该系列仅在原课程基础上部分知识点添加个人学习笔记,或相关推导补充等。如有错误,还请批评指教。在学习了 Andrew Ng 课程的基础上,为了更方便的查阅复习,将其整理成文字。因本人一直在学习英语,所以该系列以英文为主,同时也建议读者以英文为主,中文辅助,以便后期进阶时,为学习相关领域的学术论文做铺垫。- ZJ
转载请注明作者和出处:ZJ 微信公众号-「SelfImprovementLab」
知乎:https://zhuanlan.zhihu.com/c_147249273
CSDN:http://blog.csdn.net/junjun_zhao/article/details/79148233
1.6 Size of dev and test sets (开发集合测试集的大小)
(字幕来源:网易云课堂)
In the last video,you saw how your dev and test sets should come from the same distribution,but how large should they be?The guidelines to help set up your dev and test sets are changing in the Deep Learning era.Let’s take a look at some best practices.You might have heard of the rule of thumbin machine learning of taking all the data you have and using a 70/30 split into a train and test set,or if you had to set up train dev and test sets maybe,you would use a 60% training and 20% dev and 20% tests.In earlier eras of machine learning, this was pretty reasonable,especially back when data set sizes were just smaller.So if you had a hundred examples in total,these 70/30 or 60/20/20 rule of thumb would be pretty reasonable.If you had thousand examples,maybe if you had ten thousand examples,these heuristic are not unreasonable.
在上一个视频中,你们知道了你的开发集和测试集为什么必须来自同一分布,但它们规模应该多大?在深度学习时代 设立开发集和测试集的方针,也在变化,我们来看看一些最佳做法,你可能听说过一条经验法则,在机器学习中 把你取得的全部数据,用 70/30 比例分成训练集和测试集,或者如果你必须设立训练集 开发集和测试集,你会这么分 60%训练集 20%开发集 20% 测试集,在机器学习的早期 这样分是相当合理的,特别是以前的数据集大小要小得多,所以如果你总共有 100 个例子,这样 70/30 或者 60/20/20 分的经验法则是相当合理的,如果你有几千个例子,或者有一万个例子,这些做法也还是合理的。
But in the modern machine learning era,we are now used to working with much larger data set sizes.So let’s say you have a million training examples,it might be quite reasonable to set up your data so that you have 98% in the training set,1% dev, and 1% test.And when you use D and T to abbreviate dev and test sets.Because if you have a million examples,then 1% of that,is 10,000 examples, and that might be plenty enough for a dev set or for a test set.So, in the modern Deep Learning era where sometimes we have much larger data sets,It’s quite reasonable to use a much smaller than 20or 30% of your data for a dev set or a test set.And because Deep Learning algorithms have such a huge hunger for data, I’m seeing that,the problems we have large data sets that havemuch larger fraction of it goes into the training set.
但在现代机器学习中,我们更习惯操作规模大得多的数据集,比如说你有 1 百万个训练例子,这样分可能更合理,98%作为训练集,1%开发集 1%测试集,我们用 D 和 T 缩写来表示开发集和测试集,因为如果你有1百万个例子,那么1%就是,10,000个例子 这对于开发集和测试集来说可能已经够了,所以在现代深度学习时代 有时我们拥有大得多的数据集,所以使用小于20%的比例,或者小于30%比例的数据作为开发集和测试集也是合理的,而且因为深度学习算法对数据的胃口很大 我们可以看到,那些有海量数据集的问题,有更高比例的数据划分到训练集里。
So, how about the test set?Remember the purpose of your test set is that,after you finish developing a system,the test set helps evaluate how good your final system is.The guideline is, to set your test set to big enough to give high confidence in the overall performance of your system.So, unless you need to havea very accurate measure of how well your final system is performing,maybe you don’t need millions and millions of examples in a test set,and maybe for your application if you think that having 10,000 examples gives you enough confidence to find the performance or maybe 100,000 or whatever it is, that might be enough.And this could be much less than,say 30% of the overall data set,depend on how much data you have.
那么测试集呢? 要记住 测试集的目的是,完成系统开发之后,测试集可以帮你评估投产系统的性能,方针就是令你的测试集足够大,能够以高置信度评估系统整体性能。所以除非你需要,对最终投产系统有一个很精确的指标,一般来说 测试集不需要上百万个例子,对于你的应用程序 也许你想,有10,000个例子就能给你足够的置信度来给出性能指标了,也许100,000个 之类的 可能就够了,这数目可能远远小于,比如说整体数据集的30%,取决于你有多少数据。
For some applications,maybe you don’t need a high confidence in the overall performance of your final system.Maybe all you need is a train and dev set,And I think, not having a test set might be okay.In fact, what sometimes happened was,people were talking about using train test splits but what they were actually doing was iterating on the test set.So rather than test set,what they had was a train dev split and no test set.If you’re actually tuning to this set,to this dev set and this test set,It’s better to call the dev set.Although I think in the history of machine learning,not everyone has been completely clean and completely records of about calling the dev set when it really should be treated as test set.But, if all you care about is having some data that you train on,and having some data to tune to,and you’re just going to ship the final system regardless and not worry too much about how it was actually doing,I think it will be healthy and just call the train dev set and acknowledge that you have no test set.This a bit unusual?I’m definitely not recommending not having a test set when building a system.I do find it reassuring to have a separate test set you can use to get an unbiased estimate of how well the performance should be,but if you have a very large dev setso that you think you won’t overfit the dev set too badly.Maybe it’s not totally unreasonable to just have a train dev set,although it’s not what I usually recommend.
对于某些应用,你也许不需要对系统性能有置信度很高的评估,也许你只需要训练集和开发集,我认为 不单独分出一个测试集也是可以的,事实上 有时在实践中,有些人会只分成,训练集和测试集 他们实际上在测试集上迭代,所以这里没有测试集,他们有的是训练集和开发集 但没有测试集,如果你真的在调试这个集,这个开发集和这个测试集,这最好称为开发集,不过在机器学习的历史里,不是每个人都把术语定义分得很清的,有时人们说的开发集 其实应该看做测试集,但如果你只要有数据去训练,有数据去调试就够了,你打算不管测试集 直接部署最终系统,所以不用太担心它的实际表现,我觉得这也是很好的 就将它们称为训练集开发集就好,然后说清楚你没有测试集,这是不是有点不正常?我绝对不建议在搭建系统时省略测试集,因为有个单独的测试集比较令我安心,因为你可以使用这组不带偏差的数据 来测量系统的性能,但如果你的开发集非常大,这样你就不会对开发集过拟合得太厉害。这种情况 只有训练集和测试集也不是完全不合理的,不过我一般不建议这么做。
So to summarize, in the era of big data,I think the old rule of thumb of a 70/30 split,that no longer applies.And the trend has been to use more data for training and less for dev and test,especially when you have a very large data sets.And the rule of thumb is really to try to set the dev set to big enough for its purpose,which helps you evaluate different ideas and pick this up, A or B better.And the purpose of test set is to help you evaluate your final cost biasYou just have to set your test set big enough for that purpose,and that could be much less than 30% of the data.
So, I hope that gives some guidance or some suggestions on how to set up your dev and test sets in the Deep Learning era.Next, it turns out that sometimes,part way through a machine learning problem,you might want to change your evaluation metric,or change your dev and test sets.Let’s talk about it when you might want to do that.
总结一下 在大数据时代,旧的经验规则 这个70/30分,不再适用了,现在流行的是 把大量数据分到训练集 然后少量数据分到开发集和测试集,特别是当你有一个非常大的数据集时,以前的经验法则其实是为了确保开发集足够大 能够达到它的目的,就是帮你评估不同的想法 然后选出 A 还是 B 更好,测试集的目的是评估你最终的成本偏差,你只需要设立足够大的测试集 可以用来这么评估就行了,可能只需要远远小于总体数据量的 30%,所以我希望本视频能给你们一点指导和建议,让你们知道如何在深度学习时代设立开发和测试集,接下来 有时候,在研究机器学习的问题途中,你可能需要更改评估指标,或者改动你的开发集和测试集,我们会讲什么时候需要这样做。
重点总结:
开发集合测试集的大小
- 98 % Training set. 1% Dev set . 1% Test set.
- 测试集帮助评估高置信度评估系统整体性能,若有该要求,取整理数据中的少于 30 %即可。
- 开发集需要足够大去评估不同的 ideas
参考文献:
[1]. 大树先生.吴恩达Coursera深度学习课程 DeepLearning.ai 提炼笔记(3-1)– 机器学习策略(1)
PS: 欢迎扫码关注公众号:「SelfImprovementLab」!专注「深度学习」,「机器学习」,「人工智能」。以及 「早起」,「阅读」,「运动」,「英语 」「其他」不定期建群 打卡互助活动。
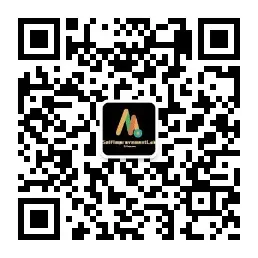