In the book, example 5.5 shows the infinity variance of the ordinary importance sampling in a specific case. I tried this experiment in my computer and get a similar result. Unfortunately, my computer’s memory size is not enough to support 100,000,000 episodes for ten runs. So, here I shows the code and the result of 1000,000 episodes for ten runs. Additionally, I tried weighted importance sampling, it converges very fast. I show the result here also.
#==================================================================
# Python3
# Copyright
# 2019 Ye Xiang (xiang_ye@outlook.com)
#==================================================================
import numpy as np
import matplotlib.pyplot as mplt_pyplt
from progressbar import ProgressBar
import threading
STATE_TRANSITION = 0
STATE_TERMINAL = 1
ACTION_LEFT = 1
ACTION_RIGHT = 0
class TrajectoryElement:
def __init__(self, state = STATE_TRANSITION, action = ACTION_LEFT, reward = 1):
self.state = state
self.action = action
self.reward = reward
class PolicyTester():
def __init__(self):
self.__sampling_data = []
def __generate_trajectory(self):
trajectory = []
state = STATE_TRANSITION
action = np.random.choice([ACTION_LEFT, ACTION_RIGHT])
reward = 0
trajectory.append(TrajectoryElement(state, action, reward))
if action == ACTION_RIGHT:
state = STATE_TERMINAL
reward = 0
else:
state = np.random.choice([STATE_TRANSITION, STATE_TERMINAL], p = [0.9, 0.1])
if state == STATE_TERMINAL:
reward = 1
while state != STATE_TERMINAL:
trajectory.append(TrajectoryElement(state, action, reward))
action = np.random.choice([ACTION_LEFT, ACTION_RIGHT])
if action == ACTION_RIGHT:
state = STATE_TERMINAL
reward = 0
else:
state = np.random.choice([STATE_TRANSITION, STATE_TERMINAL], p = [0.9, 0.1])
if state == STATE_TERMINAL:
reward = 1
trajectory.append(TrajectoryElement(state, action, reward))
return trajectory
def __calculate_rho(self, trajectory):
b = 1.0
pai = 1.0
for trj in trajectory:
b *= 0.5
if trj.action == ACTION_RIGHT:
pai = 0
break
return pai / b
def MC_off_policy_first_visit_ordinary(self, episodes):
self.__sampling_data = []
pb = ProgressBar().start()
nominator_sum = 0.0
for i in range(0, episodes):
trajectory = self.__generate_trajectory()
rho = self.__calculate_rho(trajectory)
nominator_sum += rho * trajectory[-1].reward
self.__sampling_data.append(nominator_sum / (i + 1))
pb.update(int(i /episodes * 100))
pb.update(100)
def MC_off_policy_first_visit_weighted(self, episodes):
self.__sampling_data = []
pb = ProgressBar().start()
nominator_sum = 0.0
denominator_sum = 0.0
for i in range(0, episodes):
trajectory = self.__generate_trajectory()
rho = self.__calculate_rho(trajectory)
nominator_sum += rho * trajectory[-1].reward
denominator_sum += rho
Vs = nominator_sum / denominator_sum if denominator_sum != 0.0 else 0.0
self.__sampling_data.append(Vs)
pb.update(int(i /episodes * 100))
pb.update(100)
@property
def sampling_data(self):
return self.__sampling_data
class TestThread_ordinary(threading.Thread):
def __init__(self, test_instance = None, episodes = 10000):
threading.Thread.__init__(self)
self.__test_instance = test_instance
self.__episodes = episodes
def run(self):
if self.__test_instance == None:
return
self.__test_instance.MC_off_policy_first_visit_ordinary(self.__episodes)
def plot_ordinary(episodes, run_times):
threads = []
policy_testers = []
for i in range(0, run_times):
policy_tester = PolicyTester()
thread = TestThread_ordinary(policy_tester, episodes)
threads.append(thread)
policy_testers.append(policy_tester)
thread.start()
for t in threads:
t.join()
for pt in policy_testers:
mplt_pyplt.plot(pt.sampling_data)
mplt_pyplt.xscale('log')
mplt_pyplt.ylim(0, 3)
mplt_pyplt.xlabel('Episodes (log scale)')
mplt_pyplt.ylabel('Monte-Carlo estimate of ' + r'$v_\pi(s)$' + 'with ordinary importance sampling')
mplt_pyplt.show()
def plot_weighted(episodes, run_times):
for i in range(0, run_times):
policy_tester = PolicyTester()
policy_tester.MC_off_policy_first_visit_weighted(episodes)
mplt_pyplt.plot(policy_tester.sampling_data)
mplt_pyplt.xscale('log')
mplt_pyplt.ylim(0, 2)
mplt_pyplt.xlabel('Episodes (log scale)')
mplt_pyplt.ylabel('Monte-Carlo estimate of ' + r'$v_\pi(s)$' + 'with weighted importance sampling')
mplt_pyplt.show()
def set_priority(pid=None,priority=1):
""" Set The Priority of a Windows Process. Priority is a value between 0-5 where
2 is normal priority. Default sets the priority of the current
python process but can take any valid process ID. """
import win32api,win32process,win32con
priorityclasses = [win32process.IDLE_PRIORITY_CLASS,
win32process.BELOW_NORMAL_PRIORITY_CLASS,
win32process.NORMAL_PRIORITY_CLASS,
win32process.ABOVE_NORMAL_PRIORITY_CLASS,
win32process.HIGH_PRIORITY_CLASS,
win32process.REALTIME_PRIORITY_CLASS]
if pid == None:
pid = win32api.GetCurrentProcessId()
handle = win32api.OpenProcess(win32con.PROCESS_ALL_ACCESS, True, pid)
win32process.SetPriorityClass(handle, priorityclasses[priority])
if __name__ == '__main__':
set_priority(priority = 4)
plot_ordinary(1000000, 10)
plot_weighted(10000, 10)
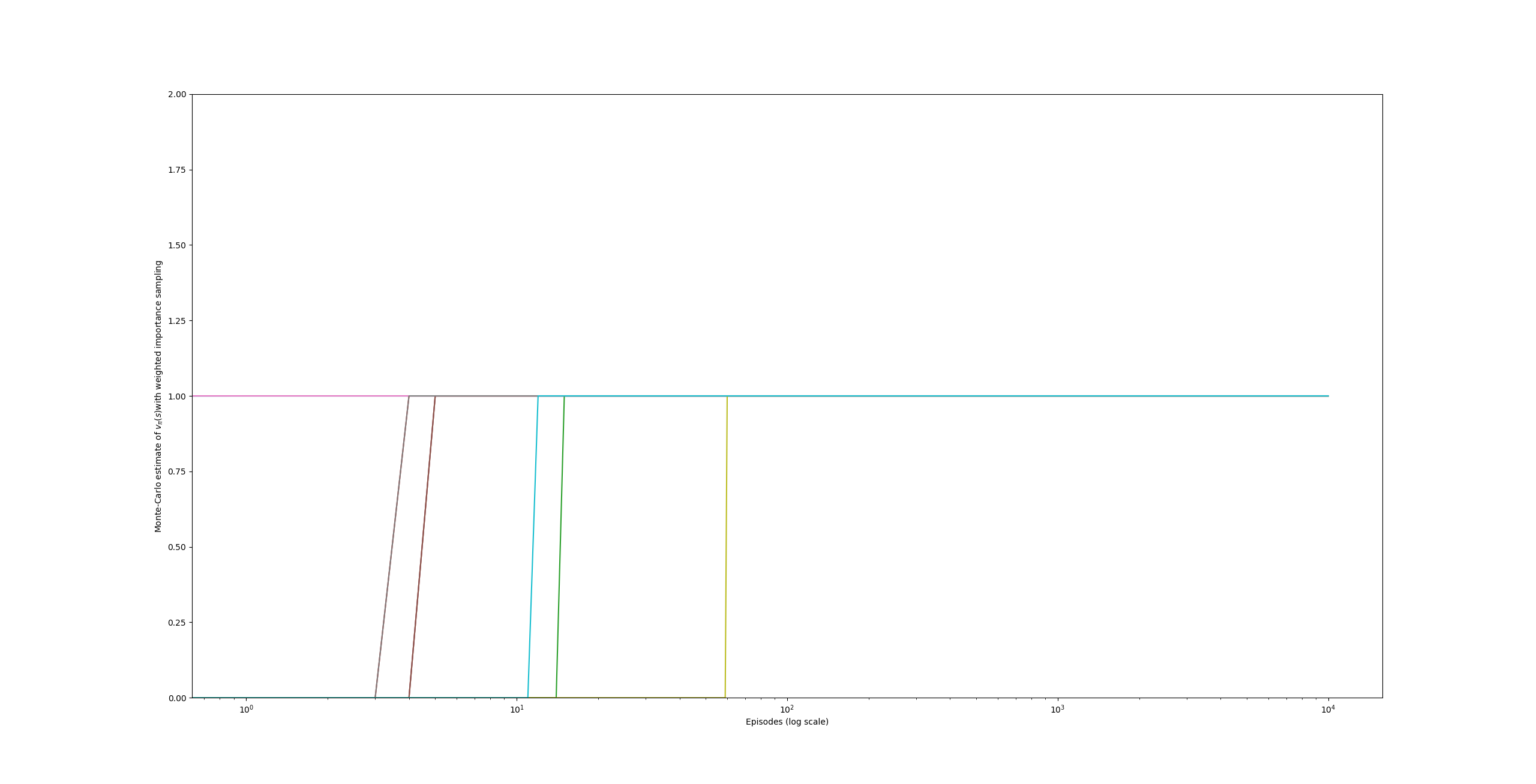