网上学习资料一大堆,但如果学到的知识不成体系,遇到问题时只是浅尝辄止,不再深入研究,那么很难做到真正的技术提升。
一个人可以走的很快,但一群人才能走的更远!不论你是正从事IT行业的老鸟或是对IT行业感兴趣的新人,都欢迎加入我们的的圈子(技术交流、学习资源、职场吐槽、大厂内推、面试辅导),让我们一起学习成长!
ax = fig.add_subplot(1, 2, 2)
ax.scatter(Y[:, 0], Y[:, 1], c=color, cmap=plt.cm.Spectral)
ax.set_title("%s (%.2g sec)" % (label, t1 - t0))
ax.xaxis.set_major_formatter(NullFormatter())
ax.yaxis.set_major_formatter(NullFormatter())
ax.axis('tight')
plt.show()
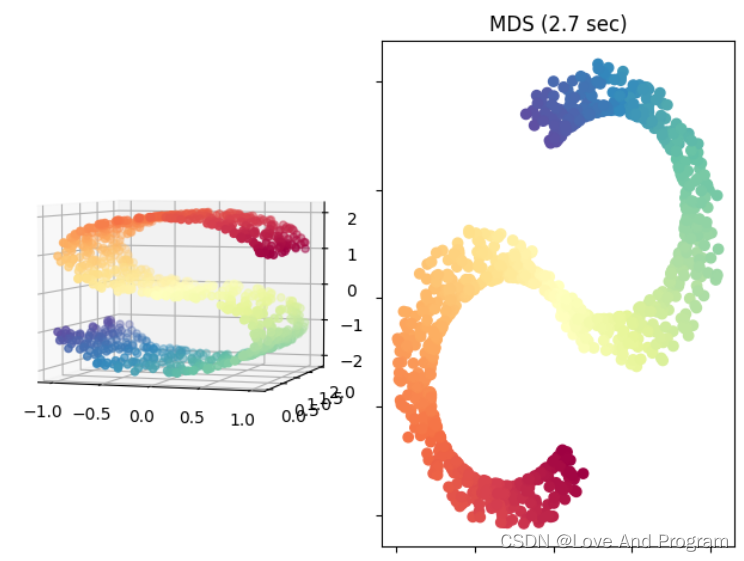
### 主成分分析法
#### 简介
主成分分析(Principal Component Analysis,PCA), 是一种统计方法,通过正交变换将一组可能存在相关性的变量转换为一组线性不相关的变量,转换后的这组变量叫主成分(来源于[百度百科](https://bbs.csdn.net/topics/618545628))。
主成分分析法适用于变量间有较强相关性的数据,毕竟数据间关系弱的,经过PCA降维后会舍弃部分信息,关系可能会更弱,那用降维的意义也就不存在了,其具体作用有两方面:
1. 舍弃此部分信息后能使样本的采样密度增大,正如上面所说:高维数据到低维数据转换后数据间的密度会大大提高;
2. 当数据受到噪声影响时,最小的特征值所对应的特征向量往往与噪声有关,将它们舍弃可以在一定程度上起到去噪的效果;
**算法描述**
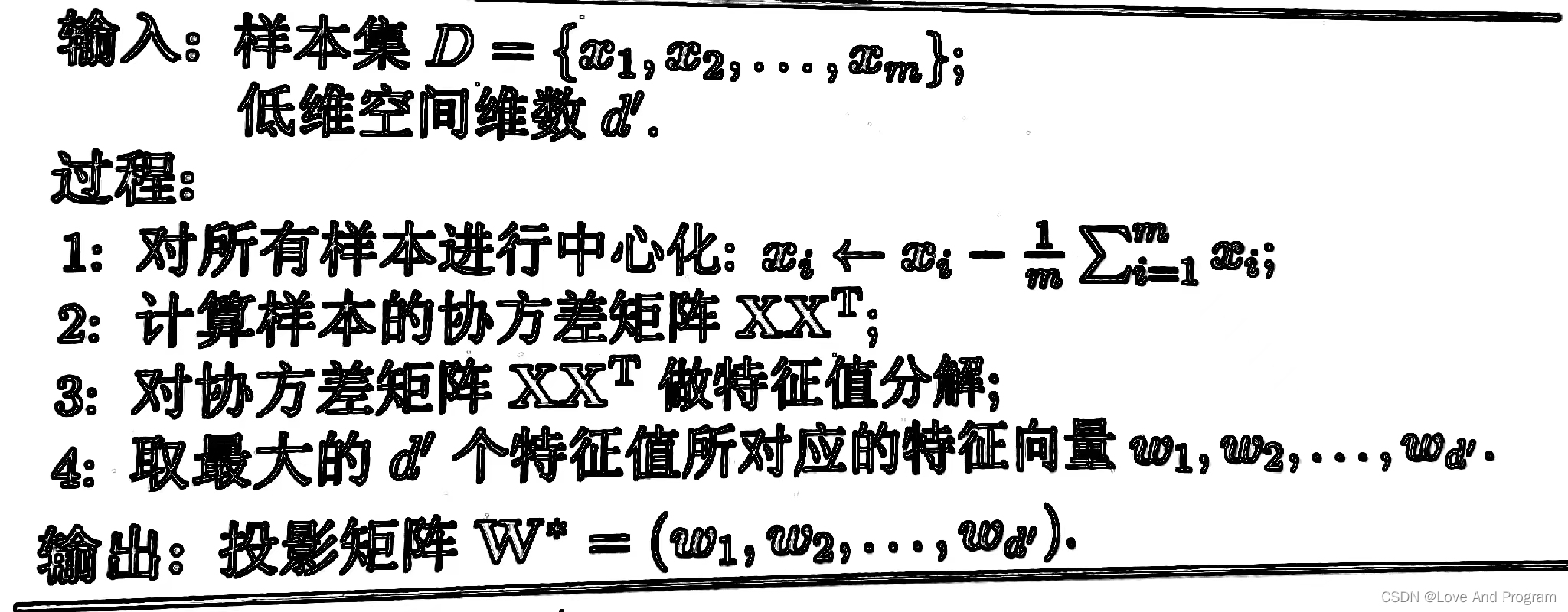
### 核化线性降维
#### 简介
核化线性降维是非线性降维,是基于核技巧对线性降维方法进行“核化”,例如核主成分分析(KPCA),优点就是,保留了最主要的特征,计算方法简单,但缺点也很明显,使用非线性核可以实现非线性降维计算开销极大,直接给我报Memory Error,以下代码请酌情更改输入数据集比例。
### **PCA和KPCA手写数字体识别实例代码**
from sklearn.model_selection import train_test_split
import pandas as pd
from sklearn.decomposition import KernelPCA
from sklearn.datasets import fetch_openml
mnist = fetch_openml(‘mnist_784’, cache=True)
print(mnist.data.shape)
import matplotlib.pyplot as plt
def display_sample(num):
image = mnist.data.iloc[num,:].to_numpy().reshape([28,28])
plt.imshow(image, cmap=plt.get_cmap('gray\_r'))
plt.show()
display_sample(10)
train_img, test_img, train_lbl, test_lbl = train_test_split(
mnist.data, mnist.target, test_size=0.8, random_state=0)
print(train_img.shape)
print(test_img.shape)
from sklearn.preprocessing import StandardScaler
scaler = StandardScaler()
scaler.fit(train_img)
train_img = scaler.transform(train_img)
test_img = scaler.transform(test_img)
from sklearn.linear_model import LogisticRegression
logisticRegr = LogisticRegression(solver = ‘lbfgs’,max_iter=1000)
logisticRegr.fit(train_img, train_lbl)
score = logisticRegr.score(test_img, test_lbl)
print(score)
KPCA
scikit_kpca = KernelPCA(n_components=2, kernel=‘rbf’, gamma=15)
X_skernpca = scikit_kpca.fit_transform(train_img)
plt.scatter(X_skernpca[test_img, 0], X_skernpca[test_img, 1], color=‘red’, marker=‘^’, alpha=0.5)
plt.scatter(X_skernpca[test_lbl, 0], X_skernpca[test_lbl==1, 1], color=‘blue’, marker=‘o’, alpha=0.5)
plt.xlabel(‘PC1’)
plt.ylabel(‘PC2’)
plt.tight_layout()
plt.show()
PCA
from sklearn.decomposition import PCA
pca = PCA(0.95)
pca.fit(train_img)
print(pca.n_components_)
train2_img = pca.transform(train_img)
test2_img = pca.transform(test_img)
print(train2_img.shape)
print(train_img.shape)
logisticRegr = LogisticRegression(solver = ‘lbfgs’,max_iter=1000)
logisticRegr.fit(train2_img, train_lbl)
score = logisticRegr.score(test2_img, test_lbl)
print(score)
#minist数据集及划分比例
#(70000, 784)
#(14000, 784)
#(56000, 784)
#降维前预测准确率
#0.8843214285714286
#保留特征数量
#293
#降维后数据量对比
#(14000, 293)
#(14000, 784)
#降维后预测准确率
#0.9033214285714286
---
## 流形学习
### 简介
流形学习是一类借鉴拓扑流形概念的降维方法,“流形”是在局部与欧氏空间同胚的空间,也就是说他在局部具有欧氏空间的性质,能用欧氏距离来进行距离计算,用到降维中,即低维流形嵌入到高维空间中,局部仍然具有欧氏空间的性质,因此很容易在局部建立降维映射关系,然后在设法将局部映射关系推广到全局,以两种著名的流形学习方法为例,即非线性降维。
### 等度量映射(Isomap)
>
> Isomap算法是最早的流形学习方法之一,它是等距映射的缩写。Isomap可以看作是多维尺度分析(MDS)或核主成分分析的扩展。
>
>
>
#### 简介
Isomap寻求一种更低维度的嵌入,以保持所有点之间的测地线距离,毕竟如下左图最上面一层到中间一层在三维坐标中不能直接计算直线距离,这样是不恰当的,需要沿着两层之间的边边连线形成距离最短的路径,也就是测地线距离,虽然低维嵌入流形上的测地线距离不能用高维空间的直线距离计算,但是可以通过近邻距离来求近似(图画的不是很好,右图线都是直的嗷,蓝线:近邻距离,也就是左图三维直线距离被二维可视化;红线:测地线距离)
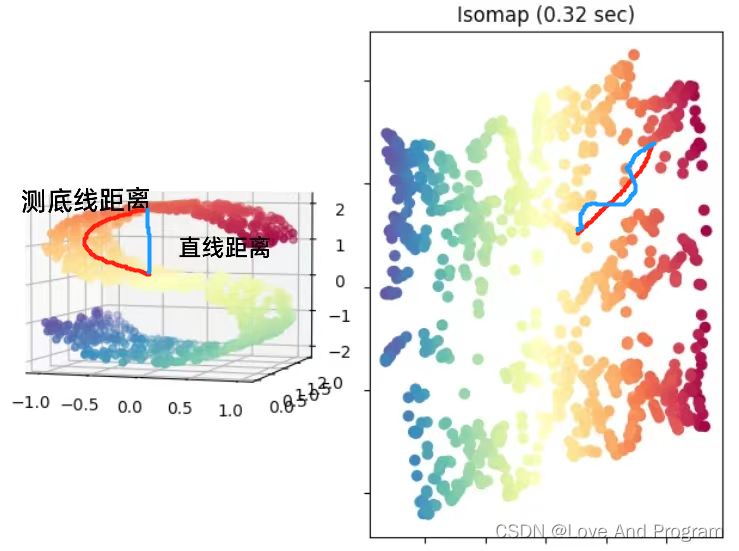
**算法描述**
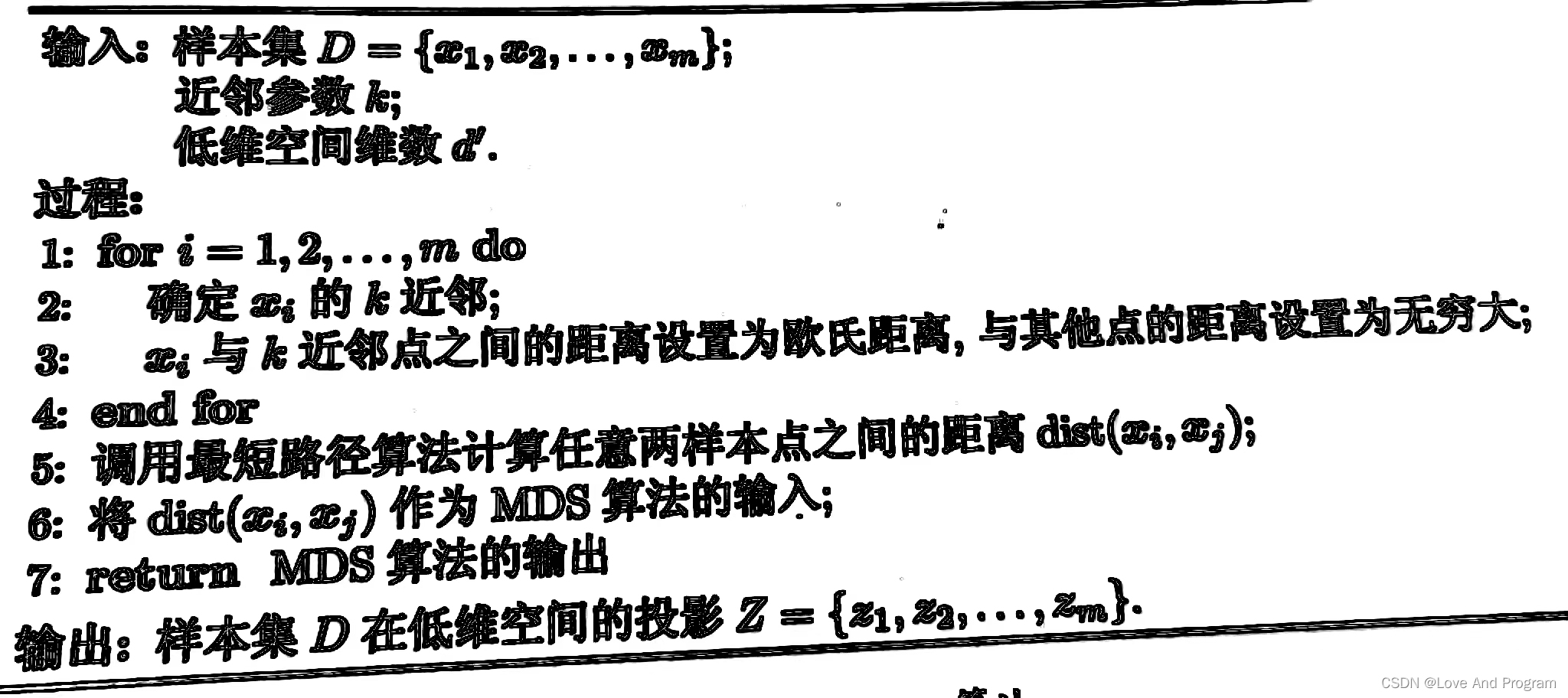
#### **Isomap示例代码**
from collections import OrderedDict
from functools import partial
from time import time
import matplotlib.pyplot as plt
from mpl_toolkits.mplot3d import Axes3D
from matplotlib.ticker import NullFormatter
from sklearn import manifold, datasets
Next line to silence pyflakes. This import is needed.
Axes3D
n_points = 1000
X, color = datasets.make_s_curve(n_points, random_state=0)
fig = plt.figure()
ax = fig.add_subplot(121, projection=‘3d’)
ax.scatter(X[:, 0], X[:, 1], X[:, 2], c=color, cmap=plt.cm.Spectral)
ax.view_init(4, -72)
methods = OrderedDict()
methods[‘Isomap’] = manifold.Isomap(n_components=2)
for i, (label, method) in enumerate(methods.items()):
t0 = time()
Y = method.fit_transform(X)
t1 = time()
print(“%s: %.2g sec” % (label, t1 - t0))
ax = fig.add_subplot(1, 2, 2)
ax.scatter(Y[:, 0], Y[:, 1], c=color, cmap=plt.cm.Spectral)
ax.set_title(“%s (%.2g sec)” % (label, t1 - t0))
ax.xaxis.set_major_formatter(NullFormatter())
ax.yaxis.set_major_formatter(NullFormatter())
ax.axis(‘tight’)
plt.show()
以S型曲线数据集为例,将三维数据映射到二维平面上,如下图所示:
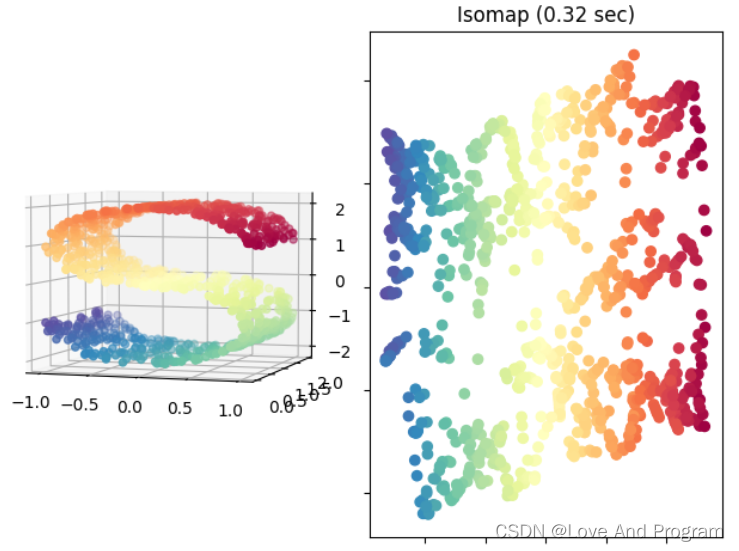
### 局部线性嵌入(LLE)
#### 简介
局部线性嵌入试图保持邻域内样本线性关系,意思就是经历过降维后仍然在原空间具有该特征。并且LLE可以在sklearn机器学习库中直接调用。
**算法描述:**
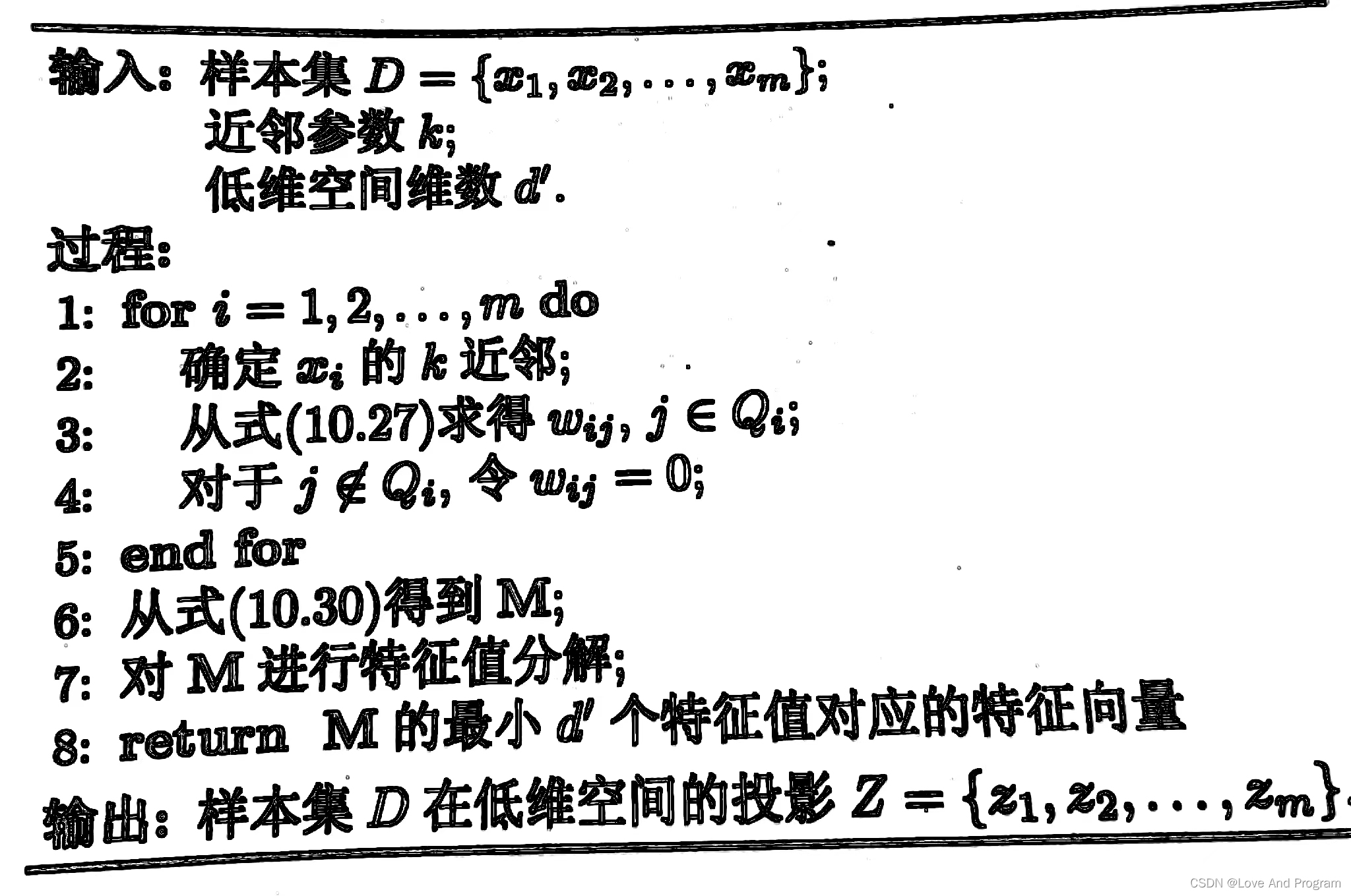
| | |
| --- | --- |
|
| 式(10.27) |
| | 式(10.30) |
[推导过程(感兴趣可以去看看)](https://bbs.csdn.net/topics/618545628)
#### **LLE示例代码**
import matplotlib.pyplot as plt
from mpl_toolkits.mplot3d import Axes3D
Axes3D
from sklearn import manifold, datasets
X, color = datasets.make_swiss_roll(n_samples=1500)
print(“Computing LLE embedding”)
X_r, err = manifold.locally_linear_embedding(X, n_neighbors=12,
网上学习资料一大堆,但如果学到的知识不成体系,遇到问题时只是浅尝辄止,不再深入研究,那么很难做到真正的技术提升。
一个人可以走的很快,但一群人才能走的更远!不论你是正从事IT行业的老鸟或是对IT行业感兴趣的新人,都欢迎加入我们的的圈子(技术交流、学习资源、职场吐槽、大厂内推、面试辅导),让我们一起学习成长!
-1715738656639)]
网上学习资料一大堆,但如果学到的知识不成体系,遇到问题时只是浅尝辄止,不再深入研究,那么很难做到真正的技术提升。
一个人可以走的很快,但一群人才能走的更远!不论你是正从事IT行业的老鸟或是对IT行业感兴趣的新人,都欢迎加入我们的的圈子(技术交流、学习资源、职场吐槽、大厂内推、面试辅导),让我们一起学习成长!