怎么把域名解析到gcp
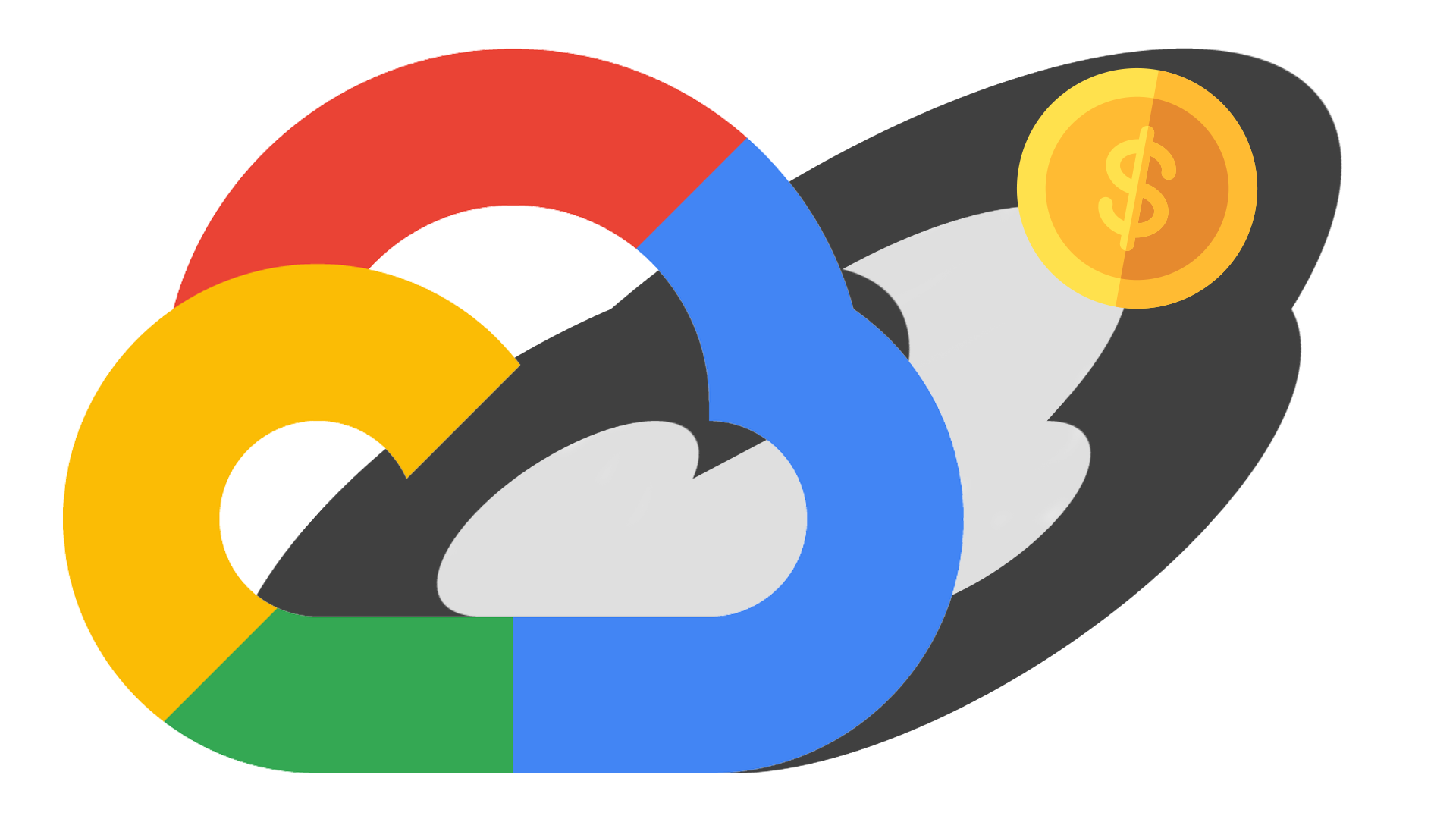
We have all been there — having a nice idea for a hackathon, hobby or a side project and having a burning desire to start coding as soon as possible. And how many possibilities (Heroku, Glitch and others) are there now to bootstrap your app and deploy it immediately.
我们都曾到过那里-对黑客马拉松,业余爱好或附带项目有一个好主意,并有强烈的愿望尽快开始编码。 现在有多少种可能性(Heroku,Glitch和其他)可以引导您的应用并立即进行部署。
Finding the balance between overcomplicated structures and oversimplified solutions with no security is a particularly challenging task sometimes. I think that modern Google Cloud Platform ecosystem provides a nice toolset for solving this problem without vendor lock-in (almost, of course we'll use some specific services but they are easy to migrate from).
在没有安全性的情况下,要在过于复杂的结构和过于简化的解决方案之间找到平衡,这是一项特别具有挑战性的任务。 我认为现代的Google Cloud Platform生态系统提供了一个很好的工具集,无需供应商锁定即可解决此问题(几乎,我们当然会使用一些特定的服务,但它们很容易从中迁移)。
As an example for an app I will use the chatbot application. It's open sourced and hosted in GitHub.
作为一个应用程序的示例,我将使用chatbot应用程序。 它是开源的,并托管在GitHub中 。
快速演示 (Quick demo)
What's better than a live demo? A live demo that is available on demand! Go to Facebook page for the chatbot and drop a few lines to it. Notice how the first response takes some time and after that the responses are smooth and fast. (Of course, if somebody used the app in the past 15 minutes there would be no warm-up time).
有什么比现场演示更好? 现场演示可按需提供! 转到聊天机器人的Facebook页面 ,并在其中添加几行。 请注意,第一个响应是如何花费一些时间的,而在此之后响应是平稳且快速的。 (当然,如果有人在过去15分钟内使用了该应用程序,则没有预热时间)。
What just happened here? The Facebook Messenger Platform got your message to the app page and hit the webhook for the chatbot backend. The Cloud Run service which is responsible for backend in our app spun up a new instance of the Docker container with our app and processed the request.
刚刚发生什么事了? Facebook Messenger平台将您的消息发送到应用程序页面,并点击了聊天机器人后端的Webhook。 负责我们应用程序后端的Cloud Run服务使用我们的应用程序启动了Docker容器的新实例并处理了请求。
架构概述 (Architecture overview)
Let's take a look at the overall app architecture in the GCP and then go through the deployment pipeline.
让我们看一下GCP中的整体应用程序架构,然后遍历部署管道。
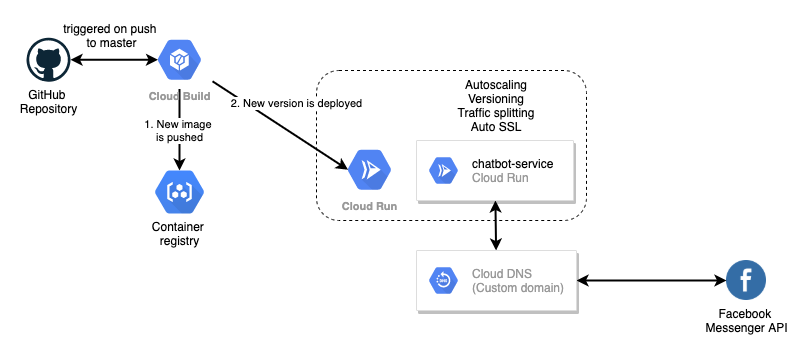
The bot itself is using the Python framework Flask for handling requests. For storage the NoSQL Firestore is used as it's a good fit for small number of concurrent requests and there are no complicated relational queries.
机器人本身正在使用Python框架Flask处理请求。 对于存储,使用NoSQL Firestore是因为它非常适合少量并发请求,并且没有复杂的关系查询。
基础设施 (Infrastructure)
The code is packaged within the Docker image which is built by the Cloud Build service and pushed to the GCP Container Registry. The Cloud Run service is basically the light version for the Kubernetes. It is managing the service exposure to the world, autoscaling, versioning and SSL keys rotation. Each deployed service deployed to the Cloud Run gets a service domain name with HTTPS enabled but you can also use the custom domain name and have SSL encryption enabled as well.
该代码打包在由Cloud Build服务构建的Docker映像中,并推送到GCP容器注册表。 Cloud Run服务基本上是Kubernetes的简易版。 它正在管理服务的公开性,自动扩展,版本控制和SSL密钥轮换。 部署到Cloud Run的每个已部署服务都具有启用HTTPS的服务域名,但是您也可以使用自定义域名并启用SSL加密。
The Cloud Run service is responsible for handling incoming requests. The app inside the Docker container can be anything you need (in terms of languages, libraries or other internal dependencies). The only thing you must remember is that apps inside Cloud Run should be stateless. For attaching volumes you would need to use the usual Kubernetes or other services. Storing data in this case is conveniently done with managed SQL or NoSQL services.
Cloud Run服务负责处理传入的请求。 Docker容器内的应用程序可以满足您的任何需求(就语言,库或其他内部依赖项而言)。 您唯一必须记住的是,Cloud Run中的应用程序应该是无状态的。 要附加卷,您将需要使用常规的Kubernetes或其他服务。 在这种情况下,存储数据可以通过托管SQL或NoSQL服务方便地完成。
部署管道 (Deployment pipeline)
The deployment is triggered automatically with push to the master branch. After that Cloud Build picks up the source code, builds a new image and stores it in the GCR. Later Cloud Build creates a new revision of the service in Cloud Run and switches traffic to it. You could also configure a partial switch here for implementing a gradual rollout and detect anomalies before the service goes live for every user.
按下主分支自动触发部署。 之后,Cloud Build会获取源代码,生成一个新映像并将其存储在GCR中。 更高版本的Cloud Build在Cloud Run中创建服务的新修订并将流量切换到该服务。 您还可以在此处配置局部开关,以实现逐步推出并在每个用户启用该服务之前检测异常。
如何使用它? (How to use it?)
We'll go through the process of setting up the similar solution by forking the chatbot app in this article.
我们将通过分叉chatbot应用程序来完成设置类似解决方案的过程。
We'll fork it and clean up so you could have a quick start but if you need a more complex example feel free to return to the original repository for reference.
我们将对其进行分叉并进行清理,以便您可以快速入门,但是如果您需要更复杂的示例,请随时返回原始存储库以供参考。
Fork the repository with the chatbot application as it contains all the necessary files.
将存储库与chatbot应用程序分叉,因为它包含所有必需的文件。
- In the GCP enable the Firestore, Cloud Build and Cloud Run APIs. 在GCP中,启用Firestore,Cloud Build和Cloud Run API。
Create a new project in GCP and go to https://console.cloud.google.com/cloud-build
在GCP中创建一个新项目,然后转到https://console.cloud.google.com/cloud-build
- Connect a forked repository. 连接分支的存储库。
Create a new trigger for this repository and specify the following Substitution variables:
为此存储库创建一个新的触发器,并指定以下Substitution变量:
_SERVICE_NAME (for the name of the service in Cloud Run)
_SERVICE_NAME (用于Cloud Run中服务的名称)
_REGION (region to deploy the service)
_REGION (部署服务的区域)
_IMAGE_NAME (image name to store in GCR)
_IMAGE_NAME (要存储在GCR中的图片名称)
- Clone the forked repository 克隆分叉的存储库
Go inside the repository folder and delete the files that are specific for the chatbot:
进入存储库文件夹并删除特定于聊天机器人的文件:
rm config.py fb.py gcp.py logging_handler.py skills.json
Replace the content of the app.py file with the following snippet:
使用以下代码段替换app.py文件的内容:
import os from flask import request from flask import Flask app = Flask(__name__) @app.route("/", methods=["GET"]) def hello_there(): return "Hello Cloud Run", 200 if __name__ == "__main__": app.run(debug=True, host="0.0.0.0", port=int(os.environ.get("PORT", 8080)))
We prepared our repository for continuous delivery of our application. Now when you'll commit the changes and push them to a forked repository the Cloud Build service will build and deploy a new application.
我们准备了存储库以连续交付应用程序。 现在,当您提交更改并将其推送到分支的存储库时,Cloud Build服务将构建并部署新的应用程序。
- Commit all the changes and push it to the remote forked repository. 提交所有更改,并将其推送到远程分支的存储库。
Go to https://console.cloud.google.com/run and retrieve the URL for a service. Check that it's working and we receive the "Hello Cloud Run" message.
转到https://console.cloud.google.com/run并检索服务的URL。 检查它是否正常运行,我们会收到“ Hello Cloud Run”消息。
Go to https://console.cloud.google.com/cloud-build to see the information about the build or any problems that occured.
转到https://console.cloud.google.com/cloud-build,以查看有关构建或发生的任何问题的信息。
Now let's take a look at the most interesting files here:
现在,让我们看一下这里最有趣的文件:
cloudbuild.yaml contains all the build and deployment steps. We specify the docker images used for each build step and provide arguments to it. The last step is creating a new revision with a fresh Docker image and finishing up the deployment.
cloudbuild.yaml包含所有构建和部署步骤。 我们指定用于每个构建步骤的docker映像并为其提供参数。 最后一步是使用新的Docker映像创建新修订并完成部署。
Dockerfile contains the information about how container is executed. In this example we are using gunicorn webserver and serving our Flask app.
Dockerfile包含有关如何执行容器的信息。 在此示例中,我们使用gunicorn网络服务器并提供了Flask应用。
帐单和费用 (Billing and costs)
Each service in this demo except for the Secrets Manager has a free tier. Cloud Run is not running 24/7 but only spins up a container instance when there is a need. This enables us to save a lot of resources, gives us more control over the application (it's not a serverless function or similar solution) and grants the autoscaling capabilities.
此演示中的每个服务(“秘密管理器”除外)都具有免费层。 Cloud Run不是全天候运行,而是仅在需要时启动容器实例。 这使我们能够节省大量资源,为我们提供了对应用程序的更多控制权(这不是无服务器功能或类似的解决方案),并授予了自动缩放功能。
The total cost for running this project with a low load (it's a simple chatbot that is not interacting with user all the time) is the cost of 2 secrets in the Secrets Manager service which is 0.12 $ per month. Everything else is covered by the free tier. Of course this applies only if the app is not having a surge in users but even in this case it could automatically scale up and down without intervention from our side.
以低负载运行此项目的总成本(这是一个始终不与用户交互的简单聊天机器人),是Secrets Manager服务中2个机密的成本,每月0.12美元。 免费套餐涵盖了其他所有内容。 当然,这仅在应用程序的用户数量没有增加的情况下适用,即使在这种情况下,它也可以自动缩放,而无需我们的干预。
何必呢? (Why bother?)
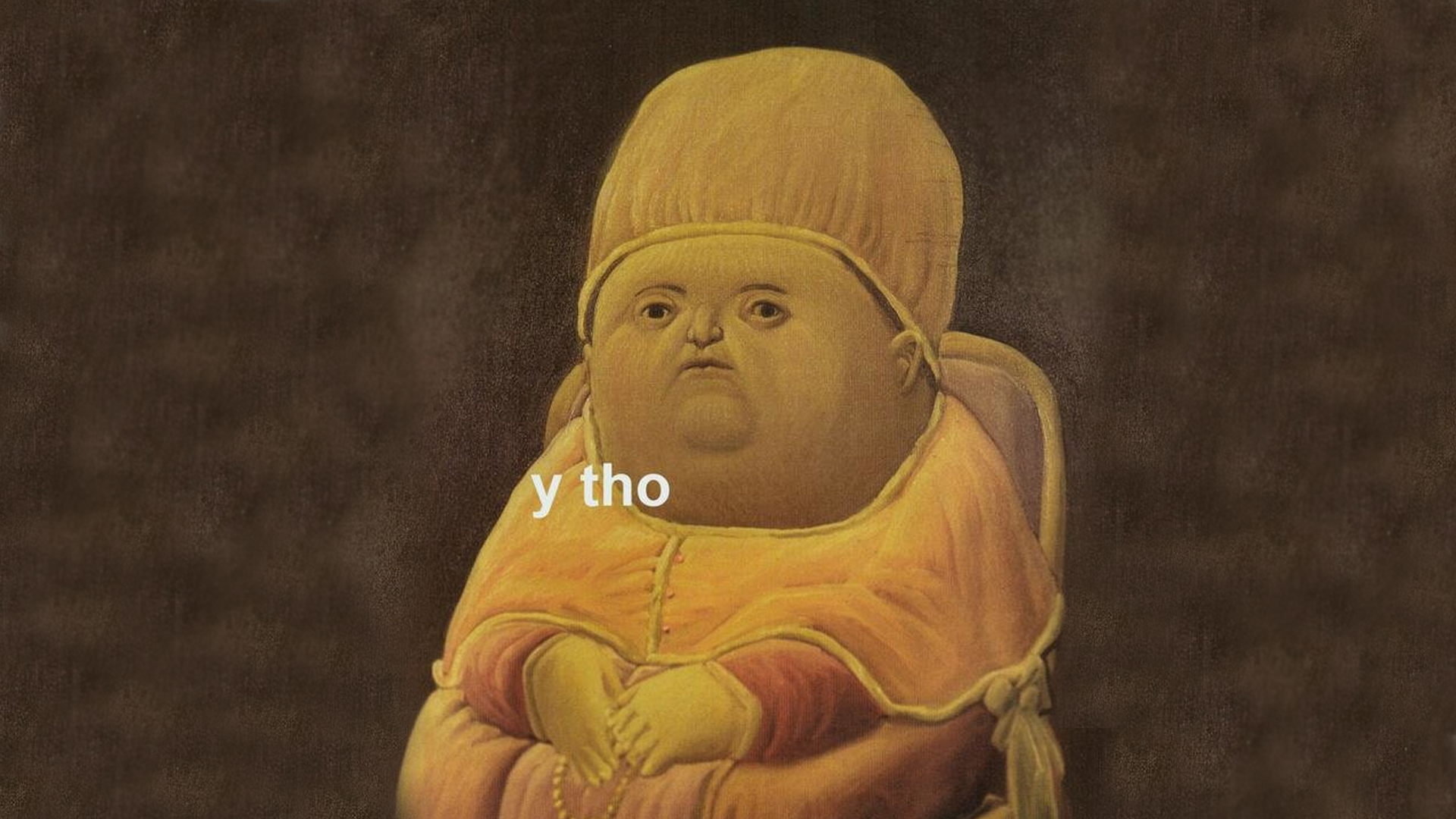
Building small applications for fun, side project or as a hackathon entry is always a tricky task. We could have no setup at all and the app would never even had a change to communicate with users. We could have an extremely expensive setup living on a trial money from GCP or other provider which would be shutdown as soon as there are no more credits. We could also deploy it as a serverless app or use a PaaS provider but it would mean we lose a major control over the app and if we would like to migrate in the future it would require some time to do this.
为娱乐,附带项目或作为黑客马拉松项目而构建小型应用程序始终是一项棘手的任务。 我们可能根本没有任何设置,而且该应用程序甚至也不会进行任何更改以与用户通信。 我们可能有一个非常昂贵的设置,要靠GCP或其他提供商提供的试用费来维持,一旦没有更多的信用额度就会立即关闭。 我们也可以将其部署为无服务器应用程序或使用PaaS提供程序,但这意味着我们对该应用程序失去了主要控制权,如果我们将来希望进行迁移,则需要一些时间来完成。
The system architecture in this article is an attempt to make small projects available for a longer periods of time while keeping them a little more secure and ready for the potential growth.
本文中的系统体系结构是为了使小型项目可以使用更长的时间,同时又使它们更安全并为潜在的增长做好准备。
怎么把域名解析到gcp