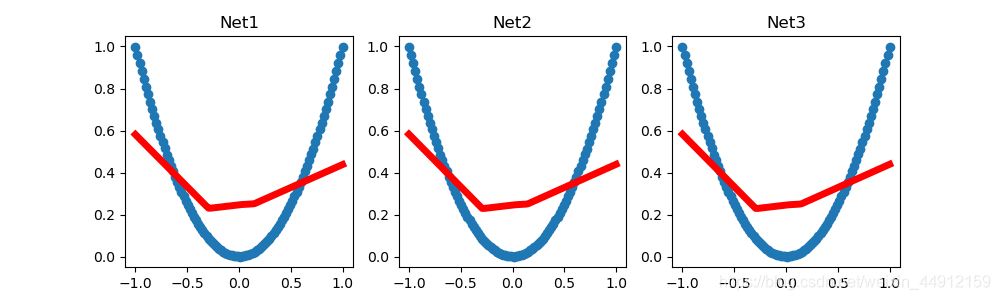