如何使用电力负荷及电对应价数据进行分析和建模_电力负荷及电价数据进行负荷预测和电价预测的仿真研究
以下文字及代码仅供参考。
文章目录
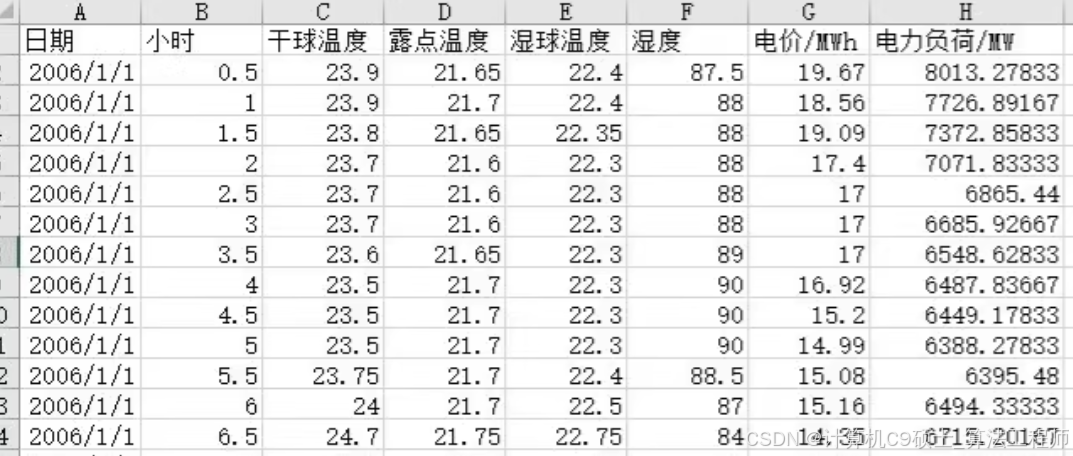
电力负荷及电对应价数据,时间步长为半小时
澳大利亚某地数据包含负荷、电价和各天气因素
来负荷预测、电价预测等仿真研究(2006-2010年真实数据)
应用澳大利亚某地2006-2010年的电力负荷及电价数据进行仿真研究,可以专注于负荷预测和电价预测。以下是如何使用这些数据进行分析和建模的详细步骤,包括数据预处理、特征工程、模型选择与训练等。
数据探索与准备
首先,需要对数据进行初步探索,了解数据结构、缺失值情况以及基本统计信息。假设数据集包含如下列:
- 时间戳(Timestamp)
- 负荷(Load)
- 电价(Price)
- 各种天气因素(如温度Temperature、湿度Humidity等)
1. 加载数据并检查
import pandas as pd
# 假设文件名为'australian_power_data.csv'
data = pd.read_csv('australian_power_data.csv', parse_dates=['Timestamp'])
# 查看数据前几行
print(data.head())
# 检查是否存在缺失值
print(data.isnull().sum())
2. 数据清洗
根据缺失值的情况决定是否删除或填充缺失值。
# 示例:如果缺失值较少,可以选择直接删除
data.dropna(inplace=True)
# 或者使用向前填充方法填补缺失值
# data.fillna(method='ffill', inplace=True)
特征工程
为了提高模型性能,可以从现有数据中提取更多有用的特征。
# 提取时间特征
data['Hour'] = data['Timestamp'].dt.hour
data['DayOfWeek'] = data['Timestamp'].dt.dayofweek
data['Month'] = data['Timestamp'].dt.month
data['Year'] = data['Timestamp'].dt.year
# 可以考虑加入滞后特征
for lag in range(1, 5): # 创建前1到4个时间步长的滞后特征
data[f'Load_lag_{lag}'] = data['Load'].shift(lag)
data[f'Price_lag_{lag}'] = data['Price'].shift(lag)
data.dropna(inplace=True) # 删除由于创建滞后特征而产生的缺失值
模型选择与训练
对于负荷预测和电价预测,可以选择LSTM或者GRU等循环神经网络模型,因为它们擅长处理序列数据。
使用Keras构建LSTM模型示例
from sklearn.model_selection import train_test_split
from tensorflow.keras.models import Sequential
from tensorflow.keras.layers import LSTM, Dense, Dropout
from tensorflow.keras.optimizers import Adam
# 分离特征和目标变量
features = ['Load_lag_1', 'Price_lag_1', 'Temperature', 'Humidity', 'Hour', 'DayOfWeek', 'Month']
X = data[features]
y_load = data['Load']
y_price = data['Price']
# 划分训练集和测试集
X_train, X_test, y_train_load, y_test_load, y_train_price, y_test_price = train_test_split(X, y_load, y_price, test_size=0.2, random_state=42)
# 构建LSTM模型
def build_lstm_model(input_shape):
model = Sequential()
model.add(LSTM(units=50, return_sequences=True, input_shape=input_shape))
model.add(Dropout(0.2))
model.add(LSTM(units=50))
model.add(Dropout(0.2))
model.add(Dense(units=1))
model.compile(optimizer=Adam(), loss='mean_squared_error')
return model
input_shape = (X_train.shape[1], 1)
model_load = build_lstm_model(input_shape)
model_price = build_lstm_model(input_shape)
# 训练模型
history_load = model_load.fit(X_train.values.reshape((X_train.shape[0], X_train.shape[1], 1)), y_train_load, epochs=50, batch_size=32, validation_split=0.2)
history_price = model_price.fit(X_train.values.reshape((X_train.shape[0], X_train.shape[1], 1)), y_train_price, epochs=50, batch_size=32, validation_split=0.2)
模型评估
评估模型在测试集上的表现,通常使用的指标有均方误差(MSE)、均方根误差(RMSE)等。
from sklearn.metrics import mean_squared_error
predictions_load = model_load.predict(X_test.values.reshape((X_test.shape[0], X_test.shape[1], 1)))
predictions_price = model_price.predict(X_test.values.reshape((X_test.shape[0], X_test.shape[1], 1)))
mse_load = mean_squared_error(y_test_load, predictions_load)
mse_price = mean_squared_error(y_test_price, predictions_price)
print(f"Load Prediction MSE: {mse_load}")
print(f"Price Prediction MSE: {mse_price}")
结论
2006-2010年澳大利亚某地的真实电力负荷及电价数据进行仿真研究,实现负荷预测和电价预测。
使用2006-2010年澳大利亚某地的电力负荷及电价数据进行负荷预测和电价预测的仿真研究,遵循以下步骤。从数据准备到模型训练与评估的全过程,旨在帮助你构建有效的预测模型。
1. 数据预处理
首先,确保你的数据集是干净且结构化的,这包括处理缺失值、异常值以及对时间序列数据进行适当的转换(例如将日期时间信息解析为可使用的格式)。
import pandas as pd
# 假设数据文件名为'australian_power_data.csv'
data = pd.read_csv('australian_power_data.csv', parse_dates=['Timestamp'])
# 检查并处理缺失值
print(data.isnull().sum())
data.dropna(inplace=True) # 或者选择填充缺失值的方法
# 设置时间戳作为索引
data.set_index('Timestamp', inplace=True)
2. 特征工程
根据业务需求和领域知识,创建额外的特征来增强模型的表现。比如,可以从时间戳中提取出小时、星期几等信息;也可以考虑加入天气因素如温度、湿度等作为输入特征。
# 提取时间相关的特征
data['Hour'] = data.index.hour
data['DayOfWeek'] = data.index.dayofweek
data['Month'] = data.index.month
# 根据需要添加其他特征,如滞后特征或滚动平均等
3. 构建模型
对于时间序列预测任务,LSTM(长短期记忆网络)和GRU(门控循环单元)是两种常用的深度学习模型。这里以LSTM为例,展示如何使用TensorFlow/Keras构建模型。
使用TensorFlow/Keras构建LSTM模型
from tensorflow.keras.models import Sequential
from tensorflow.keras.layers import LSTM, Dense, Dropout
from sklearn.preprocessing import MinMaxScaler
from sklearn.model_selection import train_test_split
# 数据标准化
scaler = MinMaxScaler(feature_range=(0, 1))
scaled_data = scaler.fit_transform(data)
# 准备训练和测试数据
X, y_load = scaled_data[:, :-1], scaled_data[:, -1] # 假设最后一列是负荷数据
X_train, X_test, y_train_load, y_test_load = train_test_split(X, y_load, test_size=0.2, shuffle=False)
def build_lstm_model(input_shape):
model = Sequential()
model.add(LSTM(units=50, return_sequences=True, input_shape=input_shape))
model.add(Dropout(0.2))
model.add(LSTM(units=50))
model.add(Dropout(0.2))
model.add(Dense(units=1))
model.compile(optimizer='adam', loss='mean_squared_error')
return model
input_shape = (X_train.shape[1], 1)
model = build_lstm_model(input_shape)
4. 训练模型
history = model.fit(X_train.reshape((X_train.shape[0], X_train.shape[1], 1)),
y_train_load,
epochs=50,
batch_size=32,
validation_data=(X_test.reshape((X_test.shape[0], X_test.shape[1], 1)), y_test_load))
5. 模型评估
在测试集上评估模型性能,并计算误差指标如均方误差(MSE)、均方根误差(RMSE)等。
from sklearn.metrics import mean_squared_error
import numpy as np
predictions = model.predict(X_test.reshape((X_test.shape[0], X_test.shape[1], 1)))
predictions_rescaled = scaler.inverse_transform(np.concatenate([X_test, predictions], axis=1))[:, -1]
y_test_rescaled = scaler.inverse_transform(np.concatenate([X_test, y_test_load.reshape(-1, 1)], axis=1))[:, -1]
mse = mean_squared_error(y_test_rescaled, predictions_rescaled)
print(f'Mean Squared Error: {mse}')
6. 电价预测
电价预测的过程类似于负荷预测,只需将目标变量改为电价数据即可。可以根据具体需求调整模型架构和参数。
通过上述步骤,你可以利用给定的数据集进行负荷预测和电价预测的研究。注意,在实际操作过程中可能需要根据实际情况调整数据预处理方法、特征选择、模型架构及参数,以达到最佳预测效果。此外,还可以尝试不同的模型(如GRU、Transformer等)以及集成学习方法来进一步提升预测准确性。