ndarray类属性
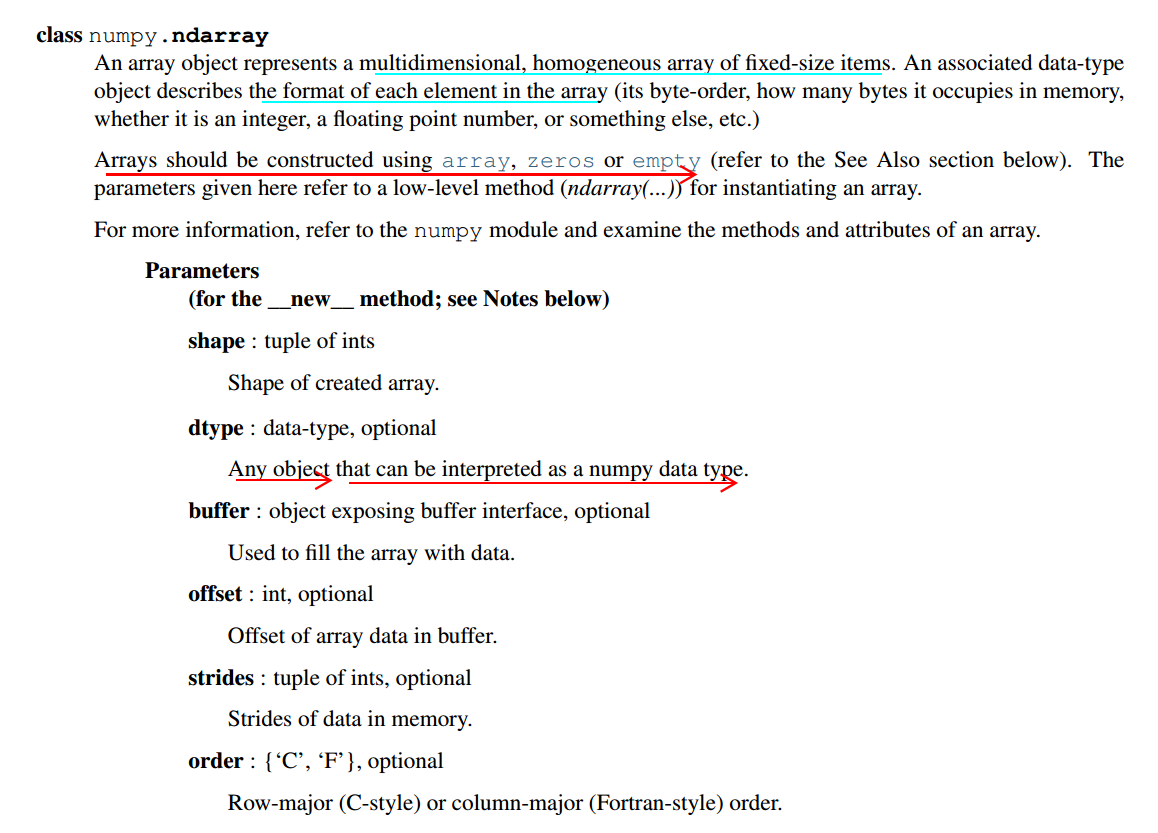
numpy的数据结构
All ndarrays are homogenous: every item takes up the same size block of memory, and all blocks are interpreted in exactly the same way.
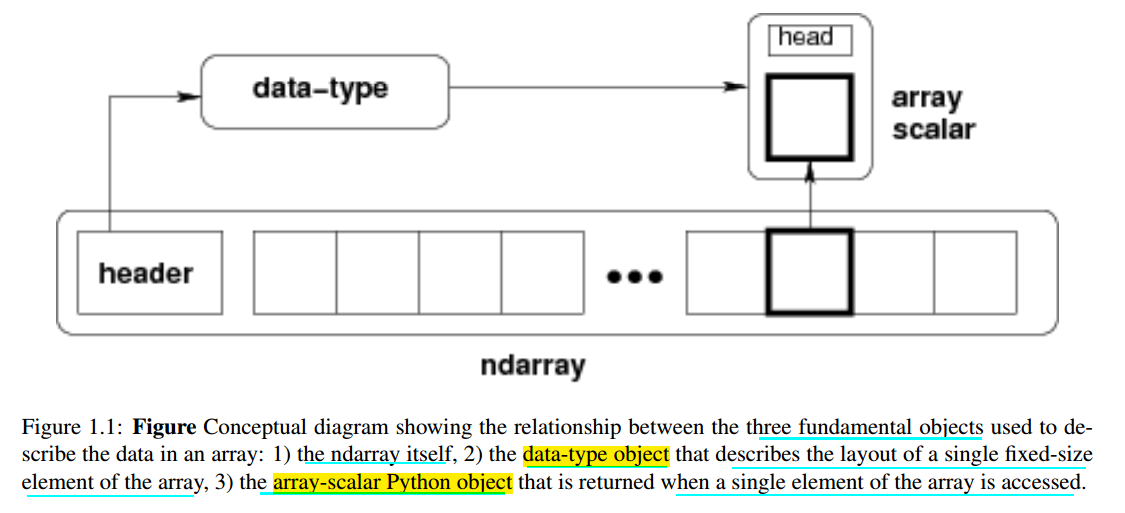
其实ndarray中的数据也可以是字符串
numpy的两个重要特点vectorization与broadcasting
Vectorization describes the absence of any explicit looping, indexing, etc., inthe code - these things are taking place, of course, just “behind the scenes”in optimized, pre-compiled C code. Vectorized code has many advantages, amongwhich are:
vectorized code is more conciseand easier to read
fewer lines of code generallymeans fewer bugs
the code more closely resemblesstandard mathematical notation (making it easier, typically, to correctly code mathematicalconstructs)
vectorization results in more“Pythonic” code. Without vectorization, our code would be littered withinefficient and difficult to read for loops.
Broadcasting is the term used to describe the implicitelement-by-element behavior of operations; generally speaking,in NumPy alloperations, not just arithmetic operations, but logical, bit-wise, functional,etc., behave in this implicit element-by-element fashion, i.e., they broadcast.
Numpy中broadcasting
Broadcasting can be understood by four rules:
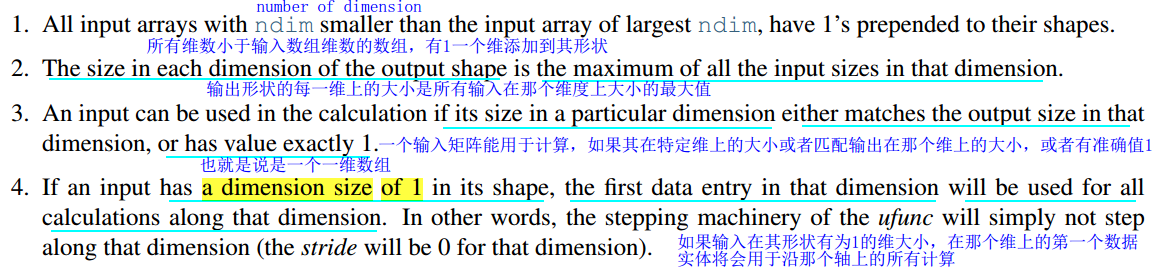
让所有输入数组都向其中shape最长的数组看齐,shape中不足的部分都通过在前面加1补齐
输出数组的shape是输入数组shape的各个轴上的最大值
如果输入数组的某个轴 (和输出数组的对应轴的长度相同)或者(其长度为1)时,这个输入数组能够用来计算,否则出错
当输入数组的某个轴的长度为1时,沿着此轴运算时都用此轴上的第一个数据实体
A set of arrays is called “broadcastable” to the same shape if the above rules produce a validresult, i.e., one of the following is true:

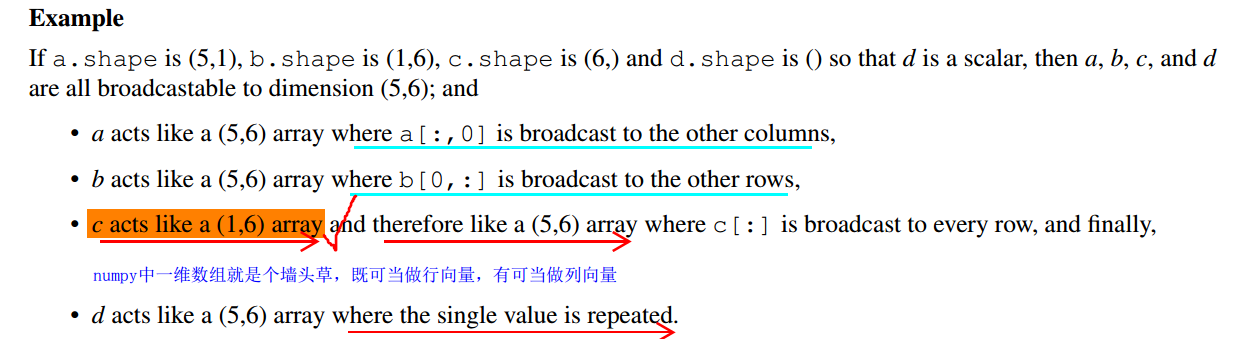
索引多维数组的某一列时返回的是一个行向量
>>> X = np.array([[1, 2, 3, 4], [5, 6, 7, 8], [9, 10, 11, 12]])
>>> X[:, 1]
array([2, 6, 10]) % 这里是一个行
>>> X[:, 1].shape % X[:, 1]的用法完全等同于一个行,而不是一个列
(3, )
所以,一种正确的索引方式是:
>>>X[:, 1][:, np.newaxis]
array([[2],
[6],
[10]])
也就是说shape为(m, )的ndarray数组,其真实的shape为(1, m),即行向量
numpy和scipy的关系
numpy 准确地说提供了一个在python中做科学计算的基础库,侠义地讲它重在数值计算,甚至可以说是用于多维数组处理的库;而 scipy 则是基于numpy,提供了一个在python中做科学计算的工具集,也就是说它是更上一个层次的库