SLAM14讲ch7原理及代码
ORB特征点提取与匹配
#include <iostream>
#include <opencv2/core/core.hpp>
#include <opencv2/features2d/features2d.hpp>
#include <opencv2/highgui/highgui.hpp>
#include <chrono>
using namespace std;
using namespace cv;
int main(int argc, char **argv) {
if (argc != 3) {
cout << "usage: feature_extraction img1 img2" << endl;
return 1;
}
//-- 读取图像
Mat img_1 = imread(argv[1], CV_LOAD_IMAGE_COLOR); //加载彩色图像
Mat img_2 = imread(argv[2], CV_LOAD_IMAGE_COLOR);
assert(img_1.data != nullptr && img_2.data != nullptr); // 检查是否成功读取图像
//-- 初始化
std::vector<KeyPoint> keypoints_1, keypoints_2;
//定义了两个容器keypoints_1和keypoints_2,用于存储检测到的关键点。
Mat descriptors_1, descriptors_2;
//定义了两个Mat类型的变量descriptors_1和descriptors_2,用于存储对应的描述子。
Ptr<FeatureDetector> detector = ORB::create();
//使用ORB特征检测器创建了一个指针对象,该检测器用于检测关键点。
Ptr<DescriptorExtractor> descriptor = ORB::create();
//使用ORB描述子提取器创建了一个指针对象,用于提取关键点的描述子。
Ptr<DescriptorMatcher> matcher = DescriptorMatcher::create("BruteForce-Hamming");
//使用"BruteForce-Hamming"算法创建了一个描述子匹配器的指针对象,该匹配器用于在描述子间进行特征匹配。
//-- 第一步:检测 Oriented FAST 角点位置
chrono::steady_clock::time_point t1 = chrono::steady_clock::now();
detector->detect(img_1, keypoints_1);
detector->detect(img_2, keypoints_2);
//-- 第二步:根据角点位置计算 BRIEF 描述子
descriptor->compute(img_1, keypoints_1, descriptors_1);
descriptor->compute(img_2, keypoints_2, descriptors_2);
chrono::steady_clock::time_point t2 = chrono::steady_clock::now();
chrono::duration<double> time_used = chrono::duration_cast<chrono::duration<double>>(t2 - t1);
cout << "extract ORB cost = " << time_used.count() << " seconds. " << endl;
Mat outimg1;
drawKeypoints(img_1, keypoints_1, outimg1, Scalar::all(-1), DrawMatchesFlags::DEFAULT);
//Scalar::all(-1):指定绘制的关键点的颜色,这里使用Scalar::all(-1)表示使用随机颜色。
//DrawMatchesFlags::DEFAULT:指定绘制关键点的方式和附加标志。
imshow("ORB features", outimg1);
//-- 第三步:对两幅图像中的BRIEF描述子进行匹配,使用 Hamming 距离
vector<DMatch> matches;
t1 = chrono::steady_clock::now();
matcher->match(descriptors_1, descriptors_2, matches);
t2 = chrono::steady_clock::now();
time_used = chrono::duration_cast<chrono::duration<double>>(t2 - t1);
cout << "match ORB cost = " << time_used.count() << " seconds. " << endl;
//-- 第四步:匹配点对筛选
// 计算最小距离和最大距离
auto min_max = minmax_element(matches.begin(), matches.end(),
[](const DMatch &m1, const DMatch &m2) { return m1.distance < m2.distance; });
//minmax_element函数来查找特征匹配中的最小距离和最大距离。
double min_dist = min_max.first->distance;
double max_dist = min_max.second->distance;
printf("-- Max dist : %f \n", max_dist);
printf("-- Min dist : %f \n", min_dist);
//当描述子之间的距离大于两倍的最小距离时,即认为匹配有误.但有时候最小距离会非常小,设置一个经验值30作为下限.
std::vector<DMatch> good_matches;
for (int i = 0; i < descriptors_1.rows; i++) {
if (matches[i].distance <= max(2 * min_dist, 30.0)) {
good_matches.push_back(matches[i]);
}
}
//-- 第五步:绘制匹配结果
Mat img_match;
Mat img_goodmatch;
drawMatches(img_1, keypoints_1, img_2, keypoints_2, matches, img_match);
drawMatches(img_1, keypoints_1, img_2, keypoints_2, good_matches, img_goodmatch);
imshow("all matches", img_match);
imshow("good matches", img_goodmatch);
waitKey(0);
return 0;
}
特征点:ORB中的特征点是一种有方向的FAST角点,使得FAST角点拥有了尺度与旋转的描述,大大提升了不同图像之间的鲁棒性。
描述子:ORB采用BRIEF描述子,是一种二进制描述子,通常采用汉明距离进行特征点之间的描述子匹配。这里用的就是暴力匹配。
基于2D-2D的特征匹配的相机运动估计
#include <iostream>
#include <opencv2/core/core.hpp>
#include <opencv2/features2d/features2d.hpp>
#include <opencv2/highgui/highgui.hpp>
#include <opencv2/calib3d/calib3d.hpp>
// #include "extra.h" // use this if in OpenCV2
using namespace std;
using namespace cv;
/****************************************************
* 本程序演示了如何使用2D-2D的特征匹配估计相机运动
* **************************************************/
void find_feature_matches(
const Mat &img_1, const Mat &img_2,
std::vector<KeyPoint> &keypoints_1,
std::vector<KeyPoint> &keypoints_2,
std::vector<DMatch> &matches); //找到两幅图像的特征点匹配
void pose_estimation_2d2d(
std::vector<KeyPoint> keypoints_1,
std::vector<KeyPoint> keypoints_2,
std::vector<DMatch> matches,
Mat &R, Mat &t);
// 根据2D-2D的特征匹配估计相机运动,本来只要传递匹配的结果matches就行了,这里之所以还要传递keypoints_1和keypoints_2,是为了提取特征点,因为特征点的坐标是相对于图像的左上角的,而我们需要的是相对于相机坐标系的坐标,需要首先完成一个转换。
// 像素坐标转相机归一化坐标
Point2d pixel2cam(const Point2d &p, const Mat &K);
int main(int argc, char **argv) {
if (argc != 3) {
cout << "usage: pose_estimation_2d2d img1 img2" << endl;
return 1;
}
//-- 读取图像
Mat img_1 = imread(argv[1], CV_LOAD_IMAGE_COLOR);
Mat img_2 = imread(argv[2], CV_LOAD_IMAGE_COLOR);
assert(img_1.data && img_2.data && "Can not load images!"); //断言语句,判断图像是否加载成功,"Can not load images!"在断言失败时输出到标准错误流
vector<KeyPoint> keypoints_1, keypoints_2;
vector<DMatch> matches;
find_feature_matches(img_1, img_2, keypoints_1, keypoints_2, matches);
cout << "一共找到了" << matches.size() << "组匹配点" << endl;
//-- 估计两张图像间运动
Mat R, t;
pose_estimation_2d2d(keypoints_1, keypoints_2, matches, R, t);
//-- 验证E=t^R*scale
Mat t_x =
(Mat_<double>(3, 3) << 0, -t.at<double>(2, 0), t.at<double>(1, 0),
t.at<double>(2, 0), 0, -t.at<double>(0, 0),
-t.at<double>(1, 0), t.at<double>(0, 0), 0);
//下划线_表示这是一个特殊的Mat_类,通常称为"特化的Mat_",它允许使用更简洁的语法来创建固定大小的矩阵。允许直接初始化矩阵的元素值,而不需要调用矩阵对象的成员函数。
cout << "t^R=" << endl << t_x * R << endl;
//-- 验证对极约束
Mat K = (Mat_<double>(3, 3) << 520.9, 0, 325.1, 0, 521.0, 249.7, 0, 0, 1);
for (DMatch m: matches) { //遍历matches中的每个DMatch对象m
Point2d pt1 = pixel2cam(keypoints_1[m.queryIdx].pt, K);
Mat y1 = (Mat_<double>(3, 1) << pt1.x, pt1.y, 1);
Point2d pt2 = pixel2cam(keypoints_2[m.trainIdx].pt, K);
Mat y2 = (Mat_<double>(3, 1) << pt2.x, pt2.y, 1);
Mat d = y2.t() * t_x * R * y1;
cout << "epipolar constraint = " << d << endl; //理论上的epipolar约束,应该为0
}
return 0;
}
void find_feature_matches(const Mat &img_1, const Mat &img_2,
std::vector<KeyPoint> &keypoints_1,
std::vector<KeyPoint> &keypoints_2,
std::vector<DMatch> &matches) {
//-- 初始化
Mat descriptors_1, descriptors_2;
// used in OpenCV3
Ptr<FeatureDetector> detector = ORB::create(); //ORB特征检测器
Ptr<DescriptorExtractor> descriptor = ORB::create(); //ORB描述子提取器
// use this if you are in OpenCV2
// Ptr<FeatureDetector> detector = FeatureDetector::create ( "ORB" );
// Ptr<DescriptorExtractor> descriptor = DescriptorExtractor::create ( "ORB" );
Ptr<DescriptorMatcher> matcher = DescriptorMatcher::create("BruteForce-Hamming"); //基于汉明距离的穷举匹配器
//-- 第一步:检测 Oriented FAST 角点位置
detector->detect(img_1, keypoints_1);
detector->detect(img_2, keypoints_2);
//-- 第二步:根据角点位置计算 BRIEF 描述子
descriptor->compute(img_1, keypoints_1, descriptors_1);
descriptor->compute(img_2, keypoints_2, descriptors_2);
//-- 第三步:对两幅图像中的BRIEF描述子进行匹配,使用 Hamming 距离
vector<DMatch> match; //在这个函数中创建的局部的vector<DMatch>变量,表示所有的匹配结果,之后要根据描述子之间的距离对匹配的结果进行筛选。
//BFMatcher matcher ( NORM_HAMMING );
matcher->match(descriptors_1, descriptors_2, match);
//-- 第四步:匹配点对筛选
double min_dist = 10000, max_dist = 0;
//找出所有匹配之间的最小距离和最大距离, 即是最相似的和最不相似的两组点之间的距离
for (int i = 0; i < descriptors_1.rows; i++) {
double dist = match[i].distance;
if (dist < min_dist) min_dist = dist;
if (dist > max_dist) max_dist = dist;
}
printf("-- Max dist : %f \n", max_dist);
printf("-- Min dist : %f \n", min_dist);
//当描述子之间的距离大于两倍的最小距离时,即认为匹配有误.但有时候最小距离会非常小,设置一个经验值30作为下限.
for (int i = 0; i < descriptors_1.rows; i++) {
if (match[i].distance <= max(2 * min_dist, 30.0)) {
matches.push_back(match[i]); //将匹配对添加到matches中
}
}
}
Point2d pixel2cam(const Point2d &p, const Mat &K) {
return Point2d
(
(p.x - K.at<double>(0, 2)) / K.at<double>(0, 0),
(p.y - K.at<double>(1, 2)) / K.at<double>(1, 1)
);
} //参数是像素坐标和相机内参矩阵K,返回相机归一化坐标
void pose_estimation_2d2d(std::vector<KeyPoint> keypoints_1,
std::vector<KeyPoint> keypoints_2,
std::vector<DMatch> matches,
Mat &R, Mat &t) {
// 相机内参,TUM Freiburg2
Mat K = (Mat_<double>(3, 3) << 520.9, 0, 325.1, 0, 521.0, 249.7, 0, 0, 1);
//-- 把匹配点转换为vector<Point2f>的形式
vector<Point2f> points1;
vector<Point2f> points2;
for (int i = 0; i < (int) matches.size(); i++) {
points1.push_back(keypoints_1[matches[i].queryIdx].pt);
points2.push_back(keypoints_2[matches[i].trainIdx].pt);
}
//这里的两个索引为什么是不一样的,一个是queryIdx,一个是trainIdx?
//因为在OpenCV中,当进行特征点匹配时,通常会得到匹配对(match pairs),每个匹配对包含了两个索引:queryIdx和trainIdx。即有两个图像,一个是查询图像,一个是训练图像,两个图像的特征点都有自己的索引。
//这里的pt函数返回的是KeyPoint的坐标,而KeyPoint的坐标是相对于图像左上角的,而我们需要的是相对于相机坐标系的坐标,需要首先完成一个转换。
//-- 计算基础矩阵
Mat fundamental_matrix;
fundamental_matrix = findFundamentalMat(points1, points2, CV_FM_8POINT); //points1, points2是两个容器。里面按照匹配的顺序存储了所有的特征点,所以在函数中不用显式地将匹配关系作为参数传入。
cout << "fundamental_matrix is " << endl << fundamental_matrix << endl;
//-- 计算本质矩阵
Point2d principal_point(325.1, 249.7); //相机光心, TUM dataset标定值
double focal_length = 521; //相机焦距, TUM dataset标定值
Mat essential_matrix;
essential_matrix = findEssentialMat(points1, points2, focal_length, principal_point);
cout << "essential_matrix is " << endl << essential_matrix << endl;
//-- 计算单应矩阵
//-- 但是本例中场景不是平面,单应矩阵意义不大
Mat homography_matrix;
homography_matrix = findHomography(points1, points2, RANSAC, 3);
cout << "homography_matrix is " << endl << homography_matrix << endl;
//当特征点位于一个平面上时,使用单应矩阵可以获得较好的结果。但是在本例中,场景不是平面,所以单应矩阵的效果不好。
//-- 从本质矩阵中恢复旋转和平移信息.
// 此函数仅在Opencv3中提供
recoverPose(essential_matrix, points1, points2, R, t, focal_length, principal_point);
cout << "R is " << endl << R << endl;
cout << "t is " << endl << t << endl;
}
基于2D-2D的特征点匹配的相机运动古迹,主要基于多视图几何中的对极几何部分,如下图所示。
将像素坐标转换为相机归一化坐标的原理,如下两张图所示:
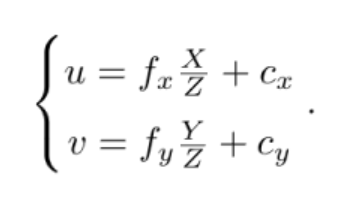
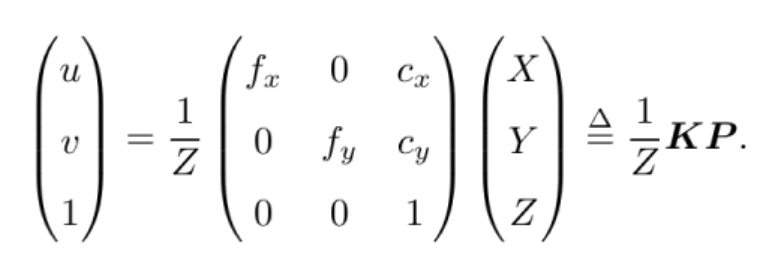
通过八点法求取本质矩阵的方法是通过八个匹配点的像素的归一化坐标对本质矩阵进行约束求解,求解的过程可以看成空间的降维,每多一层约束,解空间的维度就降一重,直到解空间降维到一维(因为本质矩阵具有齐次性),这就要求八个点构成的约束矩阵是行满秩的,但是一般来说匹配点远远超过8个,不必担心该问题。
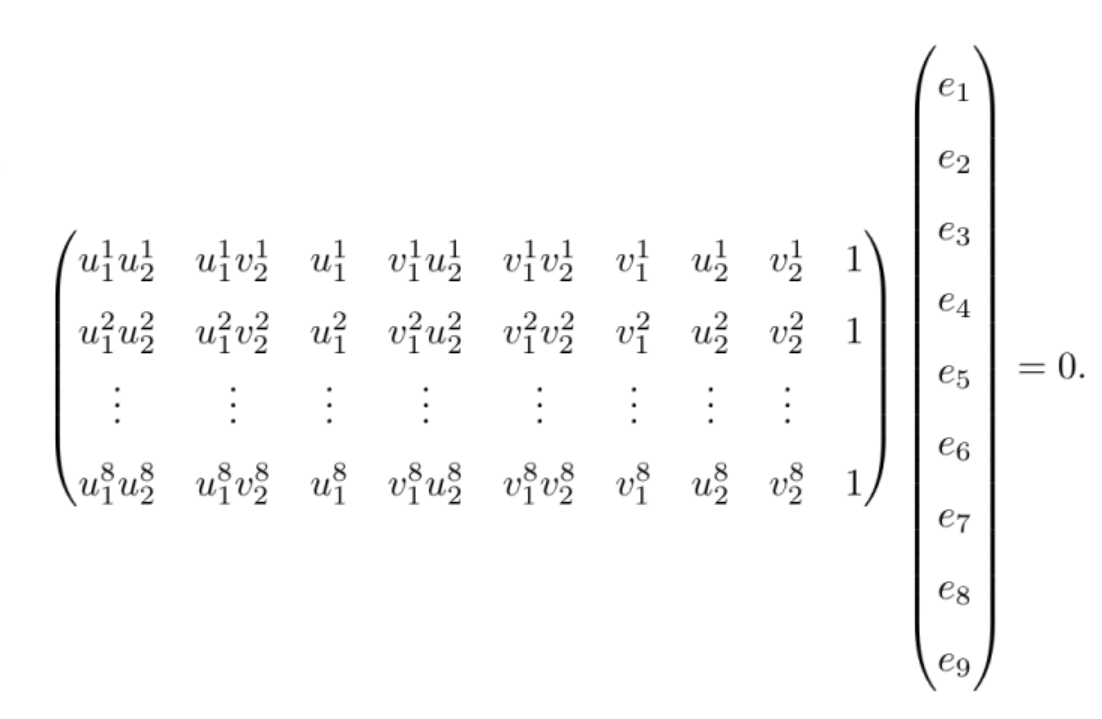
三角测量
三角测量干了什么?
三角测量通过两个相机(一个相机的两个位置,左右位置)之间的位姿关系,和它们测量的对应的特征点的归一化坐标,恢复特征点的深度信息,就像人眼恢复深度信息一样。
在前一个程序的基础上进行,前一个程序通过对极几何实现了对相机运动的估计(也就是相机之间的位姿关系),在这个程序中,利用到了该估计的结果。
在书中说求得的R和t不一定是精确的,存在误差,所以更好的方法是采用最小二乘法。如下图所示,进行外积之后,只对其中的一个相机进行了约束,其中有R和t,更好的方法是求这个约束方程的最小二乘解,而不是零解。

#include <iostream>
#include <opencv2/opencv.hpp>
// #include "extra.h" // used in opencv2
using namespace std;
using namespace cv;
void find_feature_matches(
const Mat &img_1, const Mat &img_2,
std::vector<KeyPoint> &keypoints_1,
std::vector<KeyPoint> &keypoints_2,
std::vector<DMatch> &matches);
void pose_estimation_2d2d(
const std::vector<KeyPoint> &keypoints_1,
const std::vector<KeyPoint> &keypoints_2,
const std::vector<DMatch> &matches,
Mat &R, Mat &t);
void triangulation(
const vector<KeyPoint> &keypoint_1,
const vector<KeyPoint> &keypoint_2,
const std::vector<DMatch> &matches,
const Mat &R, const Mat &t,
vector<Point3d> &points
);
/// 作图用
inline cv::Scalar get_color(float depth) {
float up_th = 50, low_th = 10, th_range = up_th - low_th;
if (depth > up_th) depth = up_th;
if (depth < low_th) depth = low_th;
return cv::Scalar(255 * depth / th_range, 0, 255 * (1 - depth / th_range));
} //这是一个作图用的函数,用来根据深度值返回颜色值,是一个内连函数
// 像素坐标转相机归一化坐标
Point2f pixel2cam(const Point2d &p, const Mat &K);
int main(int argc, char **argv) {
if (argc != 3) {
cout << "usage: triangulation img1 img2" << endl;
return 1;
}
//-- 读取图像
Mat img_1 = imread(argv[1], CV_LOAD_IMAGE_COLOR);
Mat img_2 = imread(argv[2], CV_LOAD_IMAGE_COLOR);
vector<KeyPoint> keypoints_1, keypoints_2;
vector<DMatch> matches;
find_feature_matches(img_1, img_2, keypoints_1, keypoints_2, matches);
cout << "一共找到了" << matches.size() << "组匹配点" << endl;
//-- 估计两张图像间运动
Mat R, t;
pose_estimation_2d2d(keypoints_1, keypoints_2, matches, R, t);
//-- 三角化
vector<Point3d> points;
triangulation(keypoints_1, keypoints_2, matches, R, t, points); //points用来存储三角化得到的点
//-- 验证三角化点与特征点的重投影关系
Mat K = (Mat_<double>(3, 3) << 520.9, 0, 325.1, 0, 521.0, 249.7, 0, 0, 1);
Mat img1_plot = img_1.clone();
Mat img2_plot = img_2.clone();
for (int i = 0; i < matches.size(); i++) {
// 第一个图
float depth1 = points[i].z;
cout << "depth: " << depth1 << endl;
Point2d pt1_cam = pixel2cam(keypoints_1[matches[i].queryIdx].pt, K);
cv::circle(img1_plot, keypoints_1[matches[i].queryIdx].pt, 2, get_color(depth1), 2);
//这种技术通常被用于可视化深度信息,以在图像中标记出不同深度区域的特征点或物体。
// 第二个图
Mat pt2_trans = R * (Mat_<double>(3, 1) << points[i].x, points[i].y, points[i].z) + t;
float depth2 = pt2_trans.at<double>(2, 0);
cv::circle(img2_plot, keypoints_2[matches[i].trainIdx].pt, 2, get_color(depth2), 2);
}
cv::imshow("img 1", img1_plot);
cv::imshow("img 2", img2_plot);
cv::waitKey();
return 0;
}
void find_feature_matches(const Mat &img_1, const Mat &img_2,
std::vector<KeyPoint> &keypoints_1,
std::vector<KeyPoint> &keypoints_2,
std::vector<DMatch> &matches) {
//-- 初始化
Mat descriptors_1, descriptors_2;
// used in OpenCV3
Ptr<FeatureDetector> detector = ORB::create();
Ptr<DescriptorExtractor> descriptor = ORB::create();
// use this if you are in OpenCV2
// Ptr<FeatureDetector> detector = FeatureDetector::create ( "ORB" );
// Ptr<DescriptorExtractor> descriptor = DescriptorExtractor::create ( "ORB" );
Ptr<DescriptorMatcher> matcher = DescriptorMatcher::create("BruteForce-Hamming");
//-- 第一步:检测 Oriented FAST 角点位置
detector->detect(img_1, keypoints_1);
detector->detect(img_2, keypoints_2);
//-- 第二步:根据角点位置计算 BRIEF 描述子
descriptor->compute(img_1, keypoints_1, descriptors_1);
descriptor->compute(img_2, keypoints_2, descriptors_2);
//-- 第三步:对两幅图像中的BRIEF描述子进行匹配,使用 Hamming 距离
vector<DMatch> match;
// BFMatcher matcher ( NORM_HAMMING );
matcher->match(descriptors_1, descriptors_2, match);
//-- 第四步:匹配点对筛选
double min_dist = 10000, max_dist = 0;
//找出所有匹配之间的最小距离和最大距离, 即是最相似的和最不相似的两组点之间的距离
for (int i = 0; i < descriptors_1.rows; i++) {
double dist = match[i].distance;
if (dist < min_dist) min_dist = dist;
if (dist > max_dist) max_dist = dist;
}
printf("-- Max dist : %f \n", max_dist);
printf("-- Min dist : %f \n", min_dist);
//当描述子之间的距离大于两倍的最小距离时,即认为匹配有误.但有时候最小距离会非常小,设置一个经验值30作为下限.
for (int i = 0; i < descriptors_1.rows; i++) {
if (match[i].distance <= max(2 * min_dist, 30.0)) {
matches.push_back(match[i]);
}
}
}
void pose_estimation_2d2d(
const std::vector<KeyPoint> &keypoints_1,
const std::vector<KeyPoint> &keypoints_2,
const std::vector<DMatch> &matches,
Mat &R, Mat &t) {
// 相机内参,TUM Freiburg2
Mat K = (Mat_<double>(3, 3) << 520.9, 0, 325.1, 0, 521.0, 249.7, 0, 0, 1);
//-- 把匹配点转换为vector<Point2f>的形式
vector<Point2f> points1;
vector<Point2f> points2;
for (int i = 0; i < (int) matches.size(); i++) {
points1.push_back(keypoints_1[matches[i].queryIdx].pt);
points2.push_back(keypoints_2[matches[i].trainIdx].pt);
}
//-- 计算本质矩阵
Point2d principal_point(325.1, 249.7); //相机主点, TUM dataset标定值
int focal_length = 521; //相机焦距, TUM dataset标定值
Mat essential_matrix;
essential_matrix = findEssentialMat(points1, points2, focal_length, principal_point);
//-- 从本质矩阵中恢复旋转和平移信息.
recoverPose(essential_matrix, points1, points2, R, t, focal_length, principal_point);
}
void triangulation(
const vector<KeyPoint> &keypoint_1,
const vector<KeyPoint> &keypoint_2,
const std::vector<DMatch> &matches,
const Mat &R, const Mat &t,
vector<Point3d> &points) {
Mat T1 = (Mat_<float>(3, 4) <<
1, 0, 0, 0,
0, 1, 0, 0,
0, 0, 1, 0);
Mat T2 = (Mat_<float>(3, 4) <<
R.at<double>(0, 0), R.at<double>(0, 1), R.at<double>(0, 2), t.at<double>(0, 0),
R.at<double>(1, 0), R.at<double>(1, 1), R.at<double>(1, 2), t.at<double>(1, 0),
R.at<double>(2, 0), R.at<double>(2, 1), R.at<double>(2, 2), t.at<double>(2, 0)
); //分别定义了两个相机的投影矩阵
Mat K = (Mat_<double>(3, 3) << 520.9, 0, 325.1, 0, 521.0, 249.7, 0, 0, 1);
vector<Point2f> pts_1, pts_2;
for (DMatch m:matches) { //这里用的是元素遍历方法,也可以使用下标遍历方法
// 将像素坐标转换至相机坐标
pts_1.push_back(pixel2cam(keypoint_1[m.queryIdx].pt, K));
pts_2.push_back(pixel2cam(keypoint_2[m.trainIdx].pt, K));
}
Mat pts_4d;
cv::triangulatePoints(T1, T2, pts_1, pts_2, pts_4d); //这是三角化的核心函数。用于从两个相机的投影矩阵和对应的像素坐标对(如匹配点)中计算出三维点的坐标。
//需要注意的是,输入的像素坐标应该已经进行了归一化处理,以确保函数的正确行为。
// 转换成非齐次坐标
for (int i = 0; i < pts_4d.cols; i++) { //pts_4d是按列进行排列的三维点的齐次坐标,这里将其转换成非齐次
Mat x = pts_4d.col(i);
x /= x.at<float>(3, 0); // 归一化
Point3d p(
x.at<float>(0, 0),
x.at<float>(1, 0),
x.at<float>(2, 0)
);
points.push_back(p);
}
}
Point2f pixel2cam(const Point2d &p, const Mat &K) {
return Point2f
(
(p.x - K.at<double>(0, 2)) / K.at<double>(0, 0),
(p.y - K.at<double>(1, 2)) / K.at<double>(1, 1)
);
}