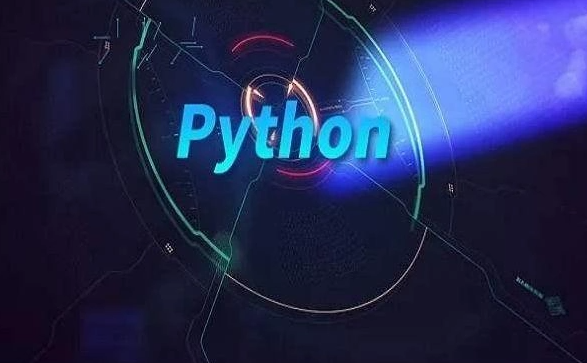
相信大家在做一些算法经常会被庞大的数据量所造成的超多计算量需要的时间而折磨的痛苦不已,接下来我们围绕四个方法来帮助大家加快一下Python的计算时间,减少大家在算法上的等待时间
1.介绍:
在PyTorch模块中,我将展示如何使用torch
和检查、初始化GPU设备pycuda
,以及如何使算法更快。
PyTorch是建立在torch的机器学习库。它得到了Facebook AI研究小组的支持。在最近开发之后,由于它的简单性,动态图形以及它本质上是Python,它在被开发出来之后变得非常流行。它的速度仍然没有落后,在很多情况下可以说是表现的非常好的。
pycuda允许你从python访问Nvidia的CUDA并行计算API。
2.如何检查cuda的可用性?
要检查是否有cuda
可用的设备Torch
,可以简单地运行一下下面的代码:
<pre spellcheck="false" class="md-fences md-end-block ty-contain-cm modeLoaded" lang="Python" cid="n38" mdtype="fences" style="box-sizing: border-box; overflow: visible; font-family: var(--monospace); font-size: 0.9em; display: block; break-inside: avoid; text-align: left; white-space: normal; background-image: inherit; background-position: inherit; background-size: inherit; background-repeat: inherit; background-attachment: inherit; background-origin: inherit; background-clip: inherit; background-color: rgb(248, 248, 248); position: relative !important; border: 1px solid rgb(231, 234, 237); border-radius: 3px; padding: 8px 4px 6px; margin-bottom: 15px; margin-top: 15px; width: inherit; color: rgb(51, 51, 51); font-style: normal; font-variant-ligatures: normal; font-variant-caps: normal; font-weight: 400; letter-spacing: normal; orphans: 2; text-indent: 0px; text-transform: none; widows: 2; word-spacing: 0px; -webkit-text-stroke-width: 0px; text-decoration-style: initial; text-decoration-color: initial;">import torch
torch.cuda.is_available()
True</pre>
3.如何获取有关你的cuda设备的更多信息?
要获取设备的基本信息,可以使用torch.cuda
。但是,要获取有关设备的更多信息,可以使用pycuda
,这是一个围绕CUDA
库开发的python包装器。你可以使用:
<pre spellcheck="false" class="md-fences md-end-block ty-contain-cm modeLoaded" lang="Python" cid="n41" mdtype="fences" style="box-sizing: border-box; overflow: visible; font-family: var(--monospace); font-size: 0.9em; display: block; break-inside: avoid; text-align: left; white-space: normal; background-image: inherit; background-position: inherit; background-size: inherit; background-repeat: inherit; background-attachment: inherit; background-origin: inherit; background-clip: inherit; background-color: rgb(248, 248, 248); position: relative !important; border: 1px solid rgb(231, 234, 237); border-radius: 3px; padding: 8px 4px 6px; margin-bottom: 15px; margin-top: 15px; width: inherit; color: rgb(51, 51, 51); font-style: normal; font-variant-ligatures: normal; font-variant-caps: normal; font-weight: 400; letter-spacing: normal; orphans: 2; text-indent: 0px; text-transform: none; widows: 2; word-spacing: 0px; -webkit-text-stroke-width: 0px; text-decoration-style: initial; text-decoration-color: initial;">import torch
import pycuda.driver as cuda
cuda.init()
获取默认设备的Id
torch.cuda.current_device()
0
cuda.Device(0).name() # '0'是你的GPU的id
Tesla K80</pre>
或者你可以这么用:
<pre spellcheck="false" class="md-fences md-end-block ty-contain-cm modeLoaded" lang="" cid="n43" mdtype="fences" style="box-sizing: border-box; overflow: visible; font-family: var(--monospace); font-size: 0.9em; display: block; break-inside: avoid; text-align: left; white-space: normal; background-image: inherit; background-position: inherit; background-size: inherit; background-repeat: inherit; background-attachment: inherit; background-origin: inherit; background-clip: inherit; background-color: rgb(248, 248, 248); position: relative !important; border: 1px solid rgb(231, 234, 237); border-radius: 3px; padding: 8px 4px 6px; margin-bottom: 15px; margin-top: 15px; width: inherit; color: rgb(51, 51, 51); font-style: normal; font-variant-ligatures: normal; font-variant-caps: normal; font-weight: 400; letter-spacing: normal; orphans: 2; text-indent: 0px; text-transform: none; widows: 2; word-spacing: 0px; -webkit-text-stroke-width: 0px; text-decoration-style: initial; text-decoration-color: initial;">torch.cuda.get_device_name(0)#获取ID为'0'的名称设备
#'Tesla K80'</pre>
我编写了一个简单的类来获取有关cuda
兼容GPU的信息:
<pre spellcheck="false" class="md-fences md-end-block ty-contain-cm modeLoaded" lang="Python" cid="n45" mdtype="fences" style="box-sizing: border-box; overflow: visible; font-family: var(--monospace); font-size: 0.9em; display: block; break-inside: avoid; text-align: left; white-space: normal; background-image: inherit; background-position: inherit; background-size: inherit; background-repeat: inherit; background-attachment: inherit; background-origin: inherit; background-clip: inherit; background-color: rgb(248, 248, 248); position: relative !important; border: 1px solid rgb(231, 234, 237); border-radius: 3px; padding: 8px 4px 6px; margin-bottom: 15px; margin-top: 15px; width: inherit; color: rgb(51, 51, 51); font-style: normal; font-variant-ligatures: normal; font-variant-caps: normal; font-weight: 400; letter-spacing: normal; orphans: 2; text-indent: 0px; text-transform: none; widows: 2; word-spacing: 0px; -webkit-text-stroke-width: 0px; text-decoration-style: initial; text-decoration-color: initial;">#一个简单的类来了解你的cuda设备
import pycuda.driver as cuda
import pycuda.autoinit #必须使用它的功能
cuda.init() # 需要使用它的功能
class aboutCudaDevices():
def init(self):
pass
def num_devices(self):
"""返回连接的设备数量."""
return cuda.Device.count()
def devices(self):
"""获取所有连接设备的信息."""
num = cuda.Device.count()
print("%d device(s) found:"%num)
for i in range(num):
print(cuda.Device(i).name(), "(Id: %d)"%i)
def mem_info(self):
"""获得所有设备的可用内存和总内存."""
available, total = cuda.mem_get_info()
print("Available: %.2f GB\nTotal: %.2f GB"%(available/1e9, total/1e9))
def attributes(self, device_id=0):
"""获取设备Id的属性 = device_id"""
return cuda.Device(device_id).get_attributes()
def repr(self):
"""类表示为连接到它们的设备的数量."""
num = cuda.Device.count()
string = ""
string += ("%d device(s) found:\n"%num)
for i in range(num):
string += ( " %d) %s (Id: %d)\n"%((i+1),cuda.Device(i).name(),i))
string += (" Memory: %.2f GB\n"%(cuda.Device(i).total_memory()/1e9))
return string
你可以通过输入它的名字来打印输出(repr):
aboutCudaDevices()
1 设备(年代):
1) Tesla K80 (Id: 0)
Memory: 12.00 GB</pre>
要获取当前的内存使用情况,你可以使用pyTorch
的函数:
<pre spellcheck="false" class="md-fences md-end-block ty-contain-cm modeLoaded" lang="Python" cid="n47" mdtype="fences" style="box-sizing: border-box; overflow: visible; font-family: var(--monospace); font-size: 0.9em; display: block; break-inside: avoid; text-align: left; white-space: normal; background-image: inherit; background-position: inherit; background-size: inherit; background-repeat: inherit; background-attachment: inherit; background-origin: inherit; background-clip: inherit; background-color: rgb(248, 248, 248); position: relative !important; border: 1px solid rgb(231, 234, 237); border-radius: 3px; padding: 8px 4px 6px; margin-bottom: 15px; margin-top: 15px; width: inherit; color: rgb(51, 51, 51); font-style: normal; font-variant-ligatures: normal; font-variant-caps: normal; font-weight: 400; letter-spacing: normal; orphans: 2; text-indent: 0px; text-transform: none; widows: 2; word-spacing: 0px; -webkit-text-stroke-width: 0px; text-decoration-style: initial; text-decoration-color: initial;">import torch
返回当前的GPU内存使用
一个给定设备的容量(以字节为单位)
torch.cuda.memory_allocated()
函数管理的当前的GPU内存
以字节为给定设备缓存的分配器
torch.cuda.memory_cached()</pre>
在运行应用程序后,可以使用一个简单的命令来清除缓存:
<pre spellcheck="false" class="md-fences md-end-block ty-contain-cm modeLoaded" lang="Python" cid="n49" mdtype="fences" style="box-sizing: border-box; overflow: visible; font-family: var(--monospace); font-size: 0.9em; display: block; break-inside: avoid; text-align: left; white-space: normal; background-image: inherit; background-position: inherit; background-size: inherit; background-repeat: inherit; background-attachment: inherit; background-origin: inherit; background-clip: inherit; background-color: rgb(248, 248, 248); position: relative !important; border: 1px solid rgb(231, 234, 237); border-radius: 3px; padding: 8px 4px 6px; margin-bottom: 15px; margin-top: 15px; width: inherit; color: rgb(51, 51, 51); font-style: normal; font-variant-ligatures: normal; font-variant-caps: normal; font-weight: 400; letter-spacing: normal; orphans: 2; text-indent: 0px; text-transform: none; widows: 2; word-spacing: 0px; -webkit-text-stroke-width: 0px; text-decoration-style: initial; text-decoration-color: initial;"># 释放缓存分配器当前持有的所有为占用的缓存内存
以便这些内存可以在其他GPU应用程序中可以使用
并且可以在NVIDIA-SMI中可以进行查看
torch.cuda.empty_cache()</pre>
但是,使用这个命令不会通过张量释放占用的GPU内存,因此它无法增加可用于PyTorch的GPU内存量。
这些内存方法仅适用于GPU。所以这才是真正需要它的地方。
4.如何存储张量并在GPU上运行模型?
使用
.cuda
函数。
如果你想要在CPU上存储一些内容,可以简单地编写代码:
<pre spellcheck="false" class="md-fences md-end-block ty-contain-cm modeLoaded" lang="Python" cid="n56" mdtype="fences" style="box-sizing: border-box; overflow: visible; font-family: var(--monospace); font-size: 0.9em; display: block; break-inside: avoid; text-align: left; white-space: normal; background-image: inherit; background-position: inherit; background-size: inherit; background-repeat: inherit; background-attachment: inherit; background-origin: inherit; background-clip: inherit; background-color: rgb(248, 248, 248); position: relative !important; border: 1px solid rgb(231, 234, 237); border-radius: 3px; padding: 8px 4px 6px; margin-bottom: 15px; margin-top: 15px; width: inherit; color: rgb(51, 51, 51); font-style: normal; font-variant-ligatures: normal; font-variant-caps: normal; font-weight: 400; letter-spacing: normal; orphans: 2; text-indent: 0px; text-transform: none; widows: 2; word-spacing: 0px; -webkit-text-stroke-width: 0px; text-decoration-style: initial; text-decoration-color: initial;">a = torch.DoubleTensor([1., 2.])</pre>
这个向量是存储在CPU上的,你对它执行的任何操作都是在CPU上执行完成的。而要将其转移到GPU上,你只需要执行以下操作.cuda
:
<pre spellcheck="false" class="md-fences md-end-block ty-contain-cm modeLoaded" lang="Python" cid="n58" mdtype="fences" style="box-sizing: border-box; overflow: visible; font-family: var(--monospace); font-size: 0.9em; display: block; break-inside: avoid; text-align: left; white-space: normal; background-image: inherit; background-position: inherit; background-size: inherit; background-repeat: inherit; background-attachment: inherit; background-origin: inherit; background-clip: inherit; background-color: rgb(248, 248, 248); position: relative !important; border: 1px solid rgb(231, 234, 237); border-radius: 3px; padding: 8px 4px 6px; margin-bottom: 15px; margin-top: 15px; width: inherit; color: rgb(51, 51, 51); font-style: normal; font-variant-ligatures: normal; font-variant-caps: normal; font-weight: 400; letter-spacing: normal; orphans: 2; text-indent: 0px; text-transform: none; widows: 2; word-spacing: 0px; -webkit-text-stroke-width: 0px; text-decoration-style: initial; text-decoration-color: initial;">a = torch.FloatTensor([1., 2.]).cuda()</pre>
或者,
<pre spellcheck="false" class="md-fences md-end-block ty-contain-cm modeLoaded" lang="Python" cid="n60" mdtype="fences" style="box-sizing: border-box; overflow: visible; font-family: var(--monospace); font-size: 0.9em; display: block; break-inside: avoid; text-align: left; white-space: normal; background-image: inherit; background-position: inherit; background-size: inherit; background-repeat: inherit; background-attachment: inherit; background-origin: inherit; background-clip: inherit; background-color: rgb(248, 248, 248); position: relative !important; border: 1px solid rgb(231, 234, 237); border-radius: 3px; padding: 8px 4px 6px; margin-bottom: 15px; margin-top: 15px; width: inherit; color: rgb(51, 51, 51); font-style: normal; font-variant-ligatures: normal; font-variant-caps: normal; font-weight: 400; letter-spacing: normal; orphans: 2; text-indent: 0px; text-transform: none; widows: 2; word-spacing: 0px; -webkit-text-stroke-width: 0px; text-decoration-style: initial; text-decoration-color: initial;">a = torch.cuda.FloatTensor([1., 2.])</pre>
这将为它选择默认设备,该默认设备可通过以下命令查看:
<pre spellcheck="false" class="md-fences md-end-block ty-contain-cm modeLoaded" lang="Python" cid="n62" mdtype="fences" style="box-sizing: border-box; overflow: visible; font-family: var(--monospace); font-size: 0.9em; display: block; break-inside: avoid; text-align: left; white-space: normal; background-image: inherit; background-position: inherit; background-size: inherit; background-repeat: inherit; background-attachment: inherit; background-origin: inherit; background-clip: inherit; background-color: rgb(248, 248, 248); position: relative !important; border: 1px solid rgb(231, 234, 237); border-radius: 3px; padding: 8px 4px 6px; margin-bottom: 15px; margin-top: 15px; width: inherit; color: rgb(51, 51, 51); font-style: normal; font-variant-ligatures: normal; font-variant-caps: normal; font-weight: 400; letter-spacing: normal; orphans: 2; text-indent: 0px; text-transform: none; widows: 2; word-spacing: 0px; -webkit-text-stroke-width: 0px; text-decoration-style: initial; text-decoration-color: initial;">torch.cuda.current_device()
#0</pre>
或者,你也可以执行以下操作:
<pre spellcheck="false" class="md-fences md-end-block ty-contain-cm modeLoaded" lang="Python" cid="n64" mdtype="fences" style="box-sizing: border-box; overflow: visible; font-family: var(--monospace); font-size: 0.9em; display: block; break-inside: avoid; text-align: left; white-space: normal; background-image: inherit; background-position: inherit; background-size: inherit; background-repeat: inherit; background-attachment: inherit; background-origin: inherit; background-clip: inherit; background-color: rgb(248, 248, 248); position: relative !important; border: 1px solid rgb(231, 234, 237); border-radius: 3px; padding: 8px 4px 6px; margin-bottom: 15px; margin-top: 15px; width: inherit; color: rgb(51, 51, 51); font-style: normal; font-variant-ligatures: normal; font-variant-caps: normal; font-weight: 400; letter-spacing: normal; orphans: 2; text-indent: 0px; text-transform: none; widows: 2; word-spacing: 0px; -webkit-text-stroke-width: 0px; text-decoration-style: initial; text-decoration-color: initial;">a.get_device()
#0</pre>
你也可以将一个模型发送到GPU设备。例如,考虑一个简单的模块nn.Sequential
:
<pre spellcheck="false" class="md-fences md-end-block ty-contain-cm modeLoaded" lang="Python" cid="n66" mdtype="fences" style="box-sizing: border-box; overflow: visible; font-family: var(--monospace); font-size: 0.9em; display: block; break-inside: avoid; text-align: left; white-space: normal; background-image: inherit; background-position: inherit; background-size: inherit; background-repeat: inherit; background-attachment: inherit; background-origin: inherit; background-clip: inherit; background-color: rgb(248, 248, 248); position: relative !important; border: 1px solid rgb(231, 234, 237); border-radius: 3px; padding: 8px 4px 6px; margin-bottom: 15px; margin-top: 15px; width: inherit; color: rgb(51, 51, 51); font-style: normal; font-variant-ligatures: normal; font-variant-caps: normal; font-weight: 400; letter-spacing: normal; orphans: 2; text-indent: 0px; text-transform: none; widows: 2; word-spacing: 0px; -webkit-text-stroke-width: 0px; text-decoration-style: initial; text-decoration-color: initial;">sq = nn.Sequential(
nn.Linear(20,20),
nn.ReLU(),
nn.Linear(
20,4 ),nn.Softmax()
)</pre>
要将其发送到GPU设备,只需执行以下操作:
<pre spellcheck="false" class="md-fences md-end-block ty-contain-cm modeLoaded" lang="Python" cid="n68" mdtype="fences" style="box-sizing: border-box; overflow: visible; font-family: var(--monospace); font-size: 0.9em; display: block; break-inside: avoid; text-align: left; white-space: normal; background-image: inherit; background-position: inherit; background-size: inherit; background-repeat: inherit; background-attachment: inherit; background-origin: inherit; background-clip: inherit; background-color: rgb(248, 248, 248); position: relative !important; border: 1px solid rgb(231, 234, 237); border-radius: 3px; padding: 8px 4px 6px; margin-bottom: 15px; margin-top: 15px; width: inherit; color: rgb(51, 51, 51); font-style: normal; font-variant-ligatures: normal; font-variant-caps: normal; font-weight: 400; letter-spacing: normal; orphans: 2; text-indent: 0px; text-transform: none; widows: 2; word-spacing: 0px; -webkit-text-stroke-width: 0px; text-decoration-style: initial; text-decoration-color: initial;">model = sq.cuda()</pre>
你可以检查它是否在GPU设备上,为此,你必须检查它的参数是否在GPU设备上,例如:
<pre spellcheck="false" class="md-fences md-end-block ty-contain-cm modeLoaded" lang="Python" cid="n70" mdtype="fences" style="box-sizing: border-box; overflow: visible; font-family: var(--monospace); font-size: 0.9em; display: block; break-inside: avoid; text-align: left; white-space: normal; background-image: inherit; background-position: inherit; background-size: inherit; background-repeat: inherit; background-attachment: inherit; background-origin: inherit; background-clip: inherit; background-color: rgb(248, 248, 248); position: relative !important; border: 1px solid rgb(231, 234, 237); border-radius: 3px; padding: 8px 4px 6px; margin-bottom: 15px; margin-top: 15px; width: inherit; color: rgb(51, 51, 51); font-style: normal; font-variant-ligatures: normal; font-variant-caps: normal; font-weight: 400; letter-spacing: normal; orphans: 2; text-indent: 0px; text-transform: none; widows: 2; word-spacing: 0px; -webkit-text-stroke-width: 0px; text-decoration-style: initial; text-decoration-color: initial;">#可以在这里进行讨论: discuss.pytorch.org/t/how-to-check-if-model-is-on-cuda
next(model.parameters()).is_cuda
True</pre>
5.如果有多个GPU,如何选择和使用GPU?
你可以为当前应用/存储选择一个GPU,该GPU可以与你上一个应用/存储选择的GPU不同。
正如在第(2)部分中已经看到的那样,我们可以获得所有与cuda
兼容的设备及其Id
使用pycuda
的情况,在此我们就不再赘述了。
考虑到你有3个cuda
兼容的设备,你可以初始化和分配tensors
到一个特定的设备,就像这样。
<pre spellcheck="false" class="md-fences md-end-block ty-contain-cm modeLoaded" lang="Python" cid="n75" mdtype="fences" style="box-sizing: border-box; overflow: visible; font-family: var(--monospace); font-size: 0.9em; display: block; break-inside: avoid; text-align: left; white-space: normal; background-image: inherit; background-position: inherit; background-size: inherit; background-repeat: inherit; background-attachment: inherit; background-origin: inherit; background-clip: inherit; background-color: rgb(248, 248, 248); position: relative !important; border: 1px solid rgb(231, 234, 237); border-radius: 3px; padding: 8px 4px 6px; margin-bottom: 15px; margin-top: 15px; width: inherit; color: rgb(51, 51, 51); font-style: normal; font-variant-ligatures: normal; font-variant-caps: normal; font-weight: 400; letter-spacing: normal; orphans: 2; text-indent: 0px; text-transform: none; widows: 2; word-spacing: 0px; -webkit-text-stroke-width: 0px; text-decoration-style: initial; text-decoration-color: initial;">cuda0 = torch.device('cuda:0')
cuda1 = torch.device('cuda:1')
cuda2 = torch.device('cuda:2')
如果你只使用 'cuda' , 张量/型号将会被发送到默认(当前)设备。(默认值= 0)
x = torch.Tensor([1., 2.], device=cuda1)
或者
x = torch.Tensor([1., 2.]).to(cuda1)
或者
x = torch.Tensor([1., 2.]).cuda(cuda1)
笔记:
如果你想改变默认设备,那么请使用:
torch.cuda.set_device(2) # 设备Id为'2'的地方
如果你只想使用3个GPU中的2个,那么你必须设置环境变量CUDA_VISIBLE_DEVICES 等于"0,2",如果你只想使用第一个和第三个GPU的话
现在如果你想要检查有多少个GPU时,它将显示两个(0,1)
import os
os.environ["CUDA_VISIBLE_DEVICES"] = "0,2"</pre>
当你对这些Tensor
s 进行任何操作时,无论选择什么设备,都可以执行该操作,结果会和Tensor
保存在同一个设备上。
<pre spellcheck="false" class="md-fences md-end-block ty-contain-cm modeLoaded" lang="Python" cid="n77" mdtype="fences" style="box-sizing: border-box; overflow: visible; font-family: var(--monospace); font-size: 0.9em; display: block; break-inside: avoid; text-align: left; white-space: normal; background-image: inherit; background-position: inherit; background-size: inherit; background-repeat: inherit; background-attachment: inherit; background-origin: inherit; background-clip: inherit; background-color: rgb(248, 248, 248); position: relative !important; border: 1px solid rgb(231, 234, 237); border-radius: 3px; padding: 8px 4px 6px; margin-bottom: 15px; margin-top: 15px; width: inherit; color: rgb(51, 51, 51); font-style: normal; font-variant-ligatures: normal; font-variant-caps: normal; font-weight: 400; letter-spacing: normal; orphans: 2; text-indent: 0px; text-transform: none; widows: 2; word-spacing: 0px; -webkit-text-stroke-width: 0px; text-decoration-style: initial; text-decoration-color: initial;">x = torch.Tensor([1., 2.]).to(cuda2)
y = torch.Tensor([3., 4.]).to(cuda2)
这个Tensor(张量)只会保存在'cuda2' 中
z = x + y</pre>
如果你有多个GPU,则可以在其中划分应用程序的工作,但是他们之间的通信会带来开销。但是,如果你不需要过多的进行传递信息,那你可以尝试一下。
实际上还有一个问题。在PyTorch
中的所有GPU操作中,默认情况下都是异步的。尽管在CPU和GPU或两个GPU之间复制数据时确实进行了必要的同步,但是,如果你在命令torch.cuda.Stream()
的帮助下创建自己的数据流,那么你讲不得不处理指令的同步
举一个PyTorch
文档中的例子,这是不正确的:
<pre spellcheck="false" class="md-fences md-end-block ty-contain-cm modeLoaded" lang="" cid="n81" mdtype="fences" style="box-sizing: border-box; overflow: visible; font-family: var(--monospace); font-size: 0.9em; display: block; break-inside: avoid; text-align: left; white-space: normal; background-image: inherit; background-position: inherit; background-size: inherit; background-repeat: inherit; background-attachment: inherit; background-origin: inherit; background-clip: inherit; background-color: rgb(248, 248, 248); position: relative !important; border: 1px solid rgb(231, 234, 237); border-radius: 3px; padding: 8px 4px 6px; margin-bottom: 15px; margin-top: 15px; width: inherit; color: rgb(51, 51, 51); font-style: normal; font-variant-ligatures: normal; font-variant-caps: normal; font-weight: 400; letter-spacing: normal; orphans: 2; text-indent: 0px; text-transform: none; widows: 2; word-spacing: 0px; -webkit-text-stroke-width: 0px; text-decoration-style: initial; text-decoration-color: initial;">cuda = torch.device('cuda')
s = torch.cuda.Stream() # 创建一个新的数据流.
A = torch.empty((100, 100), device=cuda).normal_(0.0, 1.0)
with torch.cuda.stream(s):
因为 sum() 可能会在normal_()结束之前开始执行!
B = torch.sum(A)</pre>
如果你想充分利用多个GPU,那么你可以:
将所有GPU用于不同的任务/应用程序
将每个GPU用于集合或堆栈中的一个模型,每个GPU都有数据副本(如果可能的话),因为大多数处理是在拟合模型期间完成的,
在每个GPU中使用带有切片输入的每个GPU和模型副本。每个GPU都会分别计算结果,并将其结果发送到目标GPU,然后再进行进一步的计算等。
6.数据并行性
在数据并行性中,我们将数据(从数据生成器中获得的一个批次的数据)分割为较小的小型批次的数据,然后将其发送到多个GPU进行并行计算。
在PyTorch
中,数据并行中是使用torch.nn.DataParallel
实现的
我们将看到一个简单的例子来了解实际情况。为此,我们将必须使用nn.parallel
的某些功能:
Replicate(复制):
Module
在多个设备上复制。Scatter(分散):
input
将第一个维度分配到这些设备中。Gather(聚集):
input
收集并连接这些设备的第一个维度。parallel_apply:将我们从我们从Scatter中得到的一套分布式的
input
,输入到相应的分布式Module
中,我们是通过复制得到的这些模块。
<pre spellcheck="false" class="md-fences md-end-block ty-contain-cm modeLoaded" lang="Python" cid="n103" mdtype="fences" style="box-sizing: border-box; overflow: visible; font-family: var(--monospace); font-size: 0.9em; display: block; break-inside: avoid; text-align: left; white-space: normal; background-image: inherit; background-position: inherit; background-size: inherit; background-repeat: inherit; background-attachment: inherit; background-origin: inherit; background-clip: inherit; background-color: rgb(248, 248, 248); position: relative !important; border: 1px solid rgb(231, 234, 237); border-radius: 3px; padding: 8px 4px 6px; margin-bottom: 15px; margin-top: 15px; width: inherit; color: rgb(51, 51, 51); font-style: normal; font-variant-ligatures: normal; font-variant-caps: normal; font-weight: 400; letter-spacing: normal; orphans: 2; text-indent: 0px; text-transform: none; widows: 2; word-spacing: 0px; -webkit-text-stroke-width: 0px; text-decoration-style: initial; text-decoration-color: initial;">#将模块复制到设备id中的设备
replicas = nn.parallel.replicate(module, device_ids)
将输入分配到设备id中的设备
inputs = nn.parallel.scatter(input, device_ids)
将模型应用于相应的输入
outputs = nn.parallel.parallel_apply(replicas, inputs)
收集所有设备的结果到output_device
result = nn.parallel.gather(outputs, output_device)</pre>
或者,只需要简单地:
<pre spellcheck="false" class="md-fences md-end-block ty-contain-cm modeLoaded" lang="" cid="n105" mdtype="fences" style="box-sizing: border-box; overflow: visible; font-family: var(--monospace); font-size: 0.9em; display: block; break-inside: avoid; text-align: left; white-space: normal; background-image: inherit; background-position: inherit; background-size: inherit; background-repeat: inherit; background-attachment: inherit; background-origin: inherit; background-clip: inherit; background-color: rgb(248, 248, 248); position: relative !important; border: 1px solid rgb(231, 234, 237); border-radius: 3px; padding: 8px 4px 6px; margin-bottom: 15px; margin-top: 15px; width: inherit; color: rgb(51, 51, 51); font-style: normal; font-variant-ligatures: normal; font-variant-caps: normal; font-weight: 400; letter-spacing: normal; orphans: 2; text-indent: 0px; text-transform: none; widows: 2; word-spacing: 0px; -webkit-text-stroke-width: 0px; text-decoration-style: initial; text-decoration-color: initial;">model = nn.DataParallel(model, device_ids=device_ids)
result = model(input)</pre>
7.数据并行比较
训练集数据+Val w/数据加载器+SSD中对真实数据的数据扩充
方式 | 1*V100/CUDA 9/CuDNN 7 | 4*V100/CUDA 9/CuDNN 7 |
---|---|---|
Pytorch | 27分钟 | 10分钟 |
Keras(TF) | 38分钟 | 18分钟 |
Tensorflow | 33分钟 | 22分钟 |
Chainer | 29分钟 | 8分钟 |
MXNet(Gluon) | 29分钟 | 10分钟 |
训练集W/在内存中的综合数据
方式 | 1*V100/CUDA 9/CuDnn 7 | 4*V100/CUDA 9 / CuDNN 7 |
---|---|---|
Pytorch | 25分钟 | 8分钟 |
Keras(TF) | 36分钟 | 15分钟 |
Tensorflow | 25分钟 | 14分钟 |
Chainer | 27分钟 | 7分钟 |
MxNet(Gluon) | 28分钟 | 8分钟 |
现在,你可以清楚的看到,即使必须在开始和结束时与主设备进行通信,并行处理也绝对是有帮助的。并且仅在多GPU情况下,PyTorch
比所有结果提供结果的时间更快仅仅略低于Chainer
。Pytorch
只需要通过对DataParallel
的一次调用,就会使其变得简单。
8.torch.multiprocessing
torch.multiprocessing
是Python multiprocessing
模块的包装,其API与原始模块100%兼容。因此,你可以在此处使用Python的多处理模块中的Queue
',Pipe
',Array
'等。此外,为了使其更快,他们添加了一个方法,share_memory_()
该方法允许数据进入一个状态,任何进程都可以直接使用它,因此将该数据作为参数传递给不同的进程将不会复制该数据。
你可以共享Tensors
,模型的parameters
,也可以根据需要在CPU或GPU上共享它们。
<pre spellcheck="false" class="md-fences md-end-block ty-contain-cm modeLoaded" lang="" cid="n164" mdtype="fences" style="box-sizing: border-box; overflow: visible; font-family: var(--monospace); font-size: 0.9em; display: block; break-inside: avoid; text-align: left; white-space: normal; background-image: inherit; background-position: inherit; background-size: inherit; background-repeat: inherit; background-attachment: inherit; background-origin: inherit; background-clip: inherit; background-color: rgb(248, 248, 248); position: relative !important; border: 1px solid rgb(231, 234, 237); border-radius: 3px; padding: 8px 4px 6px; margin-bottom: 15px; margin-top: 15px; width: inherit; color: rgb(51, 51, 51); font-style: normal; font-variant-ligatures: normal; font-variant-caps: normal; font-weight: 400; letter-spacing: normal; orphans: 2; text-indent: 0px; text-transform: none; widows: 2; word-spacing: 0px; -webkit-text-stroke-width: 0px; text-decoration-style: initial; text-decoration-color: initial;">来自Pytorch的警告:(关于GPU上的共享)
CUDA API要求导出到其他进程的分配只要在被其他进程使用时就保持有效。你应该小心并确保你共享的CUDA Tensors在必要时不会超出范围。这对于共享模型参数应该不是问题,但是传递其他类型的数据时应格外小心。请注意,此限制不适用于共享CPU内存。</pre>
你可以在此处的“池和进程”部分中使用上面的方法,并且要获得更快的速度,可以使用share_memory_()
方法Tensor
在所有进程之间共享(例如)而不被复制。
<pre spellcheck="false" class="md-fences md-end-block ty-contain-cm modeLoaded" lang="Python" cid="n166" mdtype="fences" style="box-sizing: border-box; overflow: visible; font-family: var(--monospace); font-size: 0.9em; display: block; break-inside: avoid; text-align: left; white-space: normal; background-image: inherit; background-position: inherit; background-size: inherit; background-repeat: inherit; background-attachment: inherit; background-origin: inherit; background-clip: inherit; background-color: rgb(248, 248, 248); position: relative !important; border: 1px solid rgb(231, 234, 237); border-radius: 3px; padding: 8px 4px 6px; margin-bottom: 15px; margin-top: 15px; width: inherit; color: rgb(51, 51, 51); font-style: normal; font-variant-ligatures: normal; font-variant-caps: normal; font-weight: 400; letter-spacing: normal; orphans: 2; text-indent: 0px; text-transform: none; widows: 2; word-spacing: 0px; -webkit-text-stroke-width: 0px; text-decoration-style: initial; text-decoration-color: initial;">#
import torch.multiprocessing as mp
def train(model):
for data, labels in data_loader:
optimizer.zero_grad()
loss_fn(model(data), labels).backward()
optimizer.step() # 这一步将更新共享参数
model = nn.Sequential(nn.Linear(n_in, n_h1),
nn.ReLU(),
nn.Linear(n_h1, n_out))
model.share_memory() # 需要 'fork' 方法工作
processes = []
for i in range(4): # No. 的过程
p = mp.Process(target=train, args=(model,))
p.start()
processes.append(p)
for p in processes: p.join()</pre>
下一期我们继续看加快Python计算的另一种方法——Numba~