TensorFlow-梯度下降优化 (TensorFlow - Gradient Descent Optimization)
Gradient descent optimization is considered to be an important concept in data science.
梯度下降优化被认为是数据科学中的重要概念。
Consider the steps shown below to understand the implementation of gradient descent optimization −
考虑以下所示的步骤,以了解梯度下降优化的实现-
第1步 (Step 1)
Include necessary modules and declaration of x and y variables through which we are going to define the gradient descent optimization.
包括必要的模块以及x和y变量的声明,我们将通过它们定义梯度下降优化。
import tensorflow as tf
x = tf.Variable(2, name = 'x', dtype = tf.float32)
log_x = tf.log(x)
log_x_squared = tf.square(log_x)
optimizer = tf.train.GradientDescentOptimizer(0.5)
train = optimizer.minimize(log_x_squared)
第2步 (Step 2)
Initialize the necessary variables and call the optimizers for defining and calling it with respective function.
初始化必要的变量,并调用优化器以使用相应的函数进行定义和调用。
init = tf.initialize_all_variables()
def optimize():
with tf.Session() as session:
session.run(init)
print("starting at", "x:", session.run(x), "log(x)^2:", session.run(log_x_squared))
for step in range(10):
session.run(train)
print("step", step, "x:", session.run(x), "log(x)^2:", session.run(log_x_squared))
optimize()
The above line of code generates an output as shown in the screenshot below −
上面的代码行生成输出,如下面的屏幕快照所示-
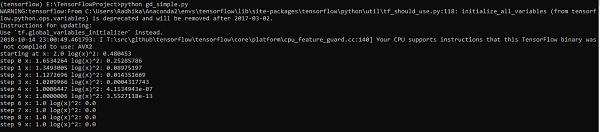
We can see that the necessary epochs and iterations are calculated as shown in the output.
我们可以看到,如输出所示,计算了必要的纪元和迭代。
翻译自: https://www.tutorialspoint.com/tensorflow/tensorflow_gradient_descent_optimization.htm