(1)Domain Adaptive Dialog Generation via Meta Learning
(2)Incorporating the Structure of the Belief State in End-to-End Task-Oriented Dialogue Systems
(3)Flexibly-Structured Model for Task-Oriented Dialogues
1
Globally和Locally有什么区别?
M指的是Model,k指的是某一个具体的domain(
D
t
r
a
i
n
S
k
D_{train}^{S_k}
DtrainSk,
D
t
r
a
i
n
T
D_{train}^{T}
DtrainT),模型主要是Domain transfer,基本模型是MAML,
restaurant, weather, and bus information->movie information search
We propose to apply meta-learning algorithm on top of the sequicity model to achieve dialog domain adaptation The intuition behind the MAML algorithm is that some internal representations are more transferable than others. This suggests that some internal features can be applied to multiple dialog domains rather than a single domain.
The idea behind this oper-ation is that the loss calculated from the updated model is obviously more sensitive to the changes in original domains, so that we learn more about the common internal representations of all source domains rather than the distinctive features of each domain.
Then in the adaptation step, since the basic internal representation has already been captured, the model is sensitive to the unique features of the new domain. As a result, one or a few gradient steps and minimum amount of data are required to optimize the model to the new domain.
单纯sum_loss会不会无法提取多领域对话的共享特征,而且在 M k ′ M_{k}^{'} Mk′更新时只有one step。
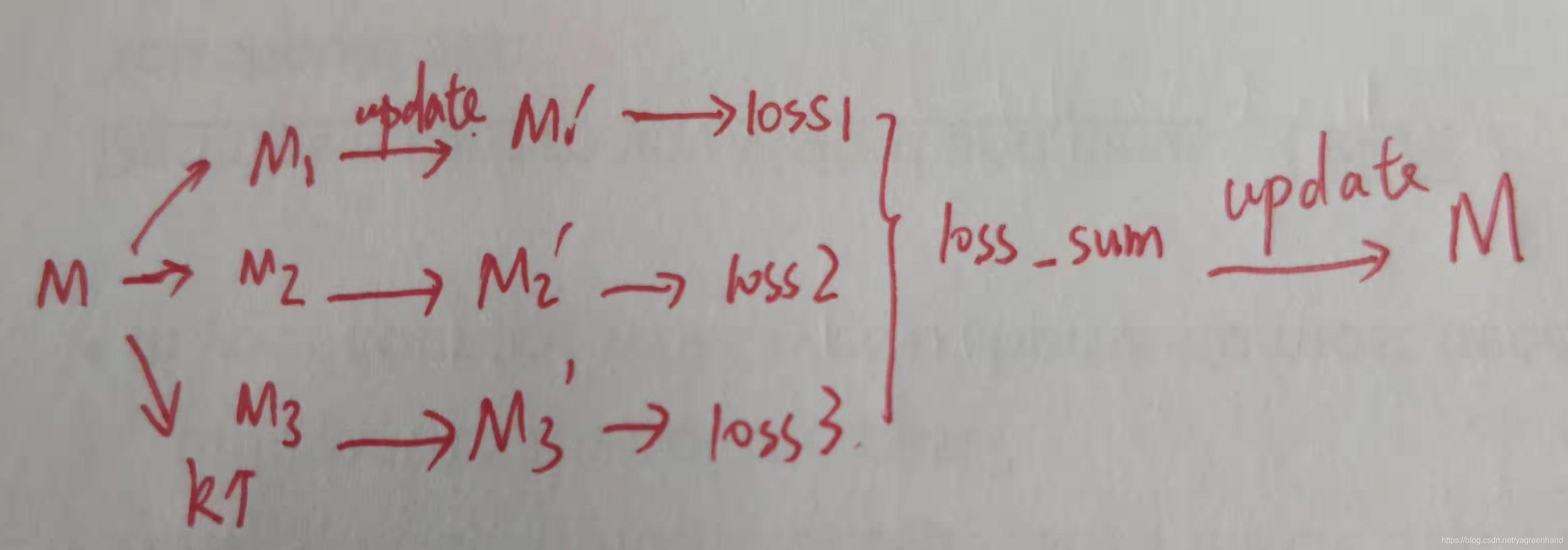
(2)
Informable Slot Value Decoder,对于每个slot是单独的GRU,那怎么知道当前句子有几个slot呢。使得每个slot的产生互不干扰,感觉很有道理。
Requestable Slot Binary Classifier,通过GRU然后二分类
Response Slot Binary Classifier,就是说
loss是几个loss的加和,小loss和大loss 一起训练。
It explicitly uses the structure of the belief state for guiding the design of an architecture that provides better inductive bias。
感觉以前不是用过了吗?
(3)
与(2)只是说法不同,模型大同小异。inductive bias作为一个词没有解释清楚,我感觉是训练集和测试集有偏差,所以模型应该要学到本质的东西。
模型说flexible(free-form)和structured的中和.
For instance, assume the requestable slot in the belief state is “address” and the KB query returned one candidate record. The response slot binary classifier may predict name slot, ad- dress slot and area slot, which are expected to appear in an agent response as “name slot is located in address slot in the area slot part of town
requestable slot提炼出“address”,KB返回“1”,则response slot里面应该有name slot, ad- dress slot and area slot,因为生成的句子需要是**“name slot is located
in address slot in the area slot part of town**。
这和copynet没有什么区别?,
模型构建的时候考虑最全面最完美的对话,有inform和request。
对于response classfier我认为是加强版的copynet,增加输入,有一点小的创新。