Multiple h
Real Learning like PLA, what about when getting all green(right)?
Bad Sample:
Eout=12 , but getting all heads( Ein=0 )!
Bad Data for one
h
Eout(h) and
Ein(h)
far away: e.g.,
Eout(h)
big( far from
f
), but Ein(h) small(correct on most examples).
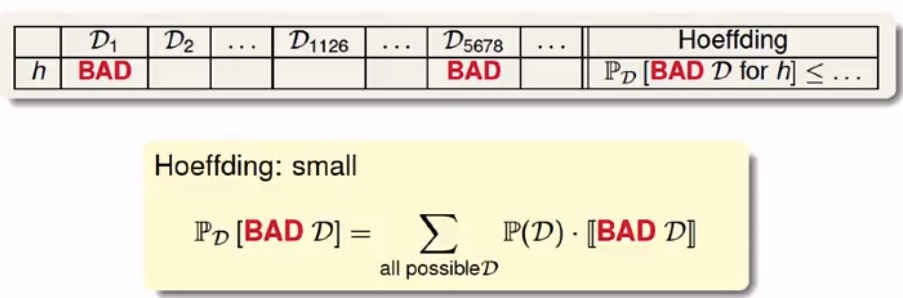
Bad Data for many h
Bad data for many
⟺
no ‘freedom of choice’ by
⟺
there exists some
h
such that
Bound of bad data
- Finite-bin version of Hoeffding, valid for all M,N,ϵ
- does not depend on any
Eout(hm)
, no need to know
Eout(hm)
—–
f
and
P can stay unknown - Ein(g)=Eout(g) is PAC, regardless of
Most reasonable : pick the hm with lowest Ein(hm) as g <script type="math/tex" id="MathJax-Element-1035">g</script>.