身体是革命的本钱
As a national sport, the benefits oftable tennis courses in universities are very significant, especially for strengtheningthe physical health of college students. And many college students have astrong interest in sports and leisure time. Therefore, many universitiesregularly carry out table tennis teaching and training, and intelligentlyevaluate the teaching effect with visual recognition technology. Yolo V4 network and mobilenet-v2 lightweightnetwork have better performance in table tennis teaching and training. In thispaper, the students body detection performance of Yolo V4 network in tabletennis teaching and training is analyzed in detail. Further combining with Mobilenet-v2 lightweight network, thetraining posture can be well detected according to the physiologicalcharacteristics of human bones. For table tennis teachingand training, Yolo V4 and Mobilenet-v2 networks can intelligently evaluatestudents' learning effect, which has afar-reaching positive effect on teaching and health.
侧重点在于台球、 国民健康、Yolo网络、Mobilenet-v2等网络介绍。
Table tennis is a part of collegesports. Universities offer different kinds of table tennis courses, and theteaching methods and means of table tennis are various. Table tennis has abroad mass base. It has distinct technical characteristics and low requirementson the ground.The main purpose of tabletennis teaching in universities is not to cultivate reserve talents ofcompetitive sports. It is to cultivate students' interest in practicing tabletennis and improve students' interest in table tennis. Popularize thedevelopment of table tennis and enhance the students' physique. The statepromulgates policies to promote the high-quality development of sports.Encourage college sports to increase the diversity of physical educationcurriculum, and maintain the leading level of Chinese table tennis in the worldwith competitive sports.It is influenced by the promotion ofphysical education reform, the development of national fitness movement and theexcellent performance of table tennis Olympic champion. Many universities haveopened table tennis compulsory courses, elective courses and special courses,and the number of courses has been increased simultaneously. It can effectivelypromote the learning effect of students' table tennis, and promote theimprovement of students' physical quality while learning the special skills oftable tennis. In such a good atmosphere, table tennis is used as the projectguide to attract more people to practice table tennis and promote the spread oftable tennis culture. And can enhance the physique of college students andpromote health.
The physical health of collegestudents is a global public health problem. For the college students whosephysical strength, energy and vitality are in the golden age. However, they often neglect their own physicalhealth problems and exercise to strengthen their physique. Insufficient physicalactivity is a key factor affecting the healthy life of university students. TheWorld Health Organization has pointed out that insufficient physical activityis the main risk factor leading to global non communicable diseases and deaths. There is no doubt that regular physical activity is beneficial to thehealth of adults. The strategy of promoting physical exercise has become apublic health method to enhance physical fitness and promote health. Theproportion of college students aged 18-24 who reached the recommended length ofphysical education decreased rapidly. Data from many countries across thecountry show that sedentary, video games and exclusion of sports activities allmake college students unable to meet the physical exercise guidelines. As anational fitness sport, table tennis is easy to establish multiple associationsand organize community activities in the university to improve the health ofcollege students and help them develop physically and mentally.The teaching and training of collegetable tennis are facing many severe teaching tasks and challenges. Trainingmode, guiding method and sports innovation have become the core of collegetable tennis teaching. Intelligent equipment and technology provide more teachingmethods and advanced equipment for table tennis training. For example,assistive devices, robots, face recognition and motion recognition areintegrated with table tennis training . Universities have tried tointroduce artificial intelligence equipment for physical education teaching.Considering that there are a large number of students in college physicaleducation teaching. This requires artificial intelligence equipment to providemore convenient, easy to operate and accurate human-computer interactionequipment. Some universities have established member information databases fortheir college students to manage and record the data of College Students'physical training, personal identity information and face recognition features. The needs of face recognition, motionrecognition and target detection in table tennis training need to be solved bycombining computer vision technology. Neural network is the core algorithm ofcomputer vision. In the past two years, a large number of patents or researchresults have emerged, which use computer vision to compare, correct andreconstruct the movements in table tennis. To promote scientific trainingmethods and improve training efficiency.
高校乒乓球教学现状:
For the teaching of table tennis inuniversities, the principle of "health first and lifelong sports" isalways adopted to improve the training level of teachers and stimulatestudents' interest. So as to promote the health of college students and improvetheir level of table tennis. However, many universities also have problems suchas lack of equipment, limited level of teachers and backward training concepts.Flipped classroom enters the classroomwith a new table tennis teaching mode. With the help of the network teachingplatform, it can mobilize students' learning motivation and make teachers'guidance and training more targeted. Artificial intelligence has also enteredthe University table tennis sports class. It uses mobile phones to remotelycontrol the Pangbot Robot, and adjusts the tabletennis assistant training robot according to its own table tennis level. It canmeet the needs of people at different levels . Although table tennis iswidely carried out in universities under the promotion of national policies, thereis no comprehensive and accurate skill evaluation index. Therefore, Li proposed the table tennis skill level standardin order to improve the control ability, and promote students to master tabletennis skills. In the University table tennis class, the skill level intervention wascarried out. The teaching confirmed that under the level index intervention,the forehand and backhand drop point control ability was significantlyenhanced, and the backhand technique was mastered faster. It promotes theenthusiasm of learning and the effect of classroom practice is obviouslyoptimized.Robot playing table tennis is theapplication of computer vision in table tennis teaching and training. ZhejiangUniversity has carried out the research project of robot playing table tennis,manufacturing the first generation of table tennis robot based on monocularvision system, and achieved 60% success rate. Then, the second generationof table tennis fighting robot with horizontal guide rail was also born. The newimitation robot can even complete 145 rounds of continuous human-machine tabletennis and 144 rounds of continuous machine to machine table tennis. In theprocess of table tennis, the opponent's stroke can often help improve theprediction accuracy of the return ball. The exploration of table tennis robotshas achieved fruitful results. The traditional table tennis robot vision systemmainly focuses on target positioning and hitting prediction, and lacks therelated research on the batter and his gestures.
Human perception of the objective world is mainly through thevisual system. The visual system collects image information through the retina,and the visible part of the objective object is projected into the retina. Thebrain performs three-dimensional data processing through two-dimensionalimaging in the retina in a short time. The shape, size, spatial relativedistance, color texture and motion characteristics of the observed object areanalyzed.Visual recognition is to imitate thevisual characteristics of the human era, use the camera to collect images, anduse the computer to do data analysis to achieve the same function as the humaneye. In table tennis teaching and training, visual recognition can be dividedinto monocular, binocular and multi ocular visual systems.
计算机视觉与乒乓球:
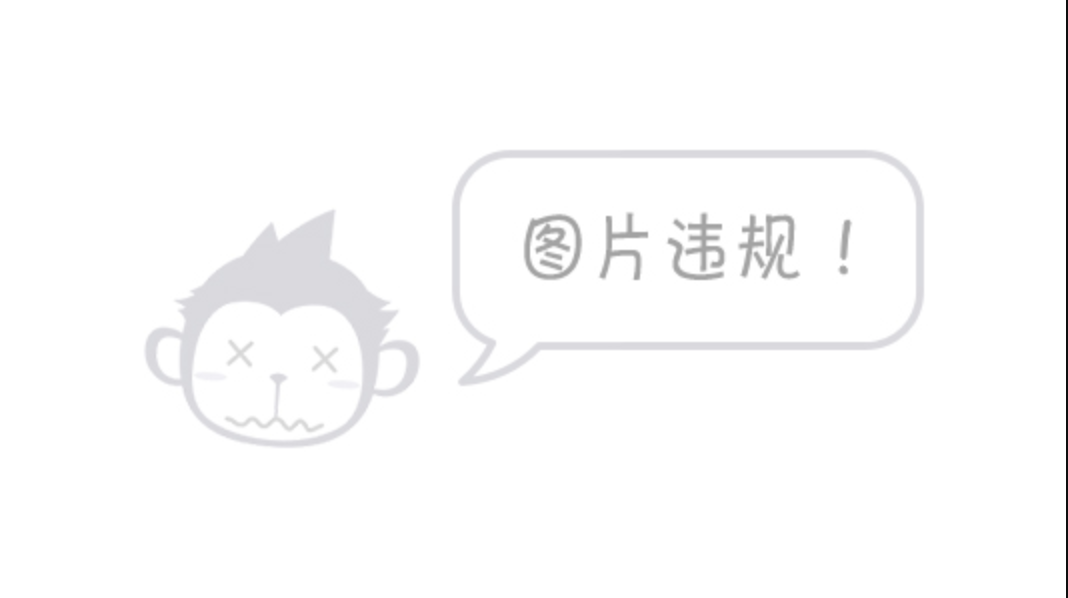
In college table tennistraining, because the students are mobile, the movement posture recognition ismore critical. Johansson found in the 1970s that most of the movements in lifecan be described by the movement of key nodes of the human body. Byconnecting the key nodes of the human body according to certain rules, suchactions as walking, running or jumping can be defined.Human posture recognitionmainly relied on the establishment of skeleton model to reconstruct humanposture. The most representative is that the Research Institute of TsinghuaUniversity proposed a new type of joint model of human upper body based on the3D model in 2002 to restore the 3D posture of human upper body. The end pointof the joint is fixed in the model, and the actual posture of the human body isobtained by solving the model.In 2012, convolutionalneural networks showed surprising results in the image processing competition, and researchers began to pay attention to applying deep learning methodsto human posture recognition. The essence of human pose recognition based ondeep neural network is to use a large amount of data to construct convolutionlayer and extract effective feature information. Toshev et al. Proposed thatdeep pose is the first deep learning to predict the two-dimensional keypoint coordinates of the human body. In the initial stage, the approximateposition of the attitude key point is output, and then the accurate coordinatesare optimized through multiple stage iterations. The appearance of mpii dataset has brought infinite hope to the research of human posturerecognition. This data set has about 25000 images. The depth network CPM [18]designed by Wei et al. Obtains receptive fields of different sizes throughmultiple convolution operations on the input image, and identifies humanposture by combining the semantic information of the image context. CPM alsointroduces the idea of relay supervision, taking into account the losses ineach intermediate stage of the model, effectively resolves the contradictionbetween network depth and gradient disappearance, improves the accuracy ofattitude recognition, and lays a foundation for subsequent related research.
In terms of human targetdetection and recognition based on vision, Yolo, as a mainstream network model,has achieved good human recognition results in many projects. Deep pose model,open pose model and Mobilenet-v2 lightweight network are commonly used forhuman posture recognition
Yolo v4和动作识别的结合:
The essence of human body detection is the targetdetection task in machine vision. The target detection model inputs pictures orvideo frames, which are input to the detection system for feature extractionafter image preprocessing. The quality of feature extraction largely determinesthe detection effect of the model. The target detection method needs tomanually select specific features according to experience and rules, and thentrain the template matching classifier with these preset features.
Human bodydetection and posture recognition need to complete the processing task ofvision system in the shortest possible time. This requires the human bodydetection model to be as fast as possible, and it is expected to achievereal-time interaction between the batter and the table tennis robot. YOLO V4algorithmdetects table tennis players, which has high detection speed while meeting thedetection accuracy, and locates the position and probability of the players inthe picture or video。 The human body detection of athletes is actually the detection of imagesframe by frame in the loaded video stream. Yolo v4 first reads theinitialization data, scales the size of the read picture, then reads thecategory data, and finally outputs the target category probability and positioncoordinates . Yolo v4network structure can be divided into two main parts.First, read all initialization data, and then perform location prediction andcategory regression.
Before calculation, Yolo v4 will firstread in the model data, that is, the parameters used for calculation, whichwill be used for subsequent calculation. Read in the a priori box data,according to the dimensions of all dimension boxes in the data set. Then, readin the category data, which needs to be consistent with the category of thedata set. If there are 10 categories of data in the dataset, the category datais also 10 categories. Finally, read in the picture size accepted by Yolo v4 algorithm. The algorithm first fills the readimage data with gray blocks according to the size allowed by Yolo v4, and thensends the image containing the target to the backbone feature extractionnetwork to extract features. Three groups of data are generated by down-sampling,and the three groups of data are decoded to output position prediction andcategory information. After the completion of human body detection, it willfurther identify the motion posture of the human body, so as to identify theposture of the table tennis trainer in the images and videos as accurately aspossible. The core is to locate the key points of the posture. Based ondifferent application scenarios, there are also certain differences in themotion posture of table tennis players output by the posture recognition model 。
Mobilenet-v2与手势识别的结合现状:
The general posture model is described based on the humanskeleton structure in physiology, as shown in Figure 5. If the human posturemodel is not defined, the detected key points are like scattered components,and the researchers cannot analyze what kind of posture these key pointsrepresent. Mobilenet-v2lightweight network can be well used as the backbone network for key pointdetection. The neural network adopts the characteristic pyramid network withhourglass model, and uses the deep separation convolution to reduce the amountof computation. Mobilenet-v2, as the backbone network of gesturerecognition in table tennis sports scene, can reduce the parameter scale andcalculation amount of the network and greatly reduce the network learning time.
Inthe table tennis player posture recognition algorithm, PCK and OKs can be usedas general measurement indicators to evaluate the effectiveness of posturerecognition. ThePCK index is used to measure the proportion of key points correctly estimated. If the normalized distance between the detected key point and itscorresponding real key point is less than the set threshold, the key point isconsidered to be correctly estimated. OKs index is also called key pointsimilarity, which is used to measure the similarity between the predictionresults of each key point and the real annotation.
其中,OKs is the mainstream evaluation index used in the current human posturekey point detection model.
可以继续学习的方向:
How to use Yolo v4 network and Mobilenet-v2to complete human body detection and motion gesture recognition is analyzed indetail. The future work plan will focus on the application of computer visionin sports, and try to optimize the most advanced neural network model toachieve more practical needs in sports.
以上参考文献有:
Johnson, S., & Everingham, M. (2010,August). Clustered Pose and Nonlinear Appearance Models for Human PoseEstimation. In bmvc (Vol. 2, No. 4, p. 5).
Krizhevsky, A., Sutskever, I., & Hinton, G.E. (2012). Imagenet classification with deep convolutional neural networks. Advancesin neural information processing systems, 25.
Toshev, A., & Szegedy, C. (2014). Deeppose:Human pose estimation via deep neural networks. In Proceedings of theIEEE conference on computer vision and pattern recognition (pp.1653-1660).
Yao, Y., Mohan, A. A., Bliss-Moreau, E.,Coleman, K., Freeman, S. M., Machado, C. J., ... & Park, H. S. (2021).Openmonkeychallenge: Dataset and benchmark challenges for pose tracking ofnon-human primates. bioRxiv.
Badiola-Bengoa, A., & Mendez-Zorrilla, A.(2021). A Systematic Review of the Application of Camera-Based Human PoseEstimation in the Field of Sport and Physical Exercise. Sensors, 21(18),5996.
Fu, W., Yan, S., Zong, Q., Anderson-Luxford,D., Song, X., Lv, Z., & Lv, C. (2021). Mental health of college studentsduring the COVID-19 epidemic in China. Journal of Affective Disorders, 280,7-10.
Yang, J., Tang, L., & Li, X. W. (2021).Research on Face Recognition Sports Intelligence Training Platform Based onArtificial Intelligence. International Journal on ArtificialIntelligence Tools, 30(06n08), 2140015.
Li, Y. Research on the Quality EvaluationSystem of Table Tennis Special Classroom Teaching For Sports Majors in Collegesand Universities.