opencv学习日记——基于Hu矩的轮廓匹配
图像结果(达不到预期)
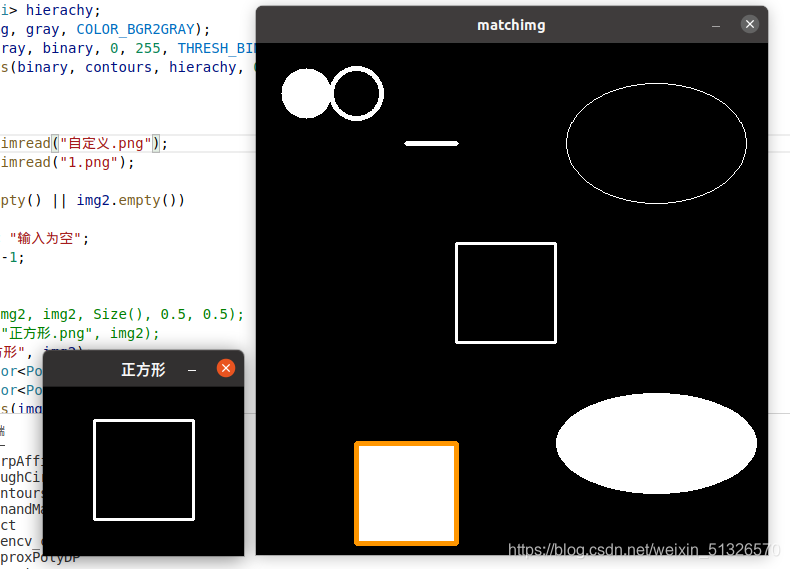
代码部分
#include <iostream>
#include <opencv2/opencv.hpp>
using namespace std;
using namespace cv;
void findcontours(Mat &img, vector<vector<Point>> &contours)
{
Mat gray, binary;
vector<Vec4i> hierachy;
cvtColor(img, gray, COLOR_BGR2GRAY);
threshold(gray, binary, 0, 255, THRESH_BINARY | THRESH_OTSU);
findContours(binary, contours, hierachy, 0, 2);
}
int main()
{
Mat img1 = imread("自定义.png");
Mat img2 = imread("1.png");
if (img1.empty() || img2.empty())
{
cout << "输入为空";
return -1;
}
imshow("正方形", img2);
vector<vector<Point>> contours1;
vector<vector<Point>> contours2;
findcontours(img1, contours1);
findcontours(img2, contours2);
Moments mm2 = moments(contours2[0]);
Mat hu2;
HuMoments(mm2, hu2);
for (int z = 0; z < contours1.size(); z++ )
{
Moments mm1 = moments(contours1[z]);
Mat hu1;
HuMoments(mm1, hu1);
double dist;
dist = matchShapes(hu1, hu2, 1, 0);
if (dist < 1)
{
cout << "yes";
drawContours(img1, contours1, z, Scalar(0, 150, 255), 3, 8);
}
imshow ("matchimg", img1);
waitKey(0);
return 0;
}
}