逻辑回归实践
数据准备
(1)生成200条二分类数据(2个特征)
from sklearn.datasets import make_blobs
X, y = make_blobs(n_samples = 200,
n_features = 2,
centers = 2,
random_state = 8)
(2)数据可视化
import matplotlib.pyplot as plt
%matplotlib inline
plt.scatter(X[:, 0], X[:, 1], c = y, cmap = plt.cm.spring, edgecolors = 'k')
plt.scatter(X[:, 0], X[:, 1], c = y)
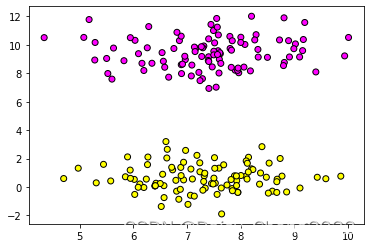
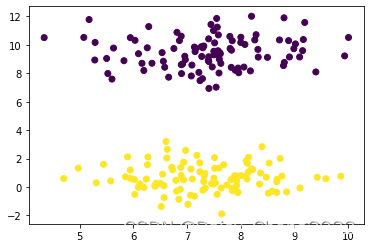
梯度下降法实现逻辑回归(批量,平均,随机)
(1)数据准备
import numpy as np
x_ones = np.ones((X.shape[0], 1))
X = np.hstack((X, x_ones))
from sklearn.model_selection import train_test_split
X_train, X_test, y_train, y_test = train_test_split(X, y, test_size = 0.3, random_state = 8)
(2)查看数据维度
print(X.shape, X_train.shape, X_test.shape)
print(y.shape, y_train.shape, y_test.shape)
(3)将因变量转为列向量
y_train = y_train.reshape(-1, 1)
y_test = y_test.reshape(-1, 1)
print(y_train.shape, y_test.shape)
(4)定义sigmoid函数
theta = np.ones([X_train.shape[1], 1])
alpha = 0.001
def sigmoid(z):
s = 1.0 / (1 + np.exp(-z))
return s
(5)预测
num_iters = 10000
m = 140
for i in range(num_iters):
h = sigmoid(np.dot(X_train, theta))
theta = theta - alpha * np.dot(X_train.T, (h - y_train)) / m
print(theta)
pred_y = sigmoid(np.dot(X_test, theta))
pred_y[pred_y > 0.5] = 1
pred_y[pred_y <= 0.5] = 0
print(pred_y.reshape(1, -1))
print(y_test.reshape(1, -1))
print('预测准确率为:', np.sum(pred_y == y_test) / len(y_test))
kaggle糖尿病预测实战
(1)数据准备
import pandas as pd
data = pd.read_csv('pima-indians-diabetes.data.csv')
print(data)
X = data.iloc[:, :-1]
y = data.iloc[:, -1]
mu = X.mean(axis = 0)
std = X.std(axis = 0)
X = (X - mu) / std
x_ones = np.ones((X.shape[0], 1))
X = np.hstack((X, x_ones))
X_train, X_test, y_train, y_test = train_test_split(X, y, test_size = 0.3, random_state = 8)
y_train = y_train.values.reshape(-1, 1)
y_test = y_test.values.reshape(-1, 1)
print(y_train.shape, y_test.shape)
(2)定义sigmoid函数
theta = np.ones([X_train.shape[1], 1])
alpha = 0.001
def sigmoid(z):
s = 1.0 / (1 + np.exp(-z))
return s
(3)预测
num_iters = 10000
m = 537
for i in range(num_iters):
h = sigmoid(np.dot(X_train, theta))
theta = theta - alpha * np.dot(X_train.T, (h - y_train)) / m
print(theta)
pred_y = sigmoid(np.dot(X_test, theta))
pred_y[pred_y > 0.5] = 1
pred_y[pred_y <= 0.5] = 0
print(pred_y.reshape(1, -1))
print(y_test.reshape(1, -1))
print('预测准确率为:', np.sum(pred_y == y_test) / len(y_test))
sklearn实现逻辑回归
逻辑回归实现三分类
(1)数据准备
from sklearn.datasets import load_iris
iris = load_iris()
X = iris.data
y = iris.target
from sklearn.model_selection import train_test_split
X_train, X_test, y_train, y_test = train_test_split(X, y, random_state = 8)
(2)导入逻辑回归模块训练
from sklearn.linear_model import LogisticRegression
logis = LogisticRegression()
logis.fit(X_train, y_train)
logis.get_params()
(3)模型评估
print(logis.score(X_test, y_test))
from sklearn.metrics import classification_report
print(classification_report(y_test, logis.predict(X_test)))
(4)更换参数查看模型效果
logis2 = LogisticRegression(multi_class='multinomial', solver='lbfgs')
logis2.fit(X_train, y_train)
print(logis2.score(X_test, y_test))
logis3 = LogisticRegression(multi_class='ovr', solver='lbfgs')
logis3.fit(X_train, y_train)
logis3.score(X_test, y_test)