2 conf的损失函数:Log loss
y_true shape:(batch_size, n_boxes, n_classes)
def log_loss(self, y_true, y_pred):
# 确保y_pred中不含0,否则会使log函数崩溃的
y_pred = tf.maximum(y_pred, 1e-15)
# Compute the log loss
log_loss = -tf.reduce_sum(y_true * tf.log(y_pred), axis=-1)
return log_loss
3 hard negative mining
主要思路:
1.根据正样本的个数和正负比例,确定负样本的个数,negative_keep
2.找到confidence loss最大的negative_keep个负样本,计算他们的分类损失之和
3.计算正样本的分类损失之和,分类损失是正样本和负样本的损失和
4.计算正样本的位置损失localization loss.无法计算负样本位置损失 %>_<%
- 对回归损失和位置损失之和
def compute_loss(self, y_true, y_pred):
self.neg_pos_ratio = tf.constant(self.neg_pos_ratio)
self.n_neg_min = tf.constant(self.n_neg_min)
self.alpha = tf.constant(self.alpha)
batch_size = tf.shape(y_pred)[0] # Output dtype: tf.int32
n_boxes = tf.shape(y_pred)[1]
# Output dtype: tf.int32, note that `n_boxes` in this context denotes the total number of boxes per image, not the number of boxes per cell.
## 计算每个box的类别和框的损失
classification_loss = tf.to_float(self.log_loss(y_true[:,:,:-12], y_pred[:,:,:-12]))
# Output shape: (batch_size, n_boxes)
localization_loss = tf.to_float(self.smooth_L1_loss(y_true[:,:,-12:-8], y_pred[:,:,-12:-8]))
# Output shape: (batch_size, n_boxes)
## 为正的和负的groud truth 制作mask
#此时需要对y_true提前进行编码。
#对于类别只有所属的类别是1,其他全是0,对于出ground truth之外的box的类别,背景设为1,其余全设为0
negatives = y_true[:,:,0] # Tensor of shape (batch_size, n_boxes)
positives = tf.to_float(tf.reduce_max(y_true[:,:,1:-12], axis=-1))
# Tensor of shape (batch_size, n_boxes)
#统计正样本的个数
n_positive = tf.reduce_sum(positives)
# 掩盖负的box,计算正样本box的损失之和
pos_class_loss = tf.reduce_sum(classification_loss * positives, axis=-1) # Tensor of shape (batch_size,)
# 计算所有负样本的box的损失之和
neg_class_loss_all = classification_loss * negatives # Tensor of shape (batch_size, n_boxes)
#计算损失非零的负样本的个数
n_neg_losses = tf.count_nonzero(neg_class_loss_all, dtype=tf.int32) # The number of non-zero loss entries in `neg_class_loss_all`
# Compute the number of negative examples we want to account for in the loss.
# 至多保留 `self.neg_pos_ratio` 倍于 y_true中正样本的数量, 至少保留 n_neg_min个负样本 per batch.
n_negative_keep = tf.minimum(tf.maximum(self.neg_pos_ratio * tf.to_int32(n_positive), self.n_neg_min), n_neg_losses)
def f1():
'''
当不存在负样本的ground truth时,直接返回0
'''
**自我介绍一下,小编13年上海交大毕业,曾经在小公司待过,也去过华为、OPPO等大厂,18年进入阿里一直到现在。**
**深知大多数Linux运维工程师,想要提升技能,往往是自己摸索成长或者是报班学习,但对于培训机构动则几千的学费,着实压力不小。自己不成体系的自学效果低效又漫长,而且极易碰到天花板技术停滞不前!**
**因此收集整理了一份《2024年Linux运维全套学习资料》,初衷也很简单,就是希望能够帮助到想自学提升又不知道该从何学起的朋友,同时减轻大家的负担。**
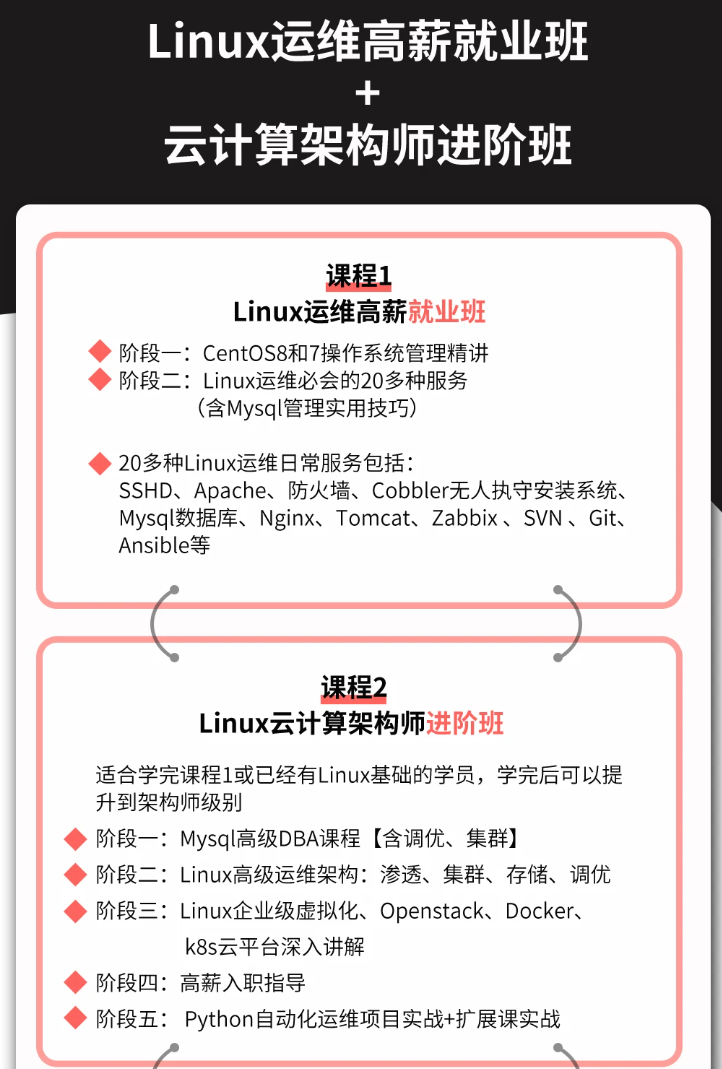
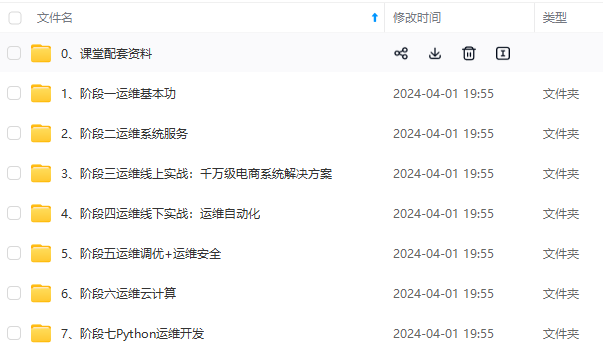
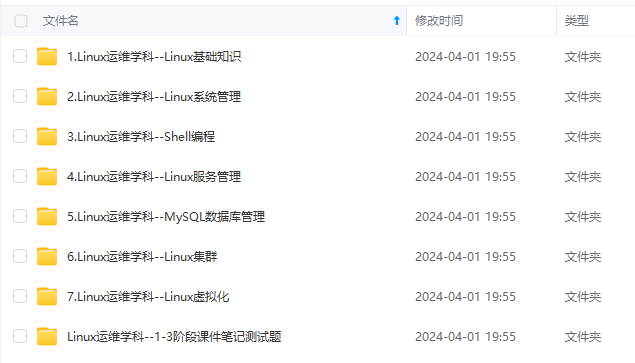
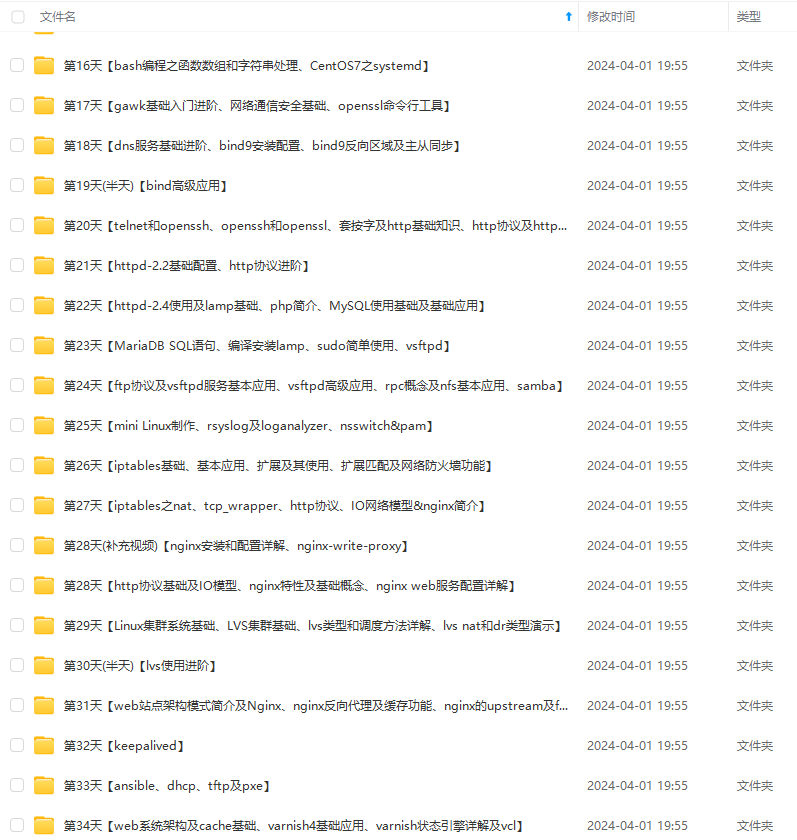
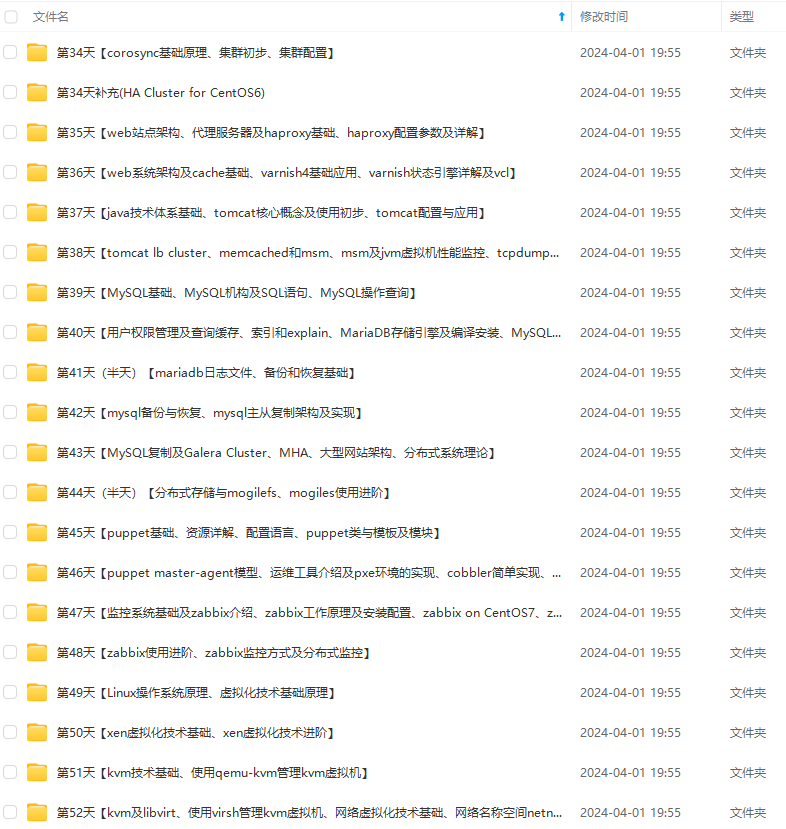
**既有适合小白学习的零基础资料,也有适合3年以上经验的小伙伴深入学习提升的进阶课程,基本涵盖了95%以上Linux运维知识点,真正体系化!**
**由于文件比较大,这里只是将部分目录大纲截图出来,每个节点里面都包含大厂面经、学习笔记、源码讲义、实战项目、讲解视频,并且后续会持续更新**
**如果你觉得这些内容对你有帮助,可以添加VX:vip1024b (备注Linux运维获取)**
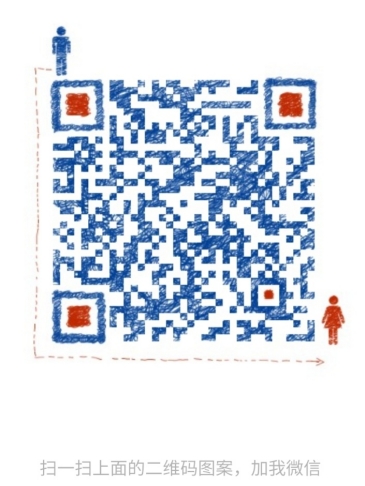
会持续更新**
**如果你觉得这些内容对你有帮助,可以添加VX:vip1024b (备注Linux运维获取)**
[外链图片转存中...(img-sWpNNV91-1712766826207)]