Content
Machine Learning 分类
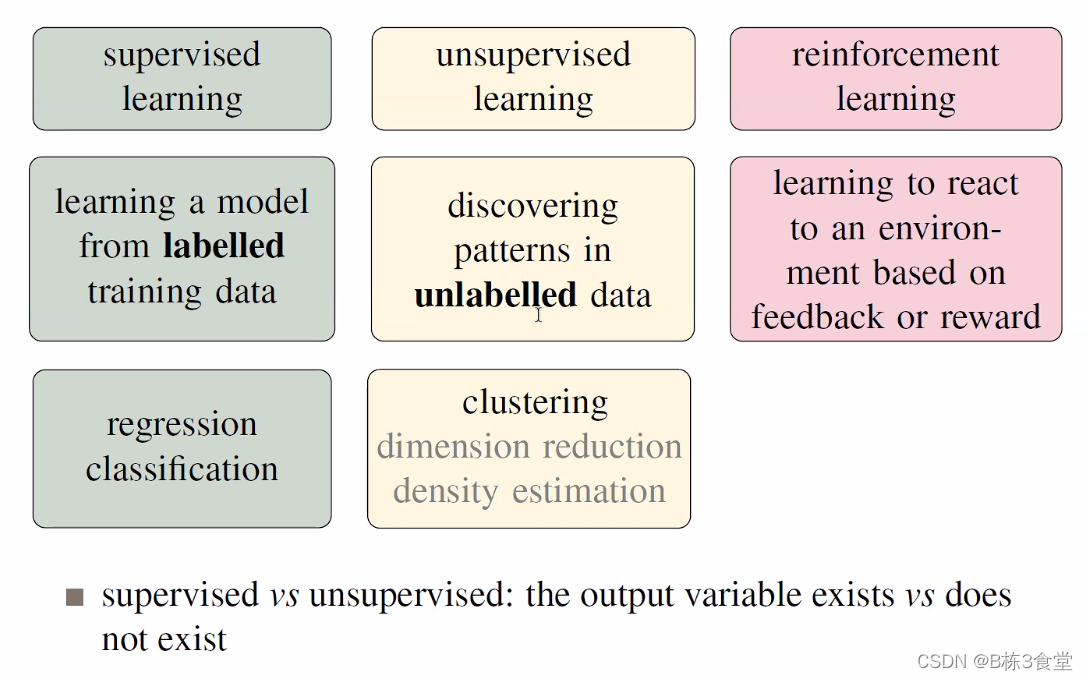

1 PCA
Consider linear combinations:
Y 1 = a 11 X 1 + a 12 X 2 + . . . + a 1 p X p = a 1 T X Y 2 = a 21 X 1 + a 22 X 2 + . . . + a 2 p X p = a 2 T X . . . Y 3 = a p 1 X 1 + a p 2 X 2 + . . . + a p p X p = a p T X Y_1=a_{11}X_1+a_{12}X_2+...+a_{1p}X_p=\mathrm{a_1}^\mathsf{T}X\\Y_2=a_{21}X_1+a_{22}X_2+...+a_{2p}X_p=\mathrm{a_2}^\mathsf{T}X\\.\\.\\.\\Y_3=a_{p1}X_1+a_{p2}X_2+...+a_{pp}X_p=\mathrm{a_p}^\mathsf{T}X Y1=a11X1+a12X2+...+a1pXp=a1TXY2=a21X1