请申请一个免费 T4 GPU 来运行该脚本
详细讲上面连接。需要科学上网
微调过程大约需要 50 分钟。
训练脚本:
from llmtuner import run_exp
%cd /content/LLaMA-Factory/
run_exp(dict(
stage=“sft”,
do_train=True,
model_name_or_path=“unsloth/llama-3-8b-Instruct-bnb-4bit”,
dataset=“identity,alpaca_gpt4_en,alpaca_gpt4_zh”,
template=“llama3”,
finetuning_type=“lora”,
lora_target=“all”,
output_dir=“llama3_lora”,
per_device_train_batch_size=2,
gradient_accumulation_steps=4,
lr_scheduler_type=“cosine”,
logging_steps=10,
warmup_ratio=0.1,
save_steps=1000,
learning_rate=5e-5,
num_train_epochs=3.0,
max_samples=500,
max_grad_norm=1.0,
quantization_bit=4,
loraplus_lr_ratio=16.0,
use_unsloth=True,
fp16=True,
))
训练过程日志
04/22/2024 04:10:40 - WARNING - llmtuner.hparams.parser - We recommend enable `upcast_layernorm` in quantized training.
WARNING:llmtuner.hparams.parser:We recommend enable `upcast_layernorm` in quantized training.
04/22/2024 04:10:40 - INFO - llmtuner.hparams.parser - Process rank: 0, device: cuda:0, n_gpu: 1, distributed training: False, compute dtype: torch.float16
INFO:llmtuner.hparams.parser:Process rank: 0, device: cuda:0, n_gpu: 1, distributed training: False, compute dtype: torch.float16
/usr/local/lib/python3.10/dist-packages/huggingface_hub/utils/_token.py:89: UserWarning:
The secret `HF_TOKEN` does not exist in your Colab secrets.
To authenticate with the Hugging Face Hub, create a token in your settings tab (<https://huggingface.co/settings/tokens>), set it as secret in your Google Colab and restart your session.
You will be able to reuse this secret in all of your notebooks.
Please note that authentication is recommended but still optional to access public models or datasets.
warnings.warn(
[INFO|tokenization_utils_base.py:2046] 2024-04-22 04:10:41,979 >> loading file tokenizer.json from cache at /root/.cache/huggingface/hub/models--unsloth--llama-3-8b-Instruct-bnb-4bit/snapshots/3fe39f383d040c52882f7f9faaf600dc57c01a8d/tokenizer.json
[INFO|tokenization_utils_base.py:2046] 2024-04-22 04:10:41,980 >> loading file added_tokens.json from cache at None
[INFO|tokenization_utils_base.py:2046] 2024-04-22 04:10:41,982 >> loading file special_tokens_map.json from cache at /root/.cache/huggingface/hub/models--unsloth--llama-3-8b-Instruct-bnb-4bit/snapshots/3fe39f383d040c52882f7f9faaf600dc57c01a8d/special_tokens_map.json
[INFO|tokenization_utils_base.py:2046] 2024-04-22 04:10:41,984 >> loading file tokenizer_config.json from cache at /root/.cache/huggingface/hub/models--unsloth--llama-3-8b-Instruct-bnb-4bit/snapshots/3fe39f383d040c52882f7f9faaf600dc57c01a8d/tokenizer_config.json
[WARNING|logging.py:314] 2024-04-22 04:10:42,384 >> Special tokens have been added in the vocabulary, make sure the associated word embeddings are fine-tuned or trained.
04/22/2024 04:10:42 - INFO - llmtuner.data.template - Replace eos token: <|eot_id|>
INFO:llmtuner.data.template:Replace eos token: <|eot_id|>
04/22/2024 04:10:42 - INFO - llmtuner.data.loader - Loading dataset identity.json...
INFO:llmtuner.data.loader:Loading dataset identity.json...
04/22/2024 04:10:42 - WARNING - llmtuner.data.utils - Checksum failed: mismatched SHA-1 hash value at data/identity.json.
WARNING:llmtuner.data.utils:Checksum failed: mismatched SHA-1 hash value at data/identity.json.
Generating train split:
91/0 [00:00<00:00, 1640.44 examples/s]
Converting format of dataset: 100%
91/91 [00:00<00:00, 2822.67 examples/s]
04/22/2024 04:10:42 - INFO - llmtuner.data.loader - Loading dataset alpaca_gpt4_data_en.json...
INFO:llmtuner.data.loader:Loading dataset alpaca_gpt4_data_en.json...
Generating train split:
52002/0 [00:00<00:00, 117346.95 examples/s]
Converting format of dataset: 100%
500/500 [00:00<00:00, 14816.36 examples/s]
04/22/2024 04:10:43 - INFO - llmtuner.data.loader - Loading dataset alpaca_gpt4_data_zh.json...
INFO:llmtuner.data.loader:Loading dataset alpaca_gpt4_data_zh.json...
Generating train split:
48818/0 [00:00<00:00, 91511.83 examples/s]
Converting format of dataset: 100%
500/500 [00:00<00:00, 11785.79 examples/s]
Running tokenizer on dataset: 100%
1091/1091 [00:00<00:00, 1358.62 examples/s]
[INFO|configuration_utils.py:728] 2024-04-22 04:10:45,417 >> loading configuration file config.json from cache at /root/.cache/huggingface/hub/models--unsloth--llama-3-8b-Instruct-bnb-4bit/snapshots/3fe39f383d040c52882f7f9faaf600dc57c01a8d/config.json
[INFO|configuration_utils.py:791] 2024-04-22 04:10:45,419 >> Model config LlamaConfig {
"_name_or_path": "unsloth/llama-3-8b-Instruct-bnb-4bit",
"architectures": [
"LlamaForCausalLM"
],
"attention_bias": false,
"attention_dropout": 0.0,
"bos_token_id": 128000,
"eos_token_id": 128001,
"hidden_act": "silu",
"hidden_size": 4096,
"initializer_range": 0.02,
"intermediate_size": 14336,
"max_position_embeddings": 8192,
"model_type": "llama",
"num_attention_heads": 32,
"num_hidden_layers": 32,
"num_key_value_heads": 8,
"pretraining_tp": 1,
"quantization_config": {
"_load_in_4bit": true,
"_load_in_8bit": false,
"bnb_4bit_compute_dtype": "bfloat16",
"bnb_4bit_quant_type": "nf4",
"bnb_4bit_use_double_quant": true,
"llm_int8_enable_fp32_cpu_offload": false,
"llm_int8_has_fp16_weight": false,
"llm_int8_skip_modules": null,
"llm_int8_threshold": 6.0,
"load_in_4bit": true,
"load_in_8bit": false,
"quant_method": "bitsandbytes"
},
"rms_norm_eps": 1e-05,
"rope_scaling": null,
"rope_theta": 500000.0,
"tie_word_embeddings": false,
"torch_dtype": "bfloat16",
"transformers_version": "4.38.2",
"use_cache": true,
"vocab_size": 128256
}
input_ids:
[128000, 128006, 9125, 128007, 271, 2675, 527, 264, 11190, 18328, 13, 128009, 128006, 882, 128007, 271, 6151, 128009, 128006, 78191, 128007, 271, 9906, 0, 358, 1097, 445, 81101, 30653, 7496, 11, 459, 15592, 18328, 8040, 555, 445, 8921, 4940, 17367, 13, 2650, 649, 358, 7945, 499, 3432, 30, 128009]
inputs:
<|begin_of_text|><|start_header_id|>system<|end_header_id|>
You are a helpful assistant.<|eot_id|><|start_header_id|>user<|end_header_id|>
hi<|eot_id|><|start_header_id|>assistant<|end_header_id|>
Hello! I am Llama-Chinese, an AI assistant developed by LLaMA Factory. How can I assist you today?<|eot_id|>
label_ids:
[-100, -100, -100, -100, -100, -100, -100, -100, -100, -100, -100, -100, -100, -100, -100, -100, -100, -100, -100, -100, -100, -100, 9906, 0, 358, 1097, 445, 81101, 30653, 7496, 11, 459, 15592, 18328, 8040, 555, 445, 8921, 4940, 17367, 13, 2650, 649, 358, 7945, 499, 3432, 30, 128009]
labels:
Hello! I am Llama-Chinese, an AI assistant developed by LLaMA Factory. How can I assist you today?<|eot_id|>
04/22/2024 04:10:45 - INFO - llmtuner.model.patcher - Loading ?-bit BITSANDBYTES-quantized model.
INFO:llmtuner.model.patcher:Loading ?-bit BITSANDBYTES-quantized model.
[INFO|configuration_utils.py:728] 2024-04-22 04:10:45,579 >> loading configuration file config.json from cache at /root/.cache/huggingface/hub/models--unsloth--llama-3-8b-Instruct-bnb-4bit/snapshots/3fe39f383d040c52882f7f9faaf600dc57c01a8d/config.json
[INFO|configuration_utils.py:791] 2024-04-22 04:10:45,581 >> Model config LlamaConfig {
"_name_or_path": "unsloth/llama-3-8b-Instruct-bnb-4bit",
"architectures": [
"LlamaForCausalLM"
],
"attention_bias": false,
"attention_dropout": 0.0,
"bos_token_id": 128000,
"eos_token_id": 128001,
"hidden_act": "silu",
"hidden_size": 4096,
"initializer_range": 0.02,
"intermediate_size": 14336,
"max_position_embeddings": 8192,
"model_type": "llama",
"num_attention_heads": 32,
"num_hidden_layers": 32,
"num_key_value_heads": 8,
"pretraining_tp": 1,
"quantization_config": {
"_load_in_4bit": true,
"_load_in_8bit": false,
"bnb_4bit_compute_dtype": "bfloat16",
"bnb_4bit_quant_type": "nf4",
"bnb_4bit_use_double_quant": true,
"llm_int8_enable_fp32_cpu_offload": false,
"llm_int8_has_fp16_weight": false,
"llm_int8_skip_modules": null,
"llm_int8_threshold": 6.0,
"load_in_4bit": true,
"load_in_8bit": false,
"quant_method": "bitsandbytes"
},
"rms_norm_eps": 1e-05,
"rope_scaling": null,
"rope_theta": 500000.0,
"tie_word_embeddings": false,
"torch_dtype": "bfloat16",
"transformers_version": "4.38.2",
"use_cache": true,
"vocab_size": 128256
}
[INFO|configuration_utils.py:728] 2024-04-22 04:10:45,634 >> loading configuration file config.json from cache at /root/.cache/huggingface/hub/models--unsloth--llama-3-8b-Instruct-bnb-4bit/snapshots/3fe39f383d040c52882f7f9faaf600dc57c01a8d/config.json
[INFO|configuration_utils.py:791] 2024-04-22 04:10:45,636 >> Model config LlamaConfig {
"_name_or_path": "unsloth/llama-3-8b-Instruct-bnb-4bit",
"architectures": [
"LlamaForCausalLM"
],
"attention_bias": false,
"attention_dropout": 0.0,
"bos_token_id": 128000,
"eos_token_id": 128001,
"hidden_act": "silu",
"hidden_size": 4096,
"initializer_range": 0.02,
"intermediate_size": 14336,
"max_position_embeddings": 8192,
"model_type": "llama",
"num_attention_heads": 32,
"num_hidden_layers": 32,
"num_key_value_heads": 8,
"pretraining_tp": 1,
"quantization_config": {
"_load_in_4bit": true,
"_load_in_8bit": false,
"bnb_4bit_compute_dtype": "bfloat16",
"bnb_4bit_quant_type": "nf4",
"bnb_4bit_use_double_quant": true,
"llm_int8_enable_fp32_cpu_offload": false,
"llm_int8_has_fp16_weight": false,
"llm_int8_skip_modules": null,
"llm_int8_threshold": 6.0,
"load_in_4bit": true,
"load_in_8bit": false,
"quant_method": "bitsandbytes"
},
"rms_norm_eps": 1e-05,
"rope_scaling": null,
"rope_theta": 500000.0,
"tie_word_embeddings": false,
"torch_dtype": "bfloat16",
"transformers_version": "4.38.2",
"use_cache": true,
"vocab_size": 128256
}
[INFO|configuration_utils.py:728] 2024-04-22 04:10:45,702 >> loading configuration file config.json from cache at /root/.cache/huggingface/hub/models--unsloth--llama-3-8b-Instruct-bnb-4bit/snapshots/3fe39f383d040c52882f7f9faaf600dc57c01a8d/config.json
[INFO|configuration_utils.py:791] 2024-04-22 04:10:45,704 >> Model config LlamaConfig {
"_name_or_path": "unsloth/llama-3-8b-Instruct-bnb-4bit",
"architectures": [
"LlamaForCausalLM"
],
"attention_bias": false,
"attention_dropout": 0.0,
"bos_token_id": 128000,
"eos_token_id": 128001,
"hidden_act": "silu",
"hidden_size": 4096,
"initializer_range": 0.02,
"intermediate_size": 14336,
"max_position_embeddings": 8192,
"model_type": "llama",
"num_attention_heads": 32,
"num_hidden_layers": 32,
"num_key_value_heads": 8,
"pretraining_tp": 1,
"quantization_config": {
"_load_in_4bit": true,
"_load_in_8bit": false,
"bnb_4bit_compute_dtype": "bfloat16",
"bnb_4bit_quant_type": "nf4",
"bnb_4bit_use_double_quant": true,
"llm_int8_enable_fp32_cpu_offload": false,
"llm_int8_has_fp16_weight": false,
"llm_int8_skip_modules": null,
"llm_int8_threshold": 6.0,
"load_in_4bit": true,
"load_in_8bit": false,
"quant_method": "bitsandbytes"
},
"rms_norm_eps": 1e-05,
"rope_scaling": null,
"rope_theta": 500000.0,
"tie_word_embeddings": false,
"torch_dtype": "float16",
"transformers_version": "4.38.2",
"use_cache": true,
"vocab_size": 128256
}
==((====))== Unsloth: Fast Llama patching release 2024.4
\\ /| GPU: Tesla T4. Max memory: 14.748 GB. Platform = Linux.
O^O/ \_/ \ Pytorch: 2.2.1+cu121. CUDA = 7.5. CUDA Toolkit = 12.1.
\ / Bfloat16 = FALSE. Xformers = 0.0.25.post1. FA = False.
"-____-" Free Apache license: [GitHub - unslothai/unsloth: Finetune Llama 3, Mistral & Gemma LLMs 2-5x faster with 80% less memory](http://github.com/unslothai/unsloth "GitHub - unslothai/unsloth: Finetune Llama 3, Mistral & Gemma LLMs 2-5x faster with 80% less memory")
[INFO|modeling_utils.py:3257] 2024-04-22 04:10:45,813 >> loading weights file model.safetensors from cache at /root/.cache/huggingface/hub/models--unsloth--llama-3-8b-Instruct-bnb-4bit/snapshots/3fe39f383d040c52882f7f9faaf600dc57c01a8d/model.safetensors
[INFO|modeling_utils.py:1400] 2024-04-22 04:10:45,863 >> Instantiating LlamaForCausalLM model under default dtype torch.float16.
[INFO|configuration_utils.py:845] 2024-04-22 04:10:45,871 >> Generate config GenerationConfig {
"bos_token_id": 128000,
"eos_token_id": 128001
}
[INFO|modeling_utils.py:3992] 2024-04-22 04:11:13,469 >> All model checkpoint weights were used when initializing LlamaForCausalLM.
[INFO|modeling_utils.py:4000] 2024-04-22 04:11:13,472 >> All the weights of LlamaForCausalLM were initialized from the model checkpoint at unsloth/llama-3-8b-Instruct-bnb-4bit.
If your task is similar to the task the model of the checkpoint was trained on, you can already use LlamaForCausalLM for predictions without further training.
[INFO|configuration_utils.py:800] 2024-04-22 04:11:13,539 >> loading configuration file generation_config.json from cache at /root/.cache/huggingface/hub/models--unsloth--llama-3-8b-Instruct-bnb-4bit/snapshots/3fe39f383d040c52882f7f9faaf600dc57c01a8d/generation_config.json
[INFO|configuration_utils.py:845] 2024-04-22 04:11:13,540 >> Generate config GenerationConfig {
"bos_token_id": 128000,
"eos_token_id": 128001
}
tokenizer_config.json: 100%
51.0k/51.0k [00:00<00:00, 2.14MB/s]
tokenizer.json: 100%
9.08M/9.08M [00:00<00:00, 60.7MB/s]
special_tokens_map.json: 100%
449/449 [00:00<00:00, 31.3kB/s]
[INFO|tokenization_utils_base.py:2046] 2024-04-22 04:11:14,466 >> loading file tokenizer.json from cache at huggingface_tokenizers_cache/models--unsloth--llama-3-8b-Instruct-bnb-4bit/snapshots/3fe39f383d040c52882f7f9faaf600dc57c01a8d/tokenizer.json
[INFO|tokenization_utils_base.py:2046] 2024-04-22 04:11:14,468 >> loading file added_tokens.json from cache at None
[INFO|tokenization_utils_base.py:2046] 2024-04-22 04:11:14,469 >> loading file special_tokens_map.json from cache at huggingface_tokenizers_cache/models--unsloth--llama-3-8b-Instruct-bnb-4bit/snapshots/3fe39f383d040c52882f7f9faaf600dc57c01a8d/special_tokens_map.json
[INFO|tokenization_utils_base.py:2046] 2024-04-22 04:11:14,472 >> loading file tokenizer_config.json from cache at huggingface_tokenizers_cache/models--unsloth--llama-3-8b-Instruct-bnb-4bit/snapshots/3fe39f383d040c52882f7f9faaf600dc57c01a8d/tokenizer_config.json
[WARNING|logging.py:314] 2024-04-22 04:11:14,881 >> Special tokens have been added in the vocabulary, make sure the associated word embeddings are fine-tuned or trained.
[INFO|tokenization_utils_base.py:2046] 2024-04-22 04:11:14,935 >> loading file tokenizer.json from cache at huggingface_tokenizers_cache/models--unsloth--llama-3-8b-Instruct-bnb-4bit/snapshots/3fe39f383d040c52882f7f9faaf600dc57c01a8d/tokenizer.json
[INFO|tokenization_utils_base.py:2046] 2024-04-22 04:11:14,936 >> loading file added_tokens.json from cache at None
[INFO|tokenization_utils_base.py:2046] 2024-04-22 04:11:14,937 >> loading file special_tokens_map.json from cache at huggingface_tokenizers_cache/models--unsloth--llama-3-8b-Instruct-bnb-4bit/snapshots/3fe39f383d040c52882f7f9faaf600dc57c01a8d/special_tokens_map.json
[INFO|tokenization_utils_base.py:2046] 2024-04-22 04:11:14,939 >> loading file tokenizer_config.json from cache at huggingface_tokenizers_cache/models--unsloth--llama-3-8b-Instruct-bnb-4bit/snapshots/3fe39f383d040c52882f7f9faaf600dc57c01a8d/tokenizer_config.json
[WARNING|logging.py:314] 2024-04-22 04:11:15,312 >> Special tokens have been added in the vocabulary, make sure the associated word embeddings are fine-tuned or trained.
04/22/2024 04:11:16 - INFO - llmtuner.model.patcher - Gradient checkpointing enabled.
INFO:llmtuner.model.patcher:Gradient checkpointing enabled.
04/22/2024 04:11:16 - INFO - llmtuner.model.adapter - Fine-tuning method: LoRA
INFO:llmtuner.model.adapter:Fine-tuning method: LoRA
04/22/2024 04:11:16 - INFO - llmtuner.model.utils - Found linear modules: k_proj,o_proj,down_proj,v_proj,up_proj,q_proj,gate_proj
INFO:llmtuner.model.utils:Found linear modules: k_proj,o_proj,down_proj,v_proj,up_proj,q_proj,gate_proj
[WARNING|logging.py:329] 2024-04-22 04:11:16,731 >> Unsloth 2024.4 patched 32 layers with 32 QKV layers, 32 O layers and 32 MLP layers.
04/22/2024 04:11:16 - INFO - llmtuner.model.loader - trainable params: 20971520 || all params: 8051232768 || trainable%: 0.2605
INFO:llmtuner.model.loader:trainable params: 20971520 || all params: 8051232768 || trainable%: 0.2605
[INFO|trainer.py:601] 2024-04-22 04:11:16,796 >> Using auto half precision backend
04/22/2024 04:11:17 - INFO - llmtuner.train.utils - Using LoRA+ optimizer with loraplus lr ratio 16.00.
INFO:llmtuner.train.utils:Using LoRA+ optimizer with loraplus lr ratio 16.00.
[WARNING|logging.py:329] 2024-04-22 04:11:17,203 >> ==((====))== Unsloth - 2x faster free finetuning | Num GPUs = 1
\\ /| Num examples = 1,091 | Num Epochs = 3
O^O/ \_/ \ Batch size per device = 2 | Gradient Accumulation steps = 4
\ / Total batch size = 8 | Total steps = 408
"-____-" Number of trainable parameters = 20,971,520
[408/408 48:57, Epoch 2/3]
Step | Training Loss |
---|---|
10 | 1.568300 |
20 | 1.478600 |
30 | 1.298700 |
40 | 1.188600 |
50 | 1.185700 |
60 | 1.200300 |
70 | 1.249100 |
80 | 1.213600 |
90 | 1.255900 |
100 | 1.186000 |
110 | 1.210600 |
120 | 1.216200 |
130 | 1.111400 |
140 | 1.077700 |
150 | 0.906100 |
160 | 0.895100 |
170 | 0.981500 |
180 | 0.759400 |
190 | 0.834800 |
200 | 0.816900 |
210 | 0.773200 |
220 | 0.946500 |
230 | 0.764600 |
240 | 0.914700 |
250 | 0.864800 |
260 | 0.840600 |
270 | 0.853600 |
280 | 0.745800 |
290 | 0.500800 |
300 | 0.597600 |
310 | 0.616400 |
320 | 0.574100 |
330 | 0.490300 |
340 | 0.602800 |
350 | 0.563700 |
360 | 0.552900 |
370 | 0.574400 |
380 | 0.468200 |
390 | 0.549200 |
400 | 0.528500 |
[INFO|<string>:460] 2024-04-22 05:00:27,815 >>
Training completed. Do not forget to share your model on huggingface.co/models =)
[INFO|trainer.py:3067] 2024-04-22 05:00:27,822 >> Saving model checkpoint to llama3_lora
[INFO|configuration_utils.py:728] 2024-04-22 05:00:28,263 >> loading configuration file config.json from cache at /root/.cache/huggingface/hub/models--unsloth--llama-3-8b-Instruct-bnb-4bit/snapshots/3fe39f383d040c52882f7f9faaf600dc57c01a8d/config.json
[INFO|configuration_utils.py:791] 2024-04-22 05:00:28,266 >> Model config LlamaConfig {
"_name_or_path": "meta-llama/Meta-Llama-3-8B-Instruct",
"architectures": [
"LlamaForCausalLM"
],
"attention_bias": false,
"attention_dropout": 0.0,
"bos_token_id": 128000,
"eos_token_id": 128001,
"hidden_act": "silu",
"hidden_size": 4096,
"initializer_range": 0.02,
"intermediate_size": 14336,
"max_position_embeddings": 8192,
"model_type": "llama",
"num_attention_heads": 32,
"num_hidden_layers": 32,
"num_key_value_heads": 8,
"pretraining_tp": 1,
"quantization_config": {
"_load_in_4bit": true,
"_load_in_8bit": false,
"bnb_4bit_compute_dtype": "bfloat16",
"bnb_4bit_quant_type": "nf4",
"bnb_4bit_use_double_quant": true,
"llm_int8_enable_fp32_cpu_offload": false,
"llm_int8_has_fp16_weight": false,
"llm_int8_skip_modules": null,
"llm_int8_threshold": 6.0,
"load_in_4bit": true,
"load_in_8bit": false,
"quant_method": "bitsandbytes"
},
"rms_norm_eps": 1e-05,
"rope_scaling": null,
"rope_theta": 500000.0,
"tie_word_embeddings": false,
"torch_dtype": "bfloat16",
"transformers_version": "4.38.2",
"use_cache": true,
"vocab_size": 128256
}
[INFO|tokenization_utils_base.py:2459] 2024-04-22 05:00:28,538 >> tokenizer config file saved in llama3_lora/tokenizer_config.json
[INFO|tokenization_utils_base.py:2468] 2024-04-22 05:00:28,541 >> Special tokens file saved in llama3_lora/special_tokens_map.json
[INFO|modelcard.py:450] 2024-04-22 05:00:28,827 >> Dropping the following result as it does not have all the necessary fields:
{'task': {'name': 'Causal Language Modeling', 'type': 'text-generation'}}
***** train metrics *****
epoch = 2.99
total_flos = 32079633GF
train_loss = 0.8929
train_runtime = 0:49:10.61
train_samples_per_second = 1.109
train_steps_per_second = 0.138
推理:
from llmtuner import ChatModel
from llmtuner.extras.misc import torch_gc
%cd /content/LLaMA-Factory/
chat_model = ChatModel(dict(
model_name_or_path=“unsloth/llama-3-8b-Instruct-bnb-4bit”,
adapter_name_or_path=“llama3_lora”,
finetuning_type=“lora”,
template=“llama3”,
))
messages = []
while True:
query = input("\nUser: ")
if query.strip() == “exit”:
torch_gc()
break
if query.strip() == “clear”:
messages = []
torch_gc()
print(“History has been removed.”)
continue
messages.append({“role”: “user”, “content”: query})
print(“Assistant: “, end=””, flush=True)
response = “”
for new_text in chat_model.stream_chat(messages):
print(new_text, end=“”, flush=True)
response += new_text
print()
messages.append({“role”: “assistant”, “content”: response})
推理执行日志
/usr/local/lib/python3.10/dist-packages/huggingface_hub/utils/_token.py:89: UserWarning:
The secret `HF_TOKEN` does not exist in your Colab secrets.
To authenticate with the Hugging Face Hub, create a token in your settings tab (<https://huggingface.co/settings/tokens>), set it as secret in your Google Colab and restart your session.
You will be able to reuse this secret in all of your notebooks.
Please note that authentication is recommended but still optional to access public models or datasets.
warnings.warn(
[INFO|tokenization_utils_base.py:2046] 2024-04-22 05:12:13,951 >> loading file tokenizer.json from cache at /root/.cache/huggingface/hub/models--unsloth--llama-3-8b-Instruct-bnb-4bit/snapshots/3fe39f383d040c52882f7f9faaf600dc57c01a8d/tokenizer.json
[INFO|tokenization_utils_base.py:2046] 2024-04-22 05:12:13,953 >> loading file added_tokens.json from cache at None
[INFO|tokenization_utils_base.py:2046] 2024-04-22 05:12:13,957 >> loading file special_tokens_map.json from cache at /root/.cache/huggingface/hub/models--unsloth--llama-3-8b-Instruct-bnb-4bit/snapshots/3fe39f383d040c52882f7f9faaf600dc57c01a8d/special_tokens_map.json
[INFO|tokenization_utils_base.py:2046] 2024-04-22 05:12:13,959 >> loading file tokenizer_config.json from cache at /root/.cache/huggingface/hub/models--unsloth--llama-3-8b-Instruct-bnb-4bit/snapshots/3fe39f383d040c52882f7f9faaf600dc57c01a8d/tokenizer_config.json
[WARNING|logging.py:314] 2024-04-22 05:12:14,407 >> Special tokens have been added in the vocabulary, make sure the associated word embeddings are fine-tuned or trained.
04/22/2024 05:12:14 - INFO - llmtuner.data.template - Replace eos token: <|eot_id|>
INFO:llmtuner.data.template:Replace eos token: <|eot_id|>
[INFO|configuration_utils.py:728] 2024-04-22 05:12:14,462 >> loading configuration file config.json from cache at /root/.cache/huggingface/hub/models--unsloth--llama-3-8b-Instruct-bnb-4bit/snapshots/3fe39f383d040c52882f7f9faaf600dc57c01a8d/config.json
[INFO|configuration_utils.py:791] 2024-04-22 05:12:14,464 >> Model config LlamaConfig {
"_name_or_path": "unsloth/llama-3-8b-Instruct-bnb-4bit",
"architectures": [
"LlamaForCausalLM"
],
"attention_bias": false,
"attention_dropout": 0.0,
"bos_token_id": 128000,
"eos_token_id": 128001,
"hidden_act": "silu",
"hidden_size": 4096,
"initializer_range": 0.02,
"intermediate_size": 14336,
"max_position_embeddings": 8192,
"model_type": "llama",
"num_attention_heads": 32,
"num_hidden_layers": 32,
"num_key_value_heads": 8,
"pretraining_tp": 1,
"quantization_config": {
"_load_in_4bit": true,
"_load_in_8bit": false,
"bnb_4bit_compute_dtype": "bfloat16",
"bnb_4bit_quant_type": "nf4",
"bnb_4bit_use_double_quant": true,
"llm_int8_enable_fp32_cpu_offload": false,
"llm_int8_has_fp16_weight": false,
"llm_int8_skip_modules": null,
"llm_int8_threshold": 6.0,
"load_in_4bit": true,
"load_in_8bit": false,
"quant_method": "bitsandbytes"
},
"rms_norm_eps": 1e-05,
"rope_scaling": null,
"rope_theta": 500000.0,
"tie_word_embeddings": false,
"torch_dtype": "bfloat16",
"transformers_version": "4.38.2",
"use_cache": true,
"vocab_size": 128256
}
04/22/2024 05:12:14 - INFO - llmtuner.model.patcher - Loading ?-bit BITSANDBYTES-quantized model.
INFO:llmtuner.model.patcher:Loading ?-bit BITSANDBYTES-quantized model.
04/22/2024 05:12:14 - INFO - llmtuner.model.patcher - Using KV cache for faster generation.
INFO:llmtuner.model.patcher:Using KV cache for faster generation.
[INFO|modeling_utils.py:3257] 2024-04-22 05:12:14,509 >> loading weights file model.safetensors from cache at /root/.cache/huggingface/hub/models--unsloth--llama-3-8b-Instruct-bnb-4bit/snapshots/3fe39f383d040c52882f7f9faaf600dc57c01a8d/model.safetensors
[INFO|modeling_utils.py:1400] 2024-04-22 05:12:14,560 >> Instantiating LlamaForCausalLM model under default dtype torch.float16.
[INFO|configuration_utils.py:845] 2024-04-22 05:12:14,569 >> Generate config GenerationConfig {
"bos_token_id": 128000,
"eos_token_id": 128001
}
[INFO|modeling_utils.py:3992] 2024-04-22 05:12:21,290 >> All model checkpoint weights were used when initializing LlamaForCausalLM.
[INFO|modeling_utils.py:4000] 2024-04-22 05:12:21,293 >> All the weights of LlamaForCausalLM were initialized from the model checkpoint at unsloth/llama-3-8b-Instruct-bnb-4bit.
If your task is similar to the task the model of the checkpoint was trained on, you can already use LlamaForCausalLM for predictions without further training.
[INFO|configuration_utils.py:800] 2024-04-22 05:12:21,357 >> loading configuration file generation_config.json from cache at /root/.cache/huggingface/hub/models--unsloth--llama-3-8b-Instruct-bnb-4bit/snapshots/3fe39f383d040c52882f7f9faaf600dc57c01a8d/generation_config.json
[INFO|configuration_utils.py:845] 2024-04-22 05:12:21,359 >> Generate config GenerationConfig {
"bos_token_id": 128000,
"eos_token_id": 128001
}
04/22/2024 05:12:21 - INFO - llmtuner.model.adapter - Fine-tuning method: LoRA
INFO:llmtuner.model.adapter:Fine-tuning method: LoRA
04/22/2024 05:12:22 - INFO - llmtuner.model.adapter - Loaded adapter(s): llama3_lora
INFO:llmtuner.model.adapter:Loaded adapter(s): llama3_lora
04/22/2024 05:12:22 - INFO - llmtuner.model.loader - all params: 8051232768
INFO:llmtuner.model.loader:all params: 8051232768
User: 你是谁
Assistant: 您好,我是 Llama-Chinese,一个由 LLaMA Factory 开发的人工智能助手。我可以根据用户的需求提供相应的回答和帮助。
User: 我的蓝牙耳机坏了,我该去看牙科还是耳鼻喉科?
Assistant: 很抱歉听到您的小配件出了问题。蓝牙耳机的问题与牙科或耳鼻喉科无关。您可以尝试与制造商或当地的维修店联系,进行维修或更换。
User: 谢谢你的帮助
Assistant: 不客气。我是人工智能助手 Llama-Chinese,很高兴能帮到您。
User: exit
如何学习大模型 AI ?
由于新岗位的生产效率,要优于被取代岗位的生产效率,所以实际上整个社会的生产效率是提升的。
但是具体到个人,只能说是:
“最先掌握AI的人,将会比较晚掌握AI的人有竞争优势”。
这句话,放在计算机、互联网、移动互联网的开局时期,都是一样的道理。
我在一线互联网企业工作十余年里,指导过不少同行后辈。帮助很多人得到了学习和成长。
我意识到有很多经验和知识值得分享给大家,也可以通过我们的能力和经验解答大家在人工智能学习中的很多困惑,所以在工作繁忙的情况下还是坚持各种整理和分享。但苦于知识传播途径有限,很多互联网行业朋友无法获得正确的资料得到学习提升,故此将并将重要的AI大模型资料包括AI大模型入门学习思维导图、精品AI大模型学习书籍手册、视频教程、实战学习等录播视频免费分享出来。
这份完整版的大模型 AI 学习资料已经上传CSDN,朋友们如果需要可以微信扫描下方CSDN官方认证二维码免费领取【保证100%免费
】
因篇幅有限,仅展示部分资料,需要点击下方链接即可前往获取
2024最新版CSDN大礼包:《AGI大模型学习资源包》免费分享!
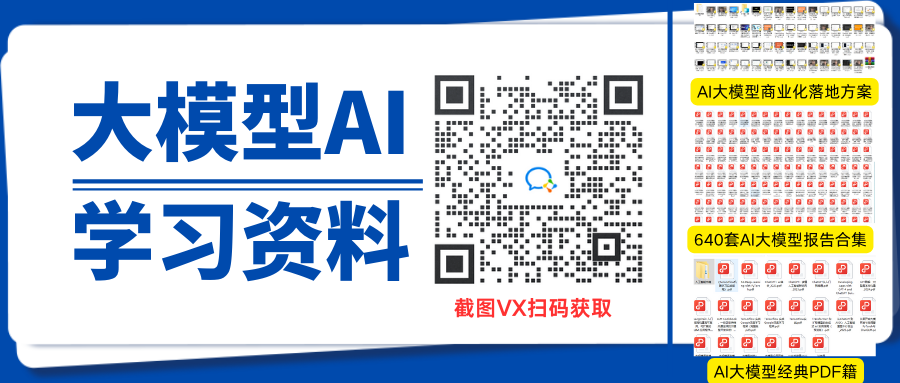
第一阶段(10天):初阶应用
该阶段让大家对大模型 AI有一个最前沿的认识,对大模型 AI 的理解超过 95% 的人,可以在相关讨论时发表高级、不跟风、又接地气的见解,别人只会和 AI 聊天,而你能调教 AI,并能用代码将大模型和业务衔接。
- 大模型 AI 能干什么?
- 大模型是怎样获得「智能」的?
- 用好 AI 的核心心法
- 大模型应用业务架构
- 大模型应用技术架构
- 代码示例:向 GPT-3.5 灌入新知识
- 提示工程的意义和核心思想
- Prompt 典型构成
- 指令调优方法论
- 思维链和思维树
- Prompt 攻击和防范
- …
第二阶段(30天):高阶应用
该阶段我们正式进入大模型 AI 进阶实战学习,学会构造私有知识库,扩展 AI 的能力。快速开发一个完整的基于 agent 对话机器人。掌握功能最强的大模型开发框架,抓住最新的技术进展,适合 Python 和 JavaScript 程序员。
- 为什么要做 RAG
- 搭建一个简单的 ChatPDF
- 检索的基础概念
- 什么是向量表示(Embeddings)
- 向量数据库与向量检索
- 基于向量检索的 RAG
- 搭建 RAG 系统的扩展知识
- 混合检索与 RAG-Fusion 简介
- 向量模型本地部署
- …
第三阶段(30天):模型训练
恭喜你,如果学到这里,你基本可以找到一份大模型 AI相关的工作,自己也能训练 GPT 了!通过微调,训练自己的垂直大模型,能独立训练开源多模态大模型,掌握更多技术方案。
到此为止,大概2个月的时间。你已经成为了一名“AI小子”。那么你还想往下探索吗?
- 为什么要做 RAG
- 什么是模型
- 什么是模型训练
- 求解器 & 损失函数简介
- 小实验2:手写一个简单的神经网络并训练它
- 什么是训练/预训练/微调/轻量化微调
- Transformer结构简介
- 轻量化微调
- 实验数据集的构建
- …
第四阶段(20天):商业闭环
对全球大模型从性能、吞吐量、成本等方面有一定的认知,可以在云端和本地等多种环境下部署大模型,找到适合自己的项目/创业方向,做一名被 AI 武装的产品经理。
- 硬件选型
- 带你了解全球大模型
- 使用国产大模型服务
- 搭建 OpenAI 代理
- 热身:基于阿里云 PAI 部署 Stable Diffusion
- 在本地计算机运行大模型
- 大模型的私有化部署
- 基于 vLLM 部署大模型
- 案例:如何优雅地在阿里云私有部署开源大模型
- 部署一套开源 LLM 项目
- 内容安全
- 互联网信息服务算法备案
- …
学习是一个过程,只要学习就会有挑战。天道酬勤,你越努力,就会成为越优秀的自己。
如果你能在15天内完成所有的任务,那你堪称天才。然而,如果你能完成 60-70% 的内容,你就已经开始具备成为一名大模型 AI 的正确特征了。
这份完整版的大模型 AI 学习资料已经上传CSDN,朋友们如果需要可以微信扫描下方CSDN官方认证二维码免费领取【保证100%免费
】
因篇幅有限,仅展示部分资料,需要点击下方链接即可前往获取
2024最新版CSDN大礼包:《AGI大模型学习资源包》免费分享!
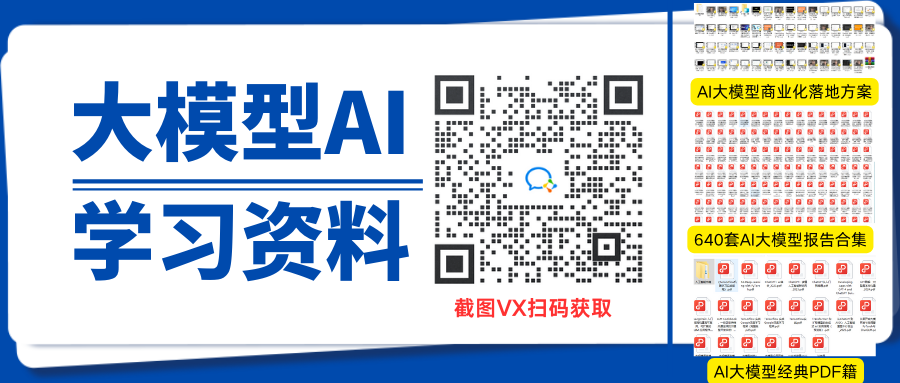